PVC-InternVL2-8B
[π Paper] [π GitHub] [π Quick Start]
Introduction
We introduce the Progressive Visual Token Compression (PVC) in large vision-language models (VLMs), which unifies the visual inputs as videos and progressively compresses vision tokens across video frames. Our PVC achieves:
- Preserve spatial details and temporal dynamics for both images and videos.
- Effectively reduce the tokens used for each video frame and image tile.
- SoTA performance on various video benchmarks, including long and fine-grained short video tasks.
- No performance loss on image benchmarks, especially on detail-sensitive tasks.
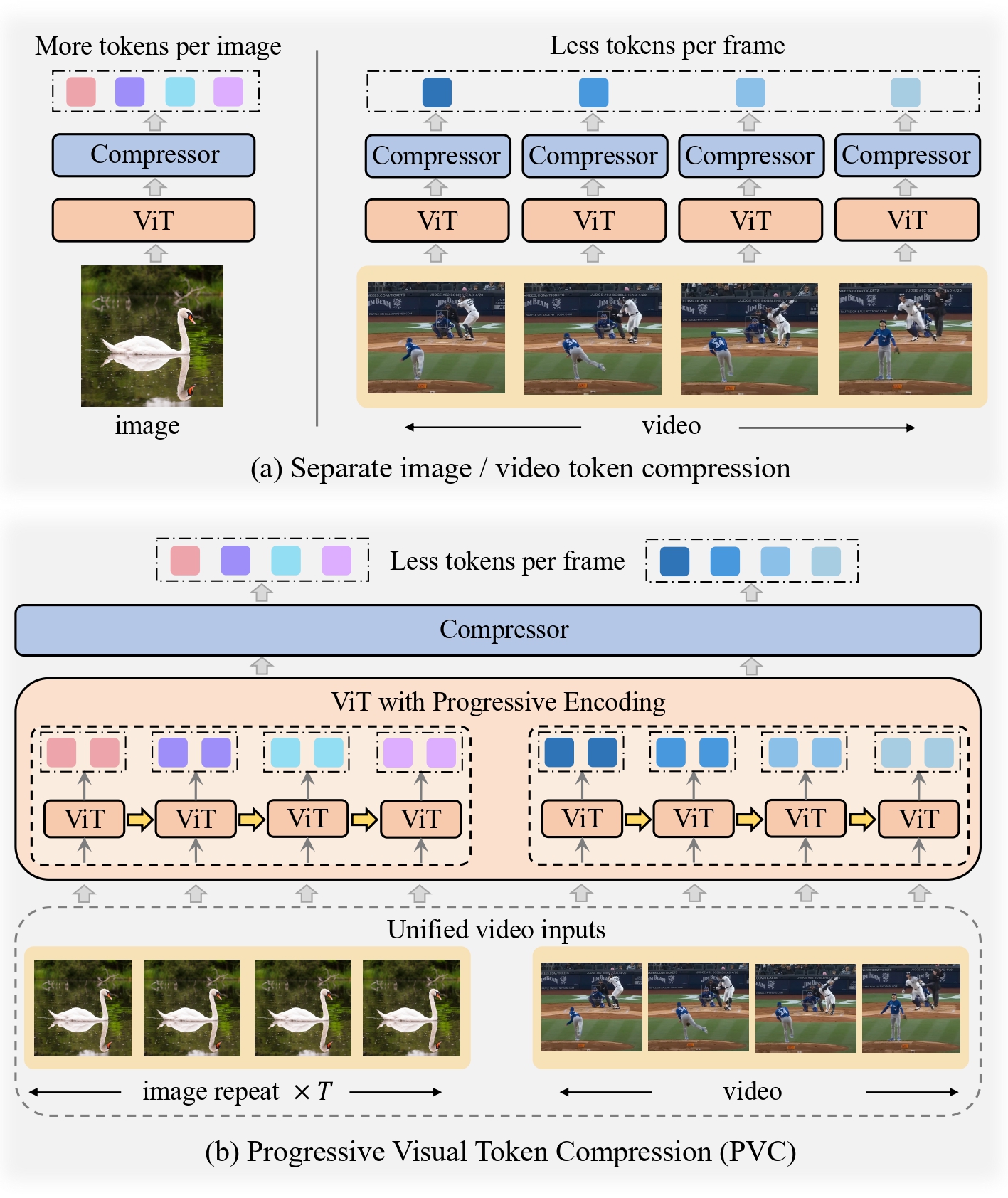
Results
Our implementation is based on the InternVL2 model, referred to as PVCInternVL2
Video Understanding Benckmarks
Model | LLaVA-OneVision-7B | Qwen2-VL-7B | InternVL2-8B | PVCInternVL2-8B |
---|---|---|---|---|
# token/frame | 196 | - | 256 | 64 |
MVbench | 56.7 | 67.0 | 66.4 | 73.8 |
VideoMME w/o-sub | 58.2 | 63.3 | 54.0 | 64.1 |
VideoMME w-sub | 61.5 | 69.0 | 56.9 | 69.7 |
MLVU | 64.7 | - | 52.0 | 72.4 |
LongVideoBench | 56.5 | - | - | 59.2 |
NextQA | 79.4 | - | - | 82.0 |
Egoschema | 60.1 | 66.7 | 55.0 | 59.6 |
PercepTest | 57.1 | 62.3 | 52.0 | 68.4 |
AcNet-QA | 56.6 | - | - | 57.1 |
Image Understanding Benckmarks
Model | LLaVA-OneVision-7B | Qwen2-VL-7B | InternVL2-8B | PVCInternVL2-8B |
---|---|---|---|---|
# token/image tile | 729 | - | 256 | 64 |
AI2Dtest | 81.4 | 83.0 | 83.8 | 83.8 |
ChartQAtest | 80.0 | 83.0 | 83.3 | 84.1 |
DocVQAtest | 87.5 | 94.5 | 91.6 | 92.5 |
InfoVQAtest | 68.8 | 76.5 | 74.8 | 75.0 |
SQAtest | 96.0 | - | 97.1 | 97.7 |
TextVQAval | - | 84.3 | 77.4 | 80.0 |
MMBen-test | - | 83.0 | 81.7 | 83.9 |
MMEsum | 1998 | 2327 | 2210 | 2282 |
MMMUval | 48.8 | 54.1 | 49.3 | 50.9 |
SEEDI | 75.4 | - | 76.2 | 77.2 |
OCRBench | - | 866 | 794 | 807 |
Quick Start
import numpy as np
import torch
import torchvision.transforms as T
from decord import VideoReader, cpu
from PIL import Image
from torchvision.transforms.functional import InterpolationMode
from transformers import AutoModel, AutoTokenizer
IMAGENET_MEAN = (0.485, 0.456, 0.406)
IMAGENET_STD = (0.229, 0.224, 0.225)
def build_transform(input_size):
MEAN, STD = IMAGENET_MEAN, IMAGENET_STD
transform = T.Compose([
T.Lambda(lambda img: img.convert('RGB') if img.mode != 'RGB' else img),
T.Resize((input_size, input_size), interpolation=InterpolationMode.BICUBIC),
T.ToTensor(),
T.Normalize(mean=MEAN, std=STD)
])
return transform
def find_closest_aspect_ratio(aspect_ratio, target_ratios, width, height, image_size):
best_ratio_diff = float('inf')
best_ratio = (1, 1)
area = width * height
for ratio in target_ratios:
target_aspect_ratio = ratio[0] / ratio[1]
ratio_diff = abs(aspect_ratio - target_aspect_ratio)
if ratio_diff < best_ratio_diff:
best_ratio_diff = ratio_diff
best_ratio = ratio
elif ratio_diff == best_ratio_diff:
if area > 0.5 * image_size * image_size * ratio[0] * ratio[1]:
best_ratio = ratio
return best_ratio
def dynamic_preprocess(image, min_num=1, max_num=12, image_size=448, use_thumbnail=False):
orig_width, orig_height = image.size
aspect_ratio = orig_width / orig_height
# calculate the existing image aspect ratio
target_ratios = set(
(i, j) for n in range(min_num, max_num + 1) for i in range(1, n + 1) for j in range(1, n + 1) if
i * j <= max_num and i * j >= min_num)
target_ratios = sorted(target_ratios, key=lambda x: x[0] * x[1])
# find the closest aspect ratio to the target
target_aspect_ratio = find_closest_aspect_ratio(
aspect_ratio, target_ratios, orig_width, orig_height, image_size)
# calculate the target width and height
target_width = image_size * target_aspect_ratio[0]
target_height = image_size * target_aspect_ratio[1]
blocks = target_aspect_ratio[0] * target_aspect_ratio[1]
# resize the image
resized_img = image.resize((target_width, target_height))
processed_images = []
for i in range(blocks):
box = (
(i % (target_width // image_size)) * image_size,
(i // (target_width // image_size)) * image_size,
((i % (target_width // image_size)) + 1) * image_size,
((i // (target_width // image_size)) + 1) * image_size
)
# split the image
split_img = resized_img.crop(box)
processed_images.append(split_img)
assert len(processed_images) == blocks
if use_thumbnail and len(processed_images) != 1:
thumbnail_img = image.resize((image_size, image_size))
processed_images.append(thumbnail_img)
return processed_images
def load_image(image_file, input_size=448, max_num=12):
image = Image.open(image_file).convert('RGB')
transform = build_transform(input_size=input_size)
images = dynamic_preprocess(image, image_size=input_size, use_thumbnail=True, max_num=max_num)
pixel_values = [transform(image) for image in images]
pixel_values = torch.stack(pixel_values)
return pixel_values
def get_index(bound, fps, max_frame, first_idx=0, num_segments=32):
if bound:
start, end = bound[0], bound[1]
else:
start, end = -100000, 100000
start_idx = max(first_idx, round(start * fps))
end_idx = min(round(end * fps), max_frame)
seg_size = float(end_idx - start_idx) / num_segments
frame_indices = np.array([
int(start_idx + (seg_size / 2) + np.round(seg_size * idx))
for idx in range(num_segments)
])
return frame_indices
def load_video(video_path, bound=None, input_size=448, max_num=1, num_segments=32):
vr = VideoReader(video_path, ctx=cpu(0), num_threads=1)
max_frame = len(vr) - 1
fps = float(vr.get_avg_fps())
pixel_values_list, num_patches_list = [], []
transform = build_transform(input_size=input_size)
frame_indices = get_index(bound, fps, max_frame, first_idx=0, num_segments=num_segments)
for frame_index in frame_indices:
img = Image.fromarray(vr[frame_index].asnumpy()).convert('RGB')
img = dynamic_preprocess(img, image_size=input_size, use_thumbnail=True, max_num=max_num)
pixel_values = [transform(tile) for tile in img]
pixel_values = torch.stack(pixel_values)
num_patches_list.append(pixel_values.shape[0])
pixel_values_list.append(pixel_values)
pixel_values = torch.cat(pixel_values_list)
return pixel_values, num_patches_list
path = 'OpenGVLab/PVC-InternVL2-8B'
model = AutoModel.from_pretrained(
path,
torch_dtype=torch.bfloat16,
low_cpu_mem_usage=True,
trust_remote_code=True).eval().cuda()
tokenizer = AutoTokenizer.from_pretrained(path, trust_remote_code=True, use_fast=False)
generation_config = dict(max_new_tokens=1024, do_sample=True)
# single-image conversation
pixel_values = load_image('./assets/example_image1.jpg', max_num=12).to(torch.bfloat16).cuda()
data_flag = torch.tensor([1], dtype=torch.long).cuda()
question = '<image>\nWhat is in the image?'
response = model.chat(tokenizer, pixel_values, question, generation_config, data_flag=data_flag)
print(f'User: {question}\nAssistant: {response}')
# multi-image conversation
pixel_values1 = load_image('./assets/example_image1.jpg', max_num=12).to(torch.bfloat16).cuda()
pixel_values2 = load_image('./assets/example_image2.jpg', max_num=12).to(torch.bfloat16).cuda()
pixel_values = torch.cat((pixel_values1, pixel_values2), dim=0)
data_flag = torch.tensor([2], dtype=torch.long).cuda()
num_patches_list = [pixel_values1.shape[0], pixel_values2.shape[0]]
question = 'Image-1: <image>\nImage-2: <image>\nWhat are the similarities and differences between these two images.'
response = model.chat(tokenizer, pixel_values, question, generation_config, data_flag=data_flag, num_patches_list=num_patches_list)
print(f'User: {question}\nAssistant: {response}')
# video conversation
pixel_values, num_patches_list = load_video('./assets/example_video.mp4', num_segments=64, max_num=1)
pixel_values = pixel_values.to(torch.bfloat16).cuda()
video_prefix = ''.join([f'Frame{i+1}: <image>\n' for i in range(len(num_patches_list))])
# Frame1: <image>\nFrame2: <image>\n...\nFrameN: <image>\n{question}
data_flag = torch.tensor([3], dtype=torch.long).cuda()
question = video_prefix + 'Describe this video in detail.'
response = model.chat(tokenizer, pixel_values, question, generation_config, data_flag=data_flag, num_patches_list=num_patches_list)
print(f'User: {question}\nAssistant: {response}')
Evaluation
Please refer to our Github Repo.
Citation
If you find this work helpful in your research, please consider citing:
@article{yang2024pvc,
title={PVC: Progressive Visual Token Compression for Unified Image and Video Processing in Large Vision-Language Models},
author={Yang, Chenyu and Dong, Xuan and Zhu, Xizhou and Su, Weijie and Wang, Jiahao and Tian, Hao and Chen, Zhe and Wang, Wenhai and Lu, Lewei and and Dai, Jifeng},
journal={arXiv preprint arXiv:2412.09613},
year={2024}
}
License
This project is released under the MIT license. Parts of this project contain code and models from other sources, which are subject to their respective licenses.
- Downloads last month
- 19
Inference Providers
NEW
This model isn't deployed by any Inference Provider.
π
Ask for provider support