Thanks for sharing
Andreas Chandra
andreaschandra
AI & ML interests
Sentiment Analysis, Question Answering, Text Summarization, Language Model
Recent Activity
replied to
juhoinkinen's
post
1 day ago
We (@osma, @MonaLehtinen & me, i.e. the Annif team at the National Library of Finland) recently took part in the LLMs4Subjects challenge at the SemEval-2025 workshop. The task was to use large language models (LLMs) to generate good quality subject indexing for bibliographic records, i.e. titles and abstracts.
We are glad to report that our system performed well; it was ranked
π₯ 1st in the category where the full vocabulary was used
π₯ 2nd in the smaller vocabulary category
π
4th in the qualitative evaluations.
14 participating teams developed their own solutions for generating subject headings and the output of each system was assessed using both quantitative and qualitative evaluations. Research papers about most of the systems are going to be published around the time of the workshop in late July, and many pre-prints are already available.
We applied Annif together with several LLMs that we used to preprocess the data sets: translated the GND vocabulary terms to English, translated bibliographic records into English and German as required, and generated additional synthetic training data. After the preprocessing, we used the traditional machine learning algorithms in Annif as well as the experimental XTransformer algorithm that is based on language models. We also combined the subject suggestions generated using English and German language records in a novel way.
More information can be found in our system description preprint: https://huggingface.co/papers/2504.19675
See also the task description preprint: https://huggingface.co/papers/2504.07199
The Annif models trained for this task are available here: https://huggingface.co/NatLibFi/Annif-LLMs4Subjects-data
reacted
to
juhoinkinen's
post
with π
1 day ago
We (@osma, @MonaLehtinen & me, i.e. the Annif team at the National Library of Finland) recently took part in the LLMs4Subjects challenge at the SemEval-2025 workshop. The task was to use large language models (LLMs) to generate good quality subject indexing for bibliographic records, i.e. titles and abstracts.
We are glad to report that our system performed well; it was ranked
π₯ 1st in the category where the full vocabulary was used
π₯ 2nd in the smaller vocabulary category
π
4th in the qualitative evaluations.
14 participating teams developed their own solutions for generating subject headings and the output of each system was assessed using both quantitative and qualitative evaluations. Research papers about most of the systems are going to be published around the time of the workshop in late July, and many pre-prints are already available.
We applied Annif together with several LLMs that we used to preprocess the data sets: translated the GND vocabulary terms to English, translated bibliographic records into English and German as required, and generated additional synthetic training data. After the preprocessing, we used the traditional machine learning algorithms in Annif as well as the experimental XTransformer algorithm that is based on language models. We also combined the subject suggestions generated using English and German language records in a novel way.
More information can be found in our system description preprint: https://huggingface.co/papers/2504.19675
See also the task description preprint: https://huggingface.co/papers/2504.07199
The Annif models trained for this task are available here: https://huggingface.co/NatLibFi/Annif-LLMs4Subjects-data
Organizations
andreaschandra's activity
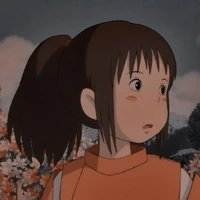
replied to
juhoinkinen's
post
1 day ago
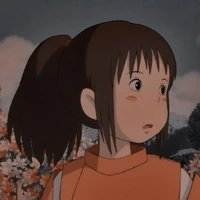
reacted to
juhoinkinen's
post with π
1 day ago
Post
2399
We (
@osma
,
@MonaLehtinen
& me, i.e. the Annif team at the National Library of Finland) recently took part in the LLMs4Subjects challenge at the SemEval-2025 workshop. The task was to use large language models (LLMs) to generate good quality subject indexing for bibliographic records, i.e. titles and abstracts.
We are glad to report that our system performed well; it was ranked
π₯ 1st in the category where the full vocabulary was used
π₯ 2nd in the smaller vocabulary category
π 4th in the qualitative evaluations.
14 participating teams developed their own solutions for generating subject headings and the output of each system was assessed using both quantitative and qualitative evaluations. Research papers about most of the systems are going to be published around the time of the workshop in late July, and many pre-prints are already available.
We applied Annif together with several LLMs that we used to preprocess the data sets: translated the GND vocabulary terms to English, translated bibliographic records into English and German as required, and generated additional synthetic training data. After the preprocessing, we used the traditional machine learning algorithms in Annif as well as the experimental XTransformer algorithm that is based on language models. We also combined the subject suggestions generated using English and German language records in a novel way.
More information can be found in our system description preprint: Annif at SemEval-2025 Task 5: Traditional XMTC augmented by LLMs (2504.19675)
See also the task description preprint: SemEval-2025 Task 5: LLMs4Subjects -- LLM-based Automated Subject Tagging for a National Technical Library's Open-Access Catalog (2504.07199)
The Annif models trained for this task are available here: NatLibFi/Annif-LLMs4Subjects-data
We are glad to report that our system performed well; it was ranked
π₯ 1st in the category where the full vocabulary was used
π₯ 2nd in the smaller vocabulary category
π 4th in the qualitative evaluations.
14 participating teams developed their own solutions for generating subject headings and the output of each system was assessed using both quantitative and qualitative evaluations. Research papers about most of the systems are going to be published around the time of the workshop in late July, and many pre-prints are already available.
We applied Annif together with several LLMs that we used to preprocess the data sets: translated the GND vocabulary terms to English, translated bibliographic records into English and German as required, and generated additional synthetic training data. After the preprocessing, we used the traditional machine learning algorithms in Annif as well as the experimental XTransformer algorithm that is based on language models. We also combined the subject suggestions generated using English and German language records in a novel way.
More information can be found in our system description preprint: Annif at SemEval-2025 Task 5: Traditional XMTC augmented by LLMs (2504.19675)
See also the task description preprint: SemEval-2025 Task 5: LLMs4Subjects -- LLM-based Automated Subject Tagging for a National Technical Library's Open-Access Catalog (2504.07199)
The Annif models trained for this task are available here: NatLibFi/Annif-LLMs4Subjects-data
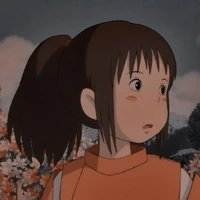
reacted to
DawnC's
post with π
1 day ago
Post
4611
VisionScout β Now with Video Analysis! π
Iβm excited to announce a major update to VisionScout, my interactive vision tool that now supports VIDEO PROCESSING, in addition to powerful object detection and scene understanding!
βοΈ NEW: Video Analysis Is Here!
π¬ Upload any video file to detect and track objects using YOLOv8.
β±οΈ Customize processing intervals to balance speed and thoroughness.
π Get comprehensive statistics and summaries showing object appearances across the entire video.
What else can VisionScout do?
πΌοΈ Analyze any image and detect 80 object types with YOLOv8.
π Switch between Nano, Medium, and XLarge models for speed or accuracy.
π― Filter by object classes (people, vehicles, animals, etc.) to focus on what matters.
π View detailed stats on detections, confidence levels, and distributions.
π§ Understand scenes β interpreting environments and potential activities.
β οΈ Automatically identify possible safety concerns based on detected objects.
Whatβs coming next?
π Expanding YOLOβs object categories.
β‘ Faster real-time performance.
π± Improved mobile responsiveness.
My goal:
To bridge the gap between raw detection and meaningful interpretation.
Iβm constantly exploring ways to help machines not just "see" but truly understand context β and to make these advanced tools accessible to everyone, regardless of technical background.
Try it now! πΌοΈπ DawnC/VisionScout
If you enjoy VisionScout, a β€οΈ Like for this project or feedback would mean a lot and keeps me motivated to keep building and improving!
#ComputerVision #ObjectDetection #VideoAnalysis #YOLO #SceneUnderstanding #MachineLearning #TechForLife
Iβm excited to announce a major update to VisionScout, my interactive vision tool that now supports VIDEO PROCESSING, in addition to powerful object detection and scene understanding!
βοΈ NEW: Video Analysis Is Here!
π¬ Upload any video file to detect and track objects using YOLOv8.
β±οΈ Customize processing intervals to balance speed and thoroughness.
π Get comprehensive statistics and summaries showing object appearances across the entire video.
What else can VisionScout do?
πΌοΈ Analyze any image and detect 80 object types with YOLOv8.
π Switch between Nano, Medium, and XLarge models for speed or accuracy.
π― Filter by object classes (people, vehicles, animals, etc.) to focus on what matters.
π View detailed stats on detections, confidence levels, and distributions.
π§ Understand scenes β interpreting environments and potential activities.
β οΈ Automatically identify possible safety concerns based on detected objects.
Whatβs coming next?
π Expanding YOLOβs object categories.
β‘ Faster real-time performance.
π± Improved mobile responsiveness.
My goal:
To bridge the gap between raw detection and meaningful interpretation.
Iβm constantly exploring ways to help machines not just "see" but truly understand context β and to make these advanced tools accessible to everyone, regardless of technical background.
Try it now! πΌοΈπ DawnC/VisionScout
If you enjoy VisionScout, a β€οΈ Like for this project or feedback would mean a lot and keeps me motivated to keep building and improving!
#ComputerVision #ObjectDetection #VideoAnalysis #YOLO #SceneUnderstanding #MachineLearning #TechForLife
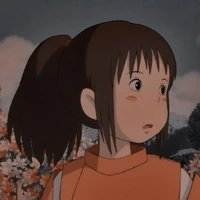
reacted to
onekq's
post with π€
1 day ago
Post
2267
This time Gemini is very quick with API support on its 2.5 pro May release. The performance is impressive too, now it is among top contenders like o4, R1, and Claude.
onekq-ai/WebApp1K-models-leaderboard
onekq-ai/WebApp1K-models-leaderboard
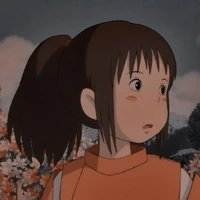
reacted to
cfahlgren1's
post with ππ₯
4 months ago
Post
1770
Wow, I just added Langfuse tracing to the Deepseek Artifacts app and it's really nice π₯
It allows me to visualize and track more things along with the cfahlgren1/react-code-instructions dataset.
It was just added as a one click Docker Space template, so it's super easy to self host πͺ
It allows me to visualize and track more things along with the cfahlgren1/react-code-instructions dataset.
It was just added as a one click Docker Space template, so it's super easy to self host πͺ