Dataset Viewer
_id
stringlengths 24
24
| id
stringlengths 5
121
| author
stringlengths 2
42
| cardData
stringlengths 2
1.07M
⌀ | disabled
bool 2
classes | gated
null | lastModified
timestamp[ns]date 2021-02-05 16:03:35
2025-04-23 23:31:25
| likes
int64 0
7.72k
| trendingScore
float64 -1
103
| private
bool 1
class | sha
stringlengths 40
40
| description
stringlengths 0
6.67k
⌀ | downloads
int64 0
5.51M
| downloadsAllTime
int64 0
142M
| tags
sequencelengths 1
7.92k
| createdAt
timestamp[ns]date 2022-03-02 23:29:22
2025-04-23 23:30:55
| paperswithcode_id
stringclasses 658
values | citation
stringlengths 0
10.7k
⌀ |
---|---|---|---|---|---|---|---|---|---|---|---|---|---|---|---|---|---|
67fce65dd1ec7d15ba6a2da3 | zwhe99/DeepMath-103K | zwhe99 | {"license": "mit", "configs": [{"config_name": "default", "data_files": [{"split": "train", "path": "data/train-*"}]}], "dataset_info": {"features": [{"name": "question", "dtype": "string"}, {"name": "final_answer", "dtype": "string"}, {"name": "difficulty", "dtype": "float64"}, {"name": "topic", "dtype": "string"}, {"name": "r1_solution_1", "dtype": "string"}, {"name": "r1_solution_2", "dtype": "string"}, {"name": "r1_solution_3", "dtype": "string"}], "splits": [{"name": "train", "num_bytes": 4963982703, "num_examples": 103110}], "download_size": 2135928958, "dataset_size": 4963982703}, "task_categories": ["text-generation", "text2text-generation"], "language": ["en"], "tags": ["math", "reasoning", "rl"], "pretty_name": "deepmath-103k", "size_categories": ["100K<n<1M"]} | false | null | 2025-04-18T06:29:38 | 138 | 103 | false | 736ce9bfca63afc046a07d545915fa261bbe843f |
DeepMath-103K
📖 Overview
DeepMath-103K is meticulously curated to push the boundaries of mathematical reasoning in language models. Key features include:1. Challenging Problems: DeepMath-103K has a strong focus on difficult mathematical problems (primarily Levels 5-9), significantly raising the complexity bar compared to many existing open datasets.
Difficulty… See the full description on the dataset page: https://huggingface.co/datasets/zwhe99/DeepMath-103K. | 9,798 | 9,798 | [
"task_categories:text-generation",
"task_categories:text2text-generation",
"language:en",
"license:mit",
"size_categories:100K<n<1M",
"format:parquet",
"modality:text",
"library:datasets",
"library:dask",
"library:mlcroissant",
"library:polars",
"arxiv:2504.11456",
"region:us",
"math",
"reasoning",
"rl"
] | 2025-04-14T10:41:33 | null | null |
67f75f7450cb3eb7e88dc887 | Anthropic/values-in-the-wild | Anthropic | {"license": "cc-by-4.0"} | false | null | 2025-04-21T00:39:48 | 86 | 86 | false | 984078fc407bb5c6c3e754c8f571825754842a18 |
Summary
This dataset presents a comprehensive taxonomy of 3307 values expressed by Claude (an AI assistant) across hundreds of thousands of real-world conversations. Using a novel privacy-preserving methodology, these values were extracted and classified without human reviewers accessing any conversation content. The dataset reveals patterns in how AI systems express values "in the wild" when interacting with diverse users and tasks.
We're releasing this resource to advance research… See the full description on the dataset page: https://huggingface.co/datasets/Anthropic/values-in-the-wild. | 185 | 185 | [
"license:cc-by-4.0",
"region:us"
] | 2025-04-10T06:04:36 | null | null |
67ec47948647cfa17739af7a | nvidia/OpenCodeReasoning | nvidia | {"license": "cc-by-4.0", "size_categories": ["100K<n<1M"], "pretty_name": "OpenCodeReasoning", "dataset_info": [{"config_name": "split_0", "features": [{"name": "id", "dtype": "string"}, {"name": "input", "dtype": "string"}, {"name": "output", "dtype": "string"}, {"name": "source", "dtype": "string"}, {"name": "license", "dtype": "string"}, {"name": "dataset", "dtype": "string"}, {"name": "split", "dtype": "string"}, {"name": "difficulty", "dtype": "string"}, {"name": "solution", "dtype": "string"}], "splits": [{"name": "split_0", "num_bytes": 28108469190, "num_examples": 567850}]}, {"config_name": "split_1", "features": [{"name": "id", "dtype": "string"}, {"name": "input", "dtype": "string"}, {"name": "output", "dtype": "string"}, {"name": "source", "dtype": "string"}, {"name": "license", "dtype": "string"}, {"name": "dataset", "dtype": "string"}, {"name": "split", "dtype": "string"}, {"name": "difficulty", "dtype": "string"}, {"name": "solution", "dtype": "string"}, {"name": "index", "dtype": "string"}], "splits": [{"name": "split_1", "num_bytes": 4722811278, "num_examples": 167405}]}], "configs": [{"config_name": "split_0", "data_files": [{"split": "split_0", "path": "split_0/train-*"}]}, {"config_name": "split_1", "data_files": [{"split": "split_1", "path": "split_1/train-*"}]}], "task_categories": ["text-generation"], "tags": ["synthetic"]} | false | null | 2025-04-15T16:56:07 | 285 | 59 | false | c141f0b01e489370f312cd54985b7b02e8dab8da |
OpenCodeReasoning: Advancing Data Distillation for Competitive Coding
Data Overview
OpenCodeReasoning is the largest reasoning-based synthetic dataset to date for coding, comprises 735,255 samples in Python across 28,319 unique competitive programming
questions. OpenCodeReasoning is designed for supervised fine-tuning (SFT).
Technical Report - Discover the methodology and technical details behind OpenCodeReasoning.
Github Repo - Access the complete pipeline used to… See the full description on the dataset page: https://huggingface.co/datasets/nvidia/OpenCodeReasoning. | 11,942 | 11,942 | [
"task_categories:text-generation",
"license:cc-by-4.0",
"size_categories:100K<n<1M",
"format:parquet",
"modality:text",
"library:datasets",
"library:dask",
"library:mlcroissant",
"library:polars",
"arxiv:2504.01943",
"region:us",
"synthetic"
] | 2025-04-01T20:07:48 | null | null |
67fa1588873f5fd677eb1161 | OpenGVLab/InternVL-Data | OpenGVLab | {"language": ["multilingual"], "license": "cc-by-4.0", "task_categories": ["image-to-text", "question-answering"], "size_categories": ["10M<n<100M"]} | false | null | 2025-04-23T18:09:39 | 44 | 44 | false | 890dc76050f25121fcfcf98b800cb49f5cf3b0a6 |
InternVL-Data
[📂 GitHub] [📜 InternVL 1.0] [📜 InternVL 1.5] [📜 InternVL 2.5] [📜 InternVL2.5-MPO] [📜 InternVL3]
[🆕 Blog] [🗨️ Chat Demo] [🤗 HF Demo] [🚀 Quick Start] [📖 Documents]
Introduction
Welcome to the InternVL3 Open Dataset! This dataset is designed to support research and development in the field of multimodal large language models (MLLMs), specifically for tasks involving image, text, and video understanding. The dataset is composed of data… See the full description on the dataset page: https://huggingface.co/datasets/OpenGVLab/InternVL-Data. | 634 | 634 | [
"task_categories:image-to-text",
"task_categories:question-answering",
"language:multilingual",
"license:cc-by-4.0",
"size_categories:10M<n<100M",
"arxiv:2312.14238",
"arxiv:2404.16821",
"arxiv:2412.05271",
"arxiv:2411.10442",
"arxiv:2504.10479",
"region:us"
] | 2025-04-12T07:26:00 | null | null |
67f9abed63243ae752060832 | openai/mrcr | openai | {"license": "mit"} | false | null | 2025-04-14T18:58:12 | 125 | 41 | false | 204b0d4e8d9ca5c0a90bf942fdb2a5969094adc0 |
OpenAI MRCR: Long context multiple needle in a haystack benchmark
OpenAI MRCR (Multi-round co-reference resolution) is a long context dataset for benchmarking an LLM's ability to distinguish between multiple needles hidden in context.
This eval is inspired by the MRCR eval first introduced by Gemini (https://arxiv.org/pdf/2409.12640v2). OpenAI MRCR expands the tasks's difficulty and provides opensource data for reproducing results.
The task is as follows: The model is given a long… See the full description on the dataset page: https://huggingface.co/datasets/openai/mrcr. | 2,733 | 2,733 | [
"license:mit",
"size_categories:1K<n<10K",
"format:parquet",
"modality:tabular",
"modality:text",
"library:datasets",
"library:dask",
"library:mlcroissant",
"library:polars",
"arxiv:2409.12640",
"region:us"
] | 2025-04-11T23:55:25 | null | null |
63990f21cc50af73d29ecfa3 | fka/awesome-chatgpt-prompts | fka | {"license": "cc0-1.0", "tags": ["ChatGPT"], "task_categories": ["question-answering"], "size_categories": ["100K<n<1M"]} | false | null | 2025-01-06T00:02:53 | 7,719 | 30 | false | 68ba7694e23014788dcc8ab5afe613824f45a05c | 🧠 Awesome ChatGPT Prompts [CSV dataset]
This is a Dataset Repository of Awesome ChatGPT Prompts
View All Prompts on GitHub
License
CC-0
| 11,526 | 148,471 | [
"task_categories:question-answering",
"license:cc0-1.0",
"size_categories:n<1K",
"format:csv",
"modality:text",
"library:datasets",
"library:pandas",
"library:mlcroissant",
"library:polars",
"region:us",
"ChatGPT"
] | 2022-12-13T23:47:45 | null | null |
679dee7e52390b33e5970da6 | future-technologies/Universal-Transformers-Dataset | future-technologies | {"task_categories": ["text-classification", "token-classification", "table-question-answering", "question-answering", "zero-shot-classification", "translation", "summarization", "feature-extraction", "text-generation", "text2text-generation", "fill-mask", "sentence-similarity", "text-to-speech", "text-to-audio", "automatic-speech-recognition", "audio-to-audio", "audio-classification", "voice-activity-detection", "depth-estimation", "image-classification", "object-detection", "image-segmentation", "text-to-image", "image-to-text", "image-to-image", "image-to-video", "unconditional-image-generation", "video-classification", "reinforcement-learning", "robotics", "tabular-classification", "tabular-regression", "tabular-to-text", "table-to-text", "multiple-choice", "text-retrieval", "time-series-forecasting", "text-to-video", "visual-question-answering", "zero-shot-image-classification", "graph-ml", "mask-generation", "zero-shot-object-detection", "text-to-3d", "image-to-3d", "image-feature-extraction", "video-text-to-text"], "language": ["ab", "ace", "ady", "af", "alt", "am", "ami", "an", "ang", "anp", "ar", "arc", "ary", "arz", "as", "ast", "atj", "av", "avk", "awa", "ay", "az", "azb", "ba", "ban", "bar", "bbc", "bcl", "be", "bg", "bh", "bi", "bjn", "blk", "bm", "bn", "bo", "bpy", "br", "bs", "bug", "bxr", "ca", "cbk", "cdo", "ce", "ceb", "ch", "chr", "chy", "ckb", "co", "cr", "crh", "cs", "csb", "cu", "cv", "cy", "da", "dag", "de", "dga", "din", "diq", "dsb", "dty", "dv", "dz", "ee", "el", "eml", "en", "eo", "es", "et", "eu", "ext", "fa", "fat", "ff", "fi", "fj", "fo", "fon", "fr", "frp", "frr", "fur", "fy", "ga", "gag", "gan", "gcr", "gd", "gl", "glk", "gn", "gom", "gor", "got", "gpe", "gsw", "gu", "guc", "gur", "guw", "gv", "ha", "hak", "haw", "hbs", "he", "hi", "hif", "hr", "hsb", "ht", "hu", "hy", "hyw", "ia", "id", "ie", "ig", "ik", "ilo", "inh", "io", "is", "it", "iu", "ja", "jam", "jbo", "jv", "ka", "kaa", "kab", "kbd", "kbp", "kcg", "kg", "ki", "kk", "kl", "km", "kn", "ko", "koi", "krc", "ks", "ksh", "ku", "kv", "kw", "ky", "la", "lad", "lb", "lbe", "lez", "lfn", "lg", "li", "lij", "lld", "lmo", "ln", "lo", "lt", "ltg", "lv", "lzh", "mad", "mai", "map", "mdf", "mg", "mhr", "mi", "min", "mk", "ml", "mn", "mni", "mnw", "mr", "mrj", "ms", "mt", "mwl", "my", "myv", "mzn", "nah", "nan", "nap", "nds", "ne", "new", "nia", "nl", "nn", "no", "nov", "nqo", "nrf", "nso", "nv", "ny", "oc", "olo", "om", "or", "os", "pa", "pag", "pam", "pap", "pcd", "pcm", "pdc", "pfl", "pi", "pih", "pl", "pms", "pnb", "pnt", "ps", "pt", "pwn", "qu", "rm", "rmy", "rn", "ro", "ru", "rue", "rup", "rw", "sa", "sah", "sat", "sc", "scn", "sco", "sd", "se", "sg", "sgs", "shi", "shn", "si", "sk", "skr", "sl", "sm", "smn", "sn", "so", "sq", "sr", "srn", "ss", "st", "stq", "su", "sv", "sw", "szl", "szy", "ta", "tay", "tcy", "te", "tet", "tg", "th", "ti", "tk", "tl", "tly", "tn", "to", "tpi", "tr", "trv", "ts", "tt", "tum", "tw", "ty", "tyv", "udm", "ug", "uk", "ur", "uz", "ve", "vec", "vep", "vi", "vls", "vo", "vro", "wa", "war", "wo", "wuu", "xal", "xh", "xmf", "yi", "yo", "yue", "za", "zea", "zgh", "zh", "zu"], "tags": ["tabular", "video", "image", "audio", "text-prompts", "text", "universal", "transformer", "database", "massive-data", "ai", "training", "huggingface", "ai", "artificial-intelligence", "machine-learning", "deep-learning", "transformers", "neural-networks", "text", "image", "audio", "video", "multimodal", "structured-data", "tabular-data", "nlp", "computer-vision", "speech-recognition", "reinforcement-learning", "time-series", "large-language-models", "generative-ai", "huggingface-dataset", "huggingface", "pytorch", "tensorflow", "jax", "pretraining", "finetuning", "self-supervised-learning", "few-shot-learning", "zero-shot-learning", "unsupervised-learning", "meta-learning", "diffusion-models"], "size_categories": ["n>1T"], "pretty_name": "Universal Transformers: Multilingual & Scalable AI Dataset"} | false | null | 2025-04-15T13:24:42 | 73 | 29 | false | 70d940db37e4cb645437f892fab8a7e5404bb7bf |
Universal Transformer Dataset
💠 A Message from Ujjawal Tyagi (Founder & CEO)
"This is more than a dataset..... it’s the start of a new world....."
I’m Ujjawal Tyagi, Founder of Lambda Go & GoX AI Platform — proudly born in the land of wisdom, resilience, and rising technology..... India 🇮🇳
What we’ve built here isn’t just numbers, files, or data points..... it’s purpose. It’s a movement. It’s for every developer, researcher, and dreamer who wants to… See the full description on the dataset page: https://huggingface.co/datasets/future-technologies/Universal-Transformers-Dataset. | 4,566 | 4,625 | [
"task_categories:text-classification",
"task_categories:token-classification",
"task_categories:table-question-answering",
"task_categories:question-answering",
"task_categories:zero-shot-classification",
"task_categories:translation",
"task_categories:summarization",
"task_categories:feature-extraction",
"task_categories:text-generation",
"task_categories:text2text-generation",
"task_categories:fill-mask",
"task_categories:sentence-similarity",
"task_categories:text-to-speech",
"task_categories:text-to-audio",
"task_categories:automatic-speech-recognition",
"task_categories:audio-to-audio",
"task_categories:audio-classification",
"task_categories:voice-activity-detection",
"task_categories:depth-estimation",
"task_categories:image-classification",
"task_categories:object-detection",
"task_categories:image-segmentation",
"task_categories:text-to-image",
"task_categories:image-to-text",
"task_categories:image-to-image",
"task_categories:image-to-video",
"task_categories:unconditional-image-generation",
"task_categories:video-classification",
"task_categories:reinforcement-learning",
"task_categories:robotics",
"task_categories:tabular-classification",
"task_categories:tabular-regression",
"task_categories:tabular-to-text",
"task_categories:table-to-text",
"task_categories:multiple-choice",
"task_categories:text-retrieval",
"task_categories:time-series-forecasting",
"task_categories:text-to-video",
"task_categories:visual-question-answering",
"task_categories:zero-shot-image-classification",
"task_categories:graph-ml",
"task_categories:mask-generation",
"task_categories:zero-shot-object-detection",
"task_categories:text-to-3d",
"task_categories:image-to-3d",
"task_categories:image-feature-extraction",
"task_categories:video-text-to-text",
"language:ab",
"language:ace",
"language:ady",
"language:af",
"language:alt",
"language:am",
"language:ami",
"language:an",
"language:ang",
"language:anp",
"language:ar",
"language:arc",
"language:ary",
"language:arz",
"language:as",
"language:ast",
"language:atj",
"language:av",
"language:avk",
"language:awa",
"language:ay",
"language:az",
"language:azb",
"language:ba",
"language:ban",
"language:bar",
"language:bbc",
"language:bcl",
"language:be",
"language:bg",
"language:bh",
"language:bi",
"language:bjn",
"language:blk",
"language:bm",
"language:bn",
"language:bo",
"language:bpy",
"language:br",
"language:bs",
"language:bug",
"language:bxr",
"language:ca",
"language:cbk",
"language:cdo",
"language:ce",
"language:ceb",
"language:ch",
"language:chr",
"language:chy",
"language:ckb",
"language:co",
"language:cr",
"language:crh",
"language:cs",
"language:csb",
"language:cu",
"language:cv",
"language:cy",
"language:da",
"language:dag",
"language:de",
"language:dga",
"language:din",
"language:diq",
"language:dsb",
"language:dty",
"language:dv",
"language:dz",
"language:ee",
"language:el",
"language:eml",
"language:en",
"language:eo",
"language:es",
"language:et",
"language:eu",
"language:ext",
"language:fa",
"language:fat",
"language:ff",
"language:fi",
"language:fj",
"language:fo",
"language:fon",
"language:fr",
"language:frp",
"language:frr",
"language:fur",
"language:fy",
"language:ga",
"language:gag",
"language:gan",
"language:gcr",
"language:gd",
"language:gl",
"language:glk",
"language:gn",
"language:gom",
"language:gor",
"language:got",
"language:gpe",
"language:gsw",
"language:gu",
"language:guc",
"language:gur",
"language:guw",
"language:gv",
"language:ha",
"language:hak",
"language:haw",
"language:hbs",
"language:he",
"language:hi",
"language:hif",
"language:hr",
"language:hsb",
"language:ht",
"language:hu",
"language:hy",
"language:hyw",
"language:ia",
"language:id",
"language:ie",
"language:ig",
"language:ik",
"language:ilo",
"language:inh",
"language:io",
"language:is",
"language:it",
"language:iu",
"language:ja",
"language:jam",
"language:jbo",
"language:jv",
"language:ka",
"language:kaa",
"language:kab",
"language:kbd",
"language:kbp",
"language:kcg",
"language:kg",
"language:ki",
"language:kk",
"language:kl",
"language:km",
"language:kn",
"language:ko",
"language:koi",
"language:krc",
"language:ks",
"language:ksh",
"language:ku",
"language:kv",
"language:kw",
"language:ky",
"language:la",
"language:lad",
"language:lb",
"language:lbe",
"language:lez",
"language:lfn",
"language:lg",
"language:li",
"language:lij",
"language:lld",
"language:lmo",
"language:ln",
"language:lo",
"language:lt",
"language:ltg",
"language:lv",
"language:lzh",
"language:mad",
"language:mai",
"language:map",
"language:mdf",
"language:mg",
"language:mhr",
"language:mi",
"language:min",
"language:mk",
"language:ml",
"language:mn",
"language:mni",
"language:mnw",
"language:mr",
"language:mrj",
"language:ms",
"language:mt",
"language:mwl",
"language:my",
"language:myv",
"language:mzn",
"language:nah",
"language:nan",
"language:nap",
"language:nds",
"language:ne",
"language:new",
"language:nia",
"language:nl",
"language:nn",
"language:no",
"language:nov",
"language:nqo",
"language:nrf",
"language:nso",
"language:nv",
"language:ny",
"language:oc",
"language:olo",
"language:om",
"language:or",
"language:os",
"language:pa",
"language:pag",
"language:pam",
"language:pap",
"language:pcd",
"language:pcm",
"language:pdc",
"language:pfl",
"language:pi",
"language:pih",
"language:pl",
"language:pms",
"language:pnb",
"language:pnt",
"language:ps",
"language:pt",
"language:pwn",
"language:qu",
"language:rm",
"language:rmy",
"language:rn",
"language:ro",
"language:ru",
"language:rue",
"language:rup",
"language:rw",
"language:sa",
"language:sah",
"language:sat",
"language:sc",
"language:scn",
"language:sco",
"language:sd",
"language:se",
"language:sg",
"language:sgs",
"language:shi",
"language:shn",
"language:si",
"language:sk",
"language:skr",
"language:sl",
"language:sm",
"language:smn",
"language:sn",
"language:so",
"language:sq",
"language:sr",
"language:srn",
"language:ss",
"language:st",
"language:stq",
"language:su",
"language:sv",
"language:sw",
"language:szl",
"language:szy",
"language:ta",
"language:tay",
"language:tcy",
"language:te",
"language:tet",
"language:tg",
"language:th",
"language:ti",
"language:tk",
"language:tl",
"language:tly",
"language:tn",
"language:to",
"language:tpi",
"language:tr",
"language:trv",
"language:ts",
"language:tt",
"language:tum",
"language:tw",
"language:ty",
"language:tyv",
"language:udm",
"language:ug",
"language:uk",
"language:ur",
"language:uz",
"language:ve",
"language:vec",
"language:vep",
"language:vi",
"language:vls",
"language:vo",
"language:vro",
"language:wa",
"language:war",
"language:wo",
"language:wuu",
"language:xal",
"language:xh",
"language:xmf",
"language:yi",
"language:yo",
"language:yue",
"language:za",
"language:zea",
"language:zgh",
"language:zh",
"language:zu",
"size_categories:10M<n<100M",
"format:parquet",
"modality:text",
"modality:tabular",
"modality:video",
"modality:image",
"modality:audio",
"library:datasets",
"library:dask",
"library:mlcroissant",
"library:polars",
"region:us",
"tabular",
"video",
"image",
"audio",
"text-prompts",
"text",
"universal",
"transformer",
"database",
"massive-data",
"ai",
"training",
"huggingface",
"artificial-intelligence",
"machine-learning",
"deep-learning",
"transformers",
"neural-networks",
"multimodal",
"structured-data",
"tabular-data",
"nlp",
"computer-vision",
"speech-recognition",
"reinforcement-learning",
"time-series",
"large-language-models",
"generative-ai",
"huggingface-dataset",
"pytorch",
"tensorflow",
"jax",
"pretraining",
"finetuning",
"self-supervised-learning",
"few-shot-learning",
"zero-shot-learning",
"unsupervised-learning",
"meta-learning",
"diffusion-models"
] | 2025-02-01T09:50:54 | null | null |
67e428ff51af4261f7bed8c7 | nvidia/ClimbLab | nvidia | {"language": ["en"], "license": "cc-by-nc-4.0", "task_categories": ["text-generation"]} | false | null | 2025-04-21T19:02:49 | 28 | 28 | false | 9c3267aa7b4b4eda47fba41bbc95d99d072416c5 |
ClimbLab Dataset
🚀 Creating the highest-quality pre-training datasets for LLMs 🌟
📄 PAPER
🤗 CLIMBLAB
🤗 CLIMBMIX
🏠 HOMEPAGE
Figure 1: Continuously training a 1B model yields a 2.0% improvement over Llama-3.2-1B, demonstrating a more efficient scaling trend compared to prior models.
Figure 2: Pre-training a 1B model from scratch on ClimbMix shows better scaling effects than training on other datasets.… See the full description on the dataset page: https://huggingface.co/datasets/nvidia/ClimbLab. | 9,827 | 9,827 | [
"task_categories:text-generation",
"language:en",
"license:cc-by-nc-4.0",
"size_categories:1B<n<10B",
"format:parquet",
"library:datasets",
"library:dask",
"library:mlcroissant",
"library:polars",
"arxiv:2504.13161",
"region:us"
] | 2025-03-26T16:19:11 | null | null |
67d3479522a51de18affff22 | nvidia/Llama-Nemotron-Post-Training-Dataset | nvidia | {"license": "cc-by-4.0", "configs": [{"config_name": "SFT", "data_files": [{"split": "code", "path": "SFT/code/*.jsonl"}, {"split": "math", "path": "SFT/math/*.jsonl"}, {"split": "science", "path": "SFT/science/*.jsonl"}, {"split": "chat", "path": "SFT/chat/*.jsonl"}, {"split": "safety", "path": "SFT/safety/*.jsonl"}], "default": true}, {"config_name": "RL", "data_files": [{"split": "instruction_following", "path": "RL/instruction_following/*.jsonl"}]}]} | false | null | 2025-04-16T18:05:39 | 423 | 24 | false | ec1aa9f7d0832333c68283cd70a3df60bb8021db |
Llama-Nemotron-Post-Training-Dataset-v1.1 Release
Update [4/8/2025]:
v1.1: We are releasing an additional 2.2M Math and 500K Code Reasoning Data in support of our release of Llama-3.1-Nemotron-Ultra-253B-v1. 🎉
Data Overview
This dataset is a compilation of SFT and RL data that supports improvements of math, code, general reasoning, and instruction following capabilities of the original Llama instruct model, in support of NVIDIA’s release of… See the full description on the dataset page: https://huggingface.co/datasets/nvidia/Llama-Nemotron-Post-Training-Dataset. | 7,189 | 7,200 | [
"license:cc-by-4.0",
"size_categories:1M<n<10M",
"format:json",
"modality:text",
"library:datasets",
"library:dask",
"library:mlcroissant",
"region:us"
] | 2025-03-13T21:01:09 | null | null |
67e4291146baf23164358d53 | nvidia/ClimbMix | nvidia | {"language": ["en"], "license": "cc-by-nc-4.0", "task_categories": ["text-generation"], "configs": [{"config_name": "default", "data_files": "*.jsonl"}]} | false | null | 2025-04-22T16:32:05 | 21 | 21 | false | 65df6ea26b23832e564517346932bd975fd313c3 |
ClimbMix Dataset
🚀 Creating the highest-quality pre-training datasets for LLMs 🌟
📄 PAPER
🤗 CLIMBLAB
🤗 CLIMBMIX
🏠 HOMEPAGE
Figure 1: Continuously training a 1B model yields a 2.0% improvement over Llama-3.2-1B, demonstrating a more efficient scaling trend compared to prior models.
Figure 2: Pre-training a 1B model from scratch on ClimbMix shows better scaling effects than training on other datasets.… See the full description on the dataset page: https://huggingface.co/datasets/nvidia/ClimbMix. | 1,285 | 1,285 | [
"task_categories:text-generation",
"language:en",
"license:cc-by-nc-4.0",
"size_categories:100M<n<1B",
"format:json",
"modality:tabular",
"library:datasets",
"library:dask",
"library:mlcroissant",
"arxiv:2504.13161",
"region:us"
] | 2025-03-26T16:19:29 | null | null |
67ffe2dd906793fb908651af | bh2821/LightNovel5000 | bh2821 | {"license": "zlib", "task_categories": ["text-generation", "text2text-generation", "translation"], "language": ["zh"], "tags": ["Novel", "Light-Novel", "Japanese", "Chinese"], "size_categories": ["100M<n<1B"]} | false | null | 2025-04-16T20:25:38 | 24 | 21 | false | a9c8ce088c4c89321b1321654568dc99930938e5 |
Light novels translated in Chinese - crawled from public websites that do not prohibit crawlers
脚盆轻小说汉化 - 从未禁止爬虫的公共网站爬取
Version 0
版本 0
Contains around 1000 light novels, including PDF with illustration and txt text files.
It may be a good source of data that can be used to train your stylish LLM.
Kindly note that the author has partially clean the text BUT DOES NOT GUARANTEE that it is fully cleaned up.
包含约 1000 部轻小说,包括带插图的 PDF 和 txt 文本文件。… See the full description on the dataset page: https://huggingface.co/datasets/bh2821/LightNovel5000. | 646 | 646 | [
"task_categories:text-generation",
"task_categories:text2text-generation",
"task_categories:translation",
"language:zh",
"license:zlib",
"size_categories:1M<n<10M",
"format:text",
"modality:text",
"library:datasets",
"library:mlcroissant",
"region:us",
"Novel",
"Light-Novel",
"Japanese",
"Chinese"
] | 2025-04-16T17:03:25 | null | null |
67f9a5dde1bb509430e6af04 | openai/graphwalks | openai | {"license": "mit"} | false | null | 2025-04-14T17:22:42 | 62 | 18 | false | 6fe75ac25ccf55853294fe7995332d4f59d91bfb |
GraphWalks: a multi hop reasoning long context benchmark
In Graphwalks, the model is given a graph represented by its edge list and asked to perform an operation.
Example prompt:
You will be given a graph as a list of directed edges. All nodes are at least degree 1.
You will also get a description of an operation to perform on the graph.
Your job is to execute the operation on the graph and return the set of nodes that the operation results in.
If asked for a breadth-first search… See the full description on the dataset page: https://huggingface.co/datasets/openai/graphwalks. | 1,148 | 1,148 | [
"license:mit",
"size_categories:1K<n<10K",
"format:parquet",
"modality:text",
"library:datasets",
"library:dask",
"library:mlcroissant",
"library:polars",
"region:us"
] | 2025-04-11T23:29:33 | null | null |
67ffa22d0d123ebf23677e9e | JoeYing/ReTool-SFT | JoeYing | {"license": "apache-2.0"} | false | null | 2025-04-16T12:58:58 | 18 | 18 | false | 8b676fbb9f095830253943699f16035381a2baa1 | null | 406 | 406 | [
"license:apache-2.0",
"size_categories:1K<n<10K",
"format:parquet",
"modality:text",
"library:datasets",
"library:pandas",
"library:mlcroissant",
"library:polars",
"region:us"
] | 2025-04-16T12:27:25 | null | null |
67ddbf33273db7cb5c4f3f32 | UCSC-VLAA/MedReason | UCSC-VLAA | {"license": "apache-2.0", "tags": ["reasoning-datasets-competition", "reasoning-LLMs"]} | false | null | 2025-04-10T20:17:26 | 37 | 17 | false | a4bbf707e122021e74b098f542f2db97a89a9ead |
MedReason: Eliciting Factual Medical Reasoning Steps in LLMs via Knowledge Graphs
📃 Paper |🤗 MedReason-8B | 📚 MedReason Data
⚡Introduction
MedReason is a large-scale high-quality medical reasoning dataset designed to enable faithful and explainable medical problem-solving in large language models (LLMs).
We utilize a structured medical knowledge graph (KG) to convert clinical QA pairs into logical chains of reasoning, or “thinking paths”.
Our pipeline generates… See the full description on the dataset page: https://huggingface.co/datasets/UCSC-VLAA/MedReason. | 1,365 | 1,365 | [
"license:apache-2.0",
"size_categories:10K<n<100K",
"format:json",
"modality:text",
"library:datasets",
"library:pandas",
"library:mlcroissant",
"library:polars",
"arxiv:2504.00993",
"region:us",
"reasoning-datasets-competition",
"reasoning-LLMs"
] | 2025-03-21T19:34:11 | null | null |
67e72ca8f52d9d15f9d38a2a | facebook/PE-Video | facebook | {"license": "cc-by-nc-4.0"} | false | null | 2025-04-18T22:33:23 | 16 | 16 | false | 43a297dde47e2036721f259397df04b3c338d002 |
PE Video Dataset (PVD)
[📃 Tech Report]
[📂 Github]
The PE Video Dataset (PVD) is a large-scale collection of 1 million diverse videos, featuring 120,000+ expertly annotated clips. The dataset was introduced in our paper "Perception Encoder".
Overview
PE Video Dataset (PVD) comprises 1M high quality and diverse videos. Among them, 120K videos are accompanied by automated and human-verified annotations. and all videos are accompanied with video description and keywords.… See the full description on the dataset page: https://huggingface.co/datasets/facebook/PE-Video. | 4,525 | 4,525 | [
"license:cc-by-nc-4.0",
"size_categories:100K<n<1M",
"format:webdataset",
"modality:text",
"library:datasets",
"library:webdataset",
"library:mlcroissant",
"arxiv:2504.13181",
"region:us"
] | 2025-03-28T23:11:36 | null | null |
6791fcbb49c4df6d798ca7c9 | cais/hle | cais | {"license": "mit", "dataset_info": {"features": [{"name": "id", "dtype": "string"}, {"name": "question", "dtype": "string"}, {"name": "image", "dtype": "string"}, {"name": "image_preview", "dtype": "image"}, {"name": "answer", "dtype": "string"}, {"name": "answer_type", "dtype": "string"}, {"name": "author_name", "dtype": "string"}, {"name": "rationale", "dtype": "string"}, {"name": "rationale_image", "dtype": "image"}, {"name": "raw_subject", "dtype": "string"}, {"name": "category", "dtype": "string"}, {"name": "canary", "dtype": "string"}], "splits": [{"name": "test", "num_bytes": 284635618, "num_examples": 2500}], "download_size": 274582371, "dataset_size": 284635618}, "configs": [{"config_name": "default", "data_files": [{"split": "test", "path": "data/test-*"}]}]} | false | null | 2025-04-04T04:00:14 | 316 | 15 | false | 1e33bd2d1346480b397ad94845067c4a088a33d3 |
Humanity's Last Exam
🌐 Website | 📄 Paper | GitHub
Center for AI Safety & Scale AI
Humanity's Last Exam (HLE) is a multi-modal benchmark at the frontier of human knowledge, designed to be the final closed-ended academic benchmark of its kind with broad subject coverage. Humanity's Last Exam consists of 2,500 questions across dozens of subjects, including mathematics, humanities, and the natural sciences. HLE is developed globally by subject-matter experts and consists of… See the full description on the dataset page: https://huggingface.co/datasets/cais/hle. | 9,336 | 22,465 | [
"license:mit",
"size_categories:1K<n<10K",
"format:parquet",
"modality:image",
"modality:text",
"library:datasets",
"library:pandas",
"library:mlcroissant",
"library:polars",
"region:us"
] | 2025-01-23T08:24:27 | null | null |
67fa39f24a13bd97755f08db | Skywork/Skywork-OR1-RL-Data | Skywork | {"dataset_info": {"features": [{"name": "data_source", "dtype": "string"}, {"name": "prompt", "list": [{"name": "content", "dtype": "string"}, {"name": "role", "dtype": "string"}]}, {"name": "ability", "dtype": "string"}, {"name": "reward_model", "struct": [{"name": "ground_truth", "dtype": "string"}, {"name": "style", "dtype": "string"}]}, {"name": "extra_info", "struct": [{"name": "index", "dtype": "int64"}, {"name": "model_difficulty", "struct": [{"name": "DeepSeek-R1-Distill-Qwen-1.5B", "dtype": "int64"}, {"name": "DeepSeek-R1-Distill-Qwen-32B", "dtype": "int64"}, {"name": "DeepSeek-R1-Distill-Qwen-7B", "dtype": "int64"}]}]}], "splits": [{"name": "math", "num_bytes": 40461845, "num_examples": 105055}, {"name": "code", "num_bytes": 1474827100, "num_examples": 14057}], "download_size": 823104116, "dataset_size": 1515288945}, "configs": [{"config_name": "default", "data_files": [{"split": "math", "path": "data/math-*"}, {"split": "code", "path": "data/code-*"}]}]} | false | null | 2025-04-15T08:31:20 | 27 | 15 | false | d3dd0aaddf1f74f14d37331b574ebf5746670645 |
🤔 Skywork-OR1-RL-Data
🔥 News
April 15, 2025: We are excited to release our RL training dataset Skywork-OR1-RL-Data
For our final training phase, we filtered problems based on their difficulty levels (0-16, higher values indicate harder problems) relative to specific model variants (DeepSeek-R1-Distill-Qwen-{1.5,7,32}B. For each model variant, we excluded problems with difficulty values of 0 and 16 specific to that model from its training data.You can… See the full description on the dataset page: https://huggingface.co/datasets/Skywork/Skywork-OR1-RL-Data. | 1,195 | 1,195 | [
"size_categories:100K<n<1M",
"format:parquet",
"modality:text",
"library:datasets",
"library:dask",
"library:mlcroissant",
"library:polars",
"region:us"
] | 2025-04-12T10:01:22 | null | null |
6801c3764dc338207b777a11 | a-m-team/AM-DeepSeek-Distilled-40M | a-m-team | {"license": "cc-by-nc-4.0", "task_categories": ["text-generation"], "language": ["zh", "en"], "tags": ["code", "math", "science", "instruction follow", "reasoning", "thinking", "deepseek-r1", "distill"], "size_categories": ["35M<n<45M"], "configs": [{"config_name": "code_1.5b_1pass", "data_files": "code_1.5b_1pass.jsonl", "features": [{"name": "question", "dtype": "string"}, {"name": "answer", "dtype": "string"}, {"name": "question_source", "dtype": "string"}, {"name": "answer_source", "dtype": "string"}, {"name": "category", "dtype": "string"}, {"name": "ground_truth", "dtype": "string"}, {"name": "test_case", "dtype": "string"}, {"name": "instruction_constrain", "dtype": "string"}, {"name": "pass_rate_r1", "dtype": "float32"}, {"name": "pass_rate_7b", "dtype": "float32"}, {"name": "pass_rate_1.5b", "dtype": "float32"}, {"name": "verify_score", "dtype": "float32"}, {"name": "ppl", "dtype": "float32"}, {"name": "model_name", "dtype": "string"}]}, {"config_name": "code_1.5b_2pass", "data_files": "code_1.5b_2pass.jsonl", "features": [{"name": "question", "dtype": "string"}, {"name": "answer", "dtype": "string"}, {"name": "question_source", "dtype": "string"}, {"name": "answer_source", "dtype": "string"}, {"name": "category", "dtype": "string"}, {"name": "ground_truth", "dtype": "string"}, {"name": "test_case", "dtype": "string"}, {"name": "instruction_constrain", "dtype": "string"}, {"name": "pass_rate_r1", "dtype": "float32"}, {"name": "pass_rate_7b", "dtype": "float32"}, {"name": "pass_rate_1.5b", "dtype": "float32"}, {"name": "verify_score", "dtype": "float32"}, {"name": "ppl", "dtype": "float32"}, {"name": "model_name", "dtype": "string"}]}, {"config_name": "code_1.5b_3pass", "data_files": "code_1.5b_3pass.jsonl", "features": [{"name": "question", "dtype": "string"}, {"name": "answer", "dtype": "string"}, {"name": "question_source", "dtype": "string"}, {"name": "answer_source", "dtype": "string"}, {"name": "category", "dtype": "string"}, {"name": "ground_truth", "dtype": "string"}, {"name": "test_case", "dtype": "string"}, {"name": "instruction_constrain", "dtype": "string"}, {"name": "pass_rate_r1", "dtype": "float32"}, {"name": "pass_rate_7b", "dtype": "float32"}, {"name": "pass_rate_1.5b", "dtype": "float32"}, {"name": "verify_score", "dtype": "float32"}, {"name": "ppl", "dtype": "float32"}, {"name": "model_name", "dtype": "string"}]}, {"config_name": "code_1.5b_4pass", "data_files": "code_1.5b_4pass.jsonl", "features": [{"name": "question", "dtype": "string"}, {"name": "answer", "dtype": "string"}, {"name": "question_source", "dtype": "string"}, {"name": "answer_source", "dtype": "string"}, {"name": "category", "dtype": "string"}, {"name": "ground_truth", "dtype": "string"}, {"name": "test_case", "dtype": "string"}, {"name": "instruction_constrain", "dtype": "string"}, {"name": "pass_rate_r1", "dtype": "float32"}, {"name": "pass_rate_7b", "dtype": "float32"}, {"name": "pass_rate_1.5b", "dtype": "float32"}, {"name": "verify_score", "dtype": "float32"}, {"name": "ppl", "dtype": "float32"}, {"name": "model_name", "dtype": "string"}]}, {"config_name": "code_7b_1pass", "data_files": "code_7b_1pass.jsonl", "features": [{"name": "question", "dtype": "string"}, {"name": "answer", "dtype": "string"}, {"name": "question_source", "dtype": "string"}, {"name": "answer_source", "dtype": "string"}, {"name": "category", "dtype": "string"}, {"name": "ground_truth", "dtype": "string"}, {"name": "test_case", "dtype": "string"}, {"name": "instruction_constrain", "dtype": "string"}, {"name": "pass_rate_r1", "dtype": "float32"}, {"name": "pass_rate_7b", "dtype": "float32"}, {"name": "pass_rate_1.5b", "dtype": "float32"}, {"name": "verify_score", "dtype": "float32"}, {"name": "ppl", "dtype": "float32"}, {"name": "model_name", "dtype": "string"}]}, {"config_name": "code_7b_2pass", "data_files": "code_7b_2pass.jsonl", "features": [{"name": "question", "dtype": "string"}, {"name": "answer", "dtype": "string"}, {"name": "question_source", "dtype": "string"}, {"name": "answer_source", "dtype": "string"}, {"name": "category", "dtype": "string"}, {"name": "ground_truth", "dtype": "string"}, {"name": "test_case", "dtype": "string"}, {"name": "instruction_constrain", "dtype": "string"}, {"name": "pass_rate_r1", "dtype": "float32"}, {"name": "pass_rate_7b", "dtype": "float32"}, {"name": "pass_rate_1.5b", "dtype": "float32"}, {"name": "verify_score", "dtype": "float32"}, {"name": "ppl", "dtype": "float32"}, {"name": "model_name", "dtype": "string"}]}, {"config_name": "code_7b_3pass", "data_files": "code_7b_3pass.jsonl", "features": [{"name": "question", "dtype": "string"}, {"name": "answer", "dtype": "string"}, {"name": "question_source", "dtype": "string"}, {"name": "answer_source", "dtype": "string"}, {"name": "category", "dtype": "string"}, {"name": "ground_truth", "dtype": "string"}, {"name": "test_case", "dtype": "string"}, {"name": "instruction_constrain", "dtype": "string"}, {"name": "pass_rate_r1", "dtype": "float32"}, {"name": "pass_rate_7b", "dtype": "float32"}, {"name": "pass_rate_1.5b", "dtype": "float32"}, {"name": "verify_score", "dtype": "float32"}, {"name": "ppl", "dtype": "float32"}, {"name": "model_name", "dtype": "string"}]}, {"config_name": "code_7b_4pass", "data_files": "code_7b_4pass.jsonl", "features": [{"name": "question", "dtype": "string"}, {"name": "answer", "dtype": "string"}, {"name": "question_source", "dtype": "string"}, {"name": "answer_source", "dtype": "string"}, {"name": "category", "dtype": "string"}, {"name": "ground_truth", "dtype": "string"}, {"name": "test_case", "dtype": "string"}, {"name": "instruction_constrain", "dtype": "string"}, {"name": "pass_rate_r1", "dtype": "float32"}, {"name": "pass_rate_7b", "dtype": "float32"}, {"name": "pass_rate_1.5b", "dtype": "float32"}, {"name": "verify_score", "dtype": "float32"}, {"name": "ppl", "dtype": "float32"}, {"name": "model_name", "dtype": "string"}]}, {"config_name": "code_r1_1pass", "data_files": "code_r1_1pass.jsonl", "features": [{"name": "question", "dtype": "string"}, {"name": "answer", "dtype": "string"}, {"name": "question_source", "dtype": "string"}, {"name": "answer_source", "dtype": "string"}, {"name": "category", "dtype": "string"}, {"name": "ground_truth", "dtype": "string"}, {"name": "test_case", "dtype": "string"}, {"name": "instruction_constrain", "dtype": "string"}, {"name": "pass_rate_r1", "dtype": "float32"}, {"name": "pass_rate_7b", "dtype": "float32"}, {"name": "pass_rate_1.5b", "dtype": "float32"}, {"name": "verify_score", "dtype": "float32"}, {"name": "ppl", "dtype": "float32"}, {"name": "model_name", "dtype": "string"}]}, {"config_name": "code_r1_2pass", "data_files": "code_r1_2pass.jsonl", "features": [{"name": "question", "dtype": "string"}, {"name": "answer", "dtype": "string"}, {"name": "question_source", "dtype": "string"}, {"name": "answer_source", "dtype": "string"}, {"name": "category", "dtype": "string"}, {"name": "ground_truth", "dtype": "string"}, {"name": "test_case", "dtype": "string"}, {"name": "instruction_constrain", "dtype": "string"}, {"name": "pass_rate_r1", "dtype": "float32"}, {"name": "pass_rate_7b", "dtype": "float32"}, {"name": "pass_rate_1.5b", "dtype": "float32"}, {"name": "verify_score", "dtype": "float32"}, {"name": "ppl", "dtype": "float32"}, {"name": "model_name", "dtype": "string"}]}, {"config_name": "code_r1_3pass", "data_files": "code_r1_3pass.jsonl", "features": [{"name": "question", "dtype": "string"}, {"name": "answer", "dtype": "string"}, {"name": "question_source", "dtype": "string"}, {"name": "answer_source", "dtype": "string"}, {"name": "category", "dtype": "string"}, {"name": "ground_truth", "dtype": "string"}, {"name": "test_case", "dtype": "string"}, {"name": "instruction_constrain", "dtype": "string"}, {"name": "pass_rate_r1", "dtype": "float32"}, {"name": "pass_rate_7b", "dtype": "float32"}, {"name": "pass_rate_1.5b", "dtype": "float32"}, {"name": "verify_score", "dtype": "float32"}, {"name": "ppl", "dtype": "float32"}, {"name": "model_name", "dtype": "string"}]}, {"config_name": "code_r1_4pass", "data_files": "code_r1_4pass.jsonl", "features": [{"name": "question", "dtype": "string"}, {"name": "answer", "dtype": "string"}, {"name": "question_source", "dtype": "string"}, {"name": "answer_source", "dtype": "string"}, {"name": "category", "dtype": "string"}, {"name": "ground_truth", "dtype": "string"}, {"name": "test_case", "dtype": "string"}, {"name": "instruction_constrain", "dtype": "string"}, {"name": "pass_rate_r1", "dtype": "float32"}, {"name": "pass_rate_7b", "dtype": "float32"}, {"name": "pass_rate_1.5b", "dtype": "float32"}, {"name": "verify_score", "dtype": "float32"}, {"name": "ppl", "dtype": "float32"}, {"name": "model_name", "dtype": "string"}]}, {"config_name": "if_1.5b_1pass", "data_files": "if_1.5b_1pass.jsonl", "features": [{"name": "question", "dtype": "string"}, {"name": "answer", "dtype": "string"}, {"name": "question_source", "dtype": "string"}, {"name": "answer_source", "dtype": "string"}, {"name": "category", "dtype": "string"}, {"name": "ground_truth", "dtype": "string"}, {"name": "test_case", "dtype": "string"}, {"name": "instruction_constrain", "dtype": "string"}, {"name": "pass_rate_r1", "dtype": "float32"}, {"name": "pass_rate_7b", "dtype": "float32"}, {"name": "pass_rate_1.5b", "dtype": "float32"}, {"name": "verify_score", "dtype": "float32"}, {"name": "ppl", "dtype": "float32"}, {"name": "model_name", "dtype": "string"}]}, {"config_name": "if_1.5b_2pass", "data_files": "if_1.5b_2pass.jsonl", "features": [{"name": "question", "dtype": "string"}, {"name": "answer", "dtype": "string"}, {"name": "question_source", "dtype": "string"}, {"name": "answer_source", "dtype": "string"}, {"name": "category", "dtype": "string"}, {"name": "ground_truth", "dtype": "string"}, {"name": "test_case", "dtype": "string"}, {"name": "instruction_constrain", "dtype": "string"}, {"name": "pass_rate_r1", "dtype": "float32"}, {"name": "pass_rate_7b", "dtype": "float32"}, {"name": "pass_rate_1.5b", "dtype": "float32"}, {"name": "verify_score", "dtype": "float32"}, {"name": "ppl", "dtype": "float32"}, {"name": "model_name", "dtype": "string"}]}, {"config_name": "if_1.5b_3pass", "data_files": "if_1.5b_3pass.jsonl", "features": [{"name": "question", "dtype": "string"}, {"name": "answer", "dtype": "string"}, {"name": "question_source", "dtype": "string"}, {"name": "answer_source", "dtype": "string"}, {"name": "category", "dtype": "string"}, {"name": "ground_truth", "dtype": "string"}, {"name": "test_case", "dtype": "string"}, {"name": "instruction_constrain", "dtype": "string"}, {"name": "pass_rate_r1", "dtype": "float32"}, {"name": "pass_rate_7b", "dtype": "float32"}, {"name": "pass_rate_1.5b", "dtype": "float32"}, {"name": "verify_score", "dtype": "float32"}, {"name": "ppl", "dtype": "float32"}, {"name": "model_name", "dtype": "string"}]}, {"config_name": "if_1.5b_4pass", "data_files": "if_1.5b_4pass.jsonl", "features": [{"name": "question", "dtype": "string"}, {"name": "answer", "dtype": "string"}, {"name": "question_source", "dtype": "string"}, {"name": "answer_source", "dtype": "string"}, {"name": "category", "dtype": "string"}, {"name": "ground_truth", "dtype": "string"}, {"name": "test_case", "dtype": "string"}, {"name": "instruction_constrain", "dtype": "string"}, {"name": "pass_rate_r1", "dtype": "float32"}, {"name": "pass_rate_7b", "dtype": "float32"}, {"name": "pass_rate_1.5b", "dtype": "float32"}, {"name": "verify_score", "dtype": "float32"}, {"name": "ppl", "dtype": "float32"}, {"name": "model_name", "dtype": "string"}]}, {"config_name": "if_7b_1pass", "data_files": "if_7b_1pass.jsonl", "features": [{"name": "question", "dtype": "string"}, {"name": "answer", "dtype": "string"}, {"name": "question_source", "dtype": "string"}, {"name": "answer_source", "dtype": "string"}, {"name": "category", "dtype": "string"}, {"name": "ground_truth", "dtype": "string"}, {"name": "test_case", "dtype": "string"}, {"name": "instruction_constrain", "dtype": "string"}, {"name": "pass_rate_r1", "dtype": "float32"}, {"name": "pass_rate_7b", "dtype": "float32"}, {"name": "pass_rate_1.5b", "dtype": "float32"}, {"name": "verify_score", "dtype": "float32"}, {"name": "ppl", "dtype": "float32"}, {"name": "model_name", "dtype": "string"}]}, {"config_name": "if_7b_2pass", "data_files": "if_7b_2pass.jsonl", "features": [{"name": "question", "dtype": "string"}, {"name": "answer", "dtype": "string"}, {"name": "question_source", "dtype": "string"}, {"name": "answer_source", "dtype": "string"}, {"name": "category", "dtype": "string"}, {"name": "ground_truth", "dtype": "string"}, {"name": "test_case", "dtype": "string"}, {"name": "instruction_constrain", "dtype": "string"}, {"name": "pass_rate_r1", "dtype": "float32"}, {"name": "pass_rate_7b", "dtype": "float32"}, {"name": "pass_rate_1.5b", "dtype": "float32"}, {"name": "verify_score", "dtype": "float32"}, {"name": "ppl", "dtype": "float32"}, {"name": "model_name", "dtype": "string"}]}, {"config_name": "if_7b_3pass", "data_files": "if_7b_3pass.jsonl", "features": [{"name": "question", "dtype": "string"}, {"name": "answer", "dtype": "string"}, {"name": "question_source", "dtype": "string"}, {"name": "answer_source", "dtype": "string"}, {"name": "category", "dtype": "string"}, {"name": "ground_truth", "dtype": "string"}, {"name": "test_case", "dtype": "string"}, {"name": "instruction_constrain", "dtype": "string"}, {"name": "pass_rate_r1", "dtype": "float32"}, {"name": "pass_rate_7b", "dtype": "float32"}, {"name": "pass_rate_1.5b", "dtype": "float32"}, {"name": "verify_score", "dtype": "float32"}, {"name": "ppl", "dtype": "float32"}, {"name": "model_name", "dtype": "string"}]}, {"config_name": "if_7b_4pass", "data_files": "if_7b_4pass.jsonl", "features": [{"name": "question", "dtype": "string"}, {"name": "answer", "dtype": "string"}, {"name": "question_source", "dtype": "string"}, {"name": "answer_source", "dtype": "string"}, {"name": "category", "dtype": "string"}, {"name": "ground_truth", "dtype": "string"}, {"name": "test_case", "dtype": "string"}, {"name": "instruction_constrain", "dtype": "string"}, {"name": "pass_rate_r1", "dtype": "float32"}, {"name": "pass_rate_7b", "dtype": "float32"}, {"name": "pass_rate_1.5b", "dtype": "float32"}, {"name": "verify_score", "dtype": "float32"}, {"name": "ppl", "dtype": "float32"}, {"name": "model_name", "dtype": "string"}]}, {"config_name": "if_r1_1pass", "data_files": "if_r1_1pass.jsonl", "features": [{"name": "question", "dtype": "string"}, {"name": "answer", "dtype": "string"}, {"name": "question_source", "dtype": "string"}, {"name": "answer_source", "dtype": "string"}, {"name": "category", "dtype": "string"}, {"name": "ground_truth", "dtype": "string"}, {"name": "test_case", "dtype": "string"}, {"name": "instruction_constrain", "dtype": "string"}, {"name": "pass_rate_r1", "dtype": "float32"}, {"name": "pass_rate_7b", "dtype": "float32"}, {"name": "pass_rate_1.5b", "dtype": "float32"}, {"name": "verify_score", "dtype": "float32"}, {"name": "ppl", "dtype": "float32"}, {"name": "model_name", "dtype": "string"}]}, {"config_name": "if_r1_2pass", "data_files": "if_r1_2pass.jsonl", "features": [{"name": "question", "dtype": "string"}, {"name": "answer", "dtype": "string"}, {"name": "question_source", "dtype": "string"}, {"name": "answer_source", "dtype": "string"}, {"name": "category", "dtype": "string"}, {"name": "ground_truth", "dtype": "string"}, {"name": "test_case", "dtype": "string"}, {"name": "instruction_constrain", "dtype": "string"}, {"name": "pass_rate_r1", "dtype": "float32"}, {"name": "pass_rate_7b", "dtype": "float32"}, {"name": "pass_rate_1.5b", "dtype": "float32"}, {"name": "verify_score", "dtype": "float32"}, {"name": "ppl", "dtype": "float32"}, {"name": "model_name", "dtype": "string"}]}, {"config_name": "if_r1_3pass", "data_files": "if_r1_3pass.jsonl", "features": [{"name": "question", "dtype": "string"}, {"name": "answer", "dtype": "string"}, {"name": "question_source", "dtype": "string"}, {"name": "answer_source", "dtype": "string"}, {"name": "category", "dtype": "string"}, {"name": "ground_truth", "dtype": "string"}, {"name": "test_case", "dtype": "string"}, {"name": "instruction_constrain", "dtype": "string"}, {"name": "pass_rate_r1", "dtype": "float32"}, {"name": "pass_rate_7b", "dtype": "float32"}, {"name": "pass_rate_1.5b", "dtype": "float32"}, {"name": "verify_score", "dtype": "float32"}, {"name": "ppl", "dtype": "float32"}, {"name": "model_name", "dtype": "string"}]}, {"config_name": "if_r1_4pass", "data_files": "if_r1_4pass.jsonl", "features": [{"name": "question", "dtype": "string"}, {"name": "answer", "dtype": "string"}, {"name": "question_source", "dtype": "string"}, {"name": "answer_source", "dtype": "string"}, {"name": "category", "dtype": "string"}, {"name": "ground_truth", "dtype": "string"}, {"name": "test_case", "dtype": "string"}, {"name": "instruction_constrain", "dtype": "string"}, {"name": "pass_rate_r1", "dtype": "float32"}, {"name": "pass_rate_7b", "dtype": "float32"}, {"name": "pass_rate_1.5b", "dtype": "float32"}, {"name": "verify_score", "dtype": "float32"}, {"name": "ppl", "dtype": "float32"}, {"name": "model_name", "dtype": "string"}]}, {"config_name": "math_1.5b_1pass", "data_files": "math_1.5b_1pass.jsonl", "features": [{"name": "question", "dtype": "string"}, {"name": "answer", "dtype": "string"}, {"name": "question_source", "dtype": "string"}, {"name": "answer_source", "dtype": "string"}, {"name": "category", "dtype": "string"}, {"name": "ground_truth", "dtype": "string"}, {"name": "test_case", "dtype": "string"}, {"name": "instruction_constrain", "dtype": "string"}, {"name": "pass_rate_r1", "dtype": "float32"}, {"name": "pass_rate_7b", "dtype": "float32"}, {"name": "pass_rate_1.5b", "dtype": "float32"}, {"name": "verify_score", "dtype": "float32"}, {"name": "ppl", "dtype": "float32"}, {"name": "model_name", "dtype": "string"}]}, {"config_name": "math_1.5b_2pass", "data_files": "math_1.5b_2pass.jsonl", "features": [{"name": "question", "dtype": "string"}, {"name": "answer", "dtype": "string"}, {"name": "question_source", "dtype": "string"}, {"name": "answer_source", "dtype": "string"}, {"name": "category", "dtype": "string"}, {"name": "ground_truth", "dtype": "string"}, {"name": "test_case", "dtype": "string"}, {"name": "instruction_constrain", "dtype": "string"}, {"name": "pass_rate_r1", "dtype": "float32"}, {"name": "pass_rate_7b", "dtype": "float32"}, {"name": "pass_rate_1.5b", "dtype": "float32"}, {"name": "verify_score", "dtype": "float32"}, {"name": "ppl", "dtype": "float32"}, {"name": "model_name", "dtype": "string"}]}, {"config_name": "math_1.5b_3pass", "data_files": "math_1.5b_3pass.jsonl", "features": [{"name": "question", "dtype": "string"}, {"name": "answer", "dtype": "string"}, {"name": "question_source", "dtype": "string"}, {"name": "answer_source", "dtype": "string"}, {"name": "category", "dtype": "string"}, {"name": "ground_truth", "dtype": "string"}, {"name": "test_case", "dtype": "string"}, {"name": "instruction_constrain", "dtype": "string"}, {"name": "pass_rate_r1", "dtype": "float32"}, {"name": "pass_rate_7b", "dtype": "float32"}, {"name": "pass_rate_1.5b", "dtype": "float32"}, {"name": "verify_score", "dtype": "float32"}, {"name": "ppl", "dtype": "float32"}, {"name": "model_name", "dtype": "string"}]}, {"config_name": "math_1.5b_4pass", "data_files": "math_1.5b_4pass.jsonl", "features": [{"name": "question", "dtype": "string"}, {"name": "answer", "dtype": "string"}, {"name": "question_source", "dtype": "string"}, {"name": "answer_source", "dtype": "string"}, {"name": "category", "dtype": "string"}, {"name": "ground_truth", "dtype": "string"}, {"name": "test_case", "dtype": "string"}, {"name": "instruction_constrain", "dtype": "string"}, {"name": "pass_rate_r1", "dtype": "float32"}, {"name": "pass_rate_7b", "dtype": "float32"}, {"name": "pass_rate_1.5b", "dtype": "float32"}, {"name": "verify_score", "dtype": "float32"}, {"name": "ppl", "dtype": "float32"}, {"name": "model_name", "dtype": "string"}]}, {"config_name": "math_7b_1pass", "data_files": "math_7b_1pass.jsonl", "features": [{"name": "question", "dtype": "string"}, {"name": "answer", "dtype": "string"}, {"name": "question_source", "dtype": "string"}, {"name": "answer_source", "dtype": "string"}, {"name": "category", "dtype": "string"}, {"name": "ground_truth", "dtype": "string"}, {"name": "test_case", "dtype": "string"}, {"name": "instruction_constrain", "dtype": "string"}, {"name": "pass_rate_r1", "dtype": "float32"}, {"name": "pass_rate_7b", "dtype": "float32"}, {"name": "pass_rate_1.5b", "dtype": "float32"}, {"name": "verify_score", "dtype": "float32"}, {"name": "ppl", "dtype": "float32"}, {"name": "model_name", "dtype": "string"}]}, {"config_name": "math_7b_2pass", "data_files": "math_7b_2pass.jsonl", "features": [{"name": "question", "dtype": "string"}, {"name": "answer", "dtype": "string"}, {"name": "question_source", "dtype": "string"}, {"name": "answer_source", "dtype": "string"}, {"name": "category", "dtype": "string"}, {"name": "ground_truth", "dtype": "string"}, {"name": "test_case", "dtype": "string"}, {"name": "instruction_constrain", "dtype": "string"}, {"name": "pass_rate_r1", "dtype": "float32"}, {"name": "pass_rate_7b", "dtype": "float32"}, {"name": "pass_rate_1.5b", "dtype": "float32"}, {"name": "verify_score", "dtype": "float32"}, {"name": "ppl", "dtype": "float32"}, {"name": "model_name", "dtype": "string"}]}, {"config_name": "math_7b_3pass", "data_files": "math_7b_3pass.jsonl", "features": [{"name": "question", "dtype": "string"}, {"name": "answer", "dtype": "string"}, {"name": "question_source", "dtype": "string"}, {"name": "answer_source", "dtype": "string"}, {"name": "category", "dtype": "string"}, {"name": "ground_truth", "dtype": "string"}, {"name": "test_case", "dtype": "string"}, {"name": "instruction_constrain", "dtype": "string"}, {"name": "pass_rate_r1", "dtype": "float32"}, {"name": "pass_rate_7b", "dtype": "float32"}, {"name": "pass_rate_1.5b", "dtype": "float32"}, {"name": "verify_score", "dtype": "float32"}, {"name": "ppl", "dtype": "float32"}, {"name": "model_name", "dtype": "string"}]}, {"config_name": "math_7b_4pass", "data_files": "math_7b_4pass.jsonl", "features": [{"name": "question", "dtype": "string"}, {"name": "answer", "dtype": "string"}, {"name": "question_source", "dtype": "string"}, {"name": "answer_source", "dtype": "string"}, {"name": "category", "dtype": "string"}, {"name": "ground_truth", "dtype": "string"}, {"name": "test_case", "dtype": "string"}, {"name": "instruction_constrain", "dtype": "string"}, {"name": "pass_rate_r1", "dtype": "float32"}, {"name": "pass_rate_7b", "dtype": "float32"}, {"name": "pass_rate_1.5b", "dtype": "float32"}, {"name": "verify_score", "dtype": "float32"}, {"name": "ppl", "dtype": "float32"}, {"name": "model_name", "dtype": "string"}]}, {"config_name": "math_r1_1pass", "data_files": "math_r1_1pass.jsonl", "features": [{"name": "question", "dtype": "string"}, {"name": "answer", "dtype": "string"}, {"name": "question_source", "dtype": "string"}, {"name": "answer_source", "dtype": "string"}, {"name": "category", "dtype": "string"}, {"name": "ground_truth", "dtype": "string"}, {"name": "test_case", "dtype": "string"}, {"name": "instruction_constrain", "dtype": "string"}, {"name": "pass_rate_r1", "dtype": "float32"}, {"name": "pass_rate_7b", "dtype": "float32"}, {"name": "pass_rate_1.5b", "dtype": "float32"}, {"name": "verify_score", "dtype": "float32"}, {"name": "ppl", "dtype": "float32"}, {"name": "model_name", "dtype": "string"}]}, {"config_name": "math_r1_2pass", "data_files": "math_r1_2pass.jsonl", "features": [{"name": "question", "dtype": "string"}, {"name": "answer", "dtype": "string"}, {"name": "question_source", "dtype": "string"}, {"name": "answer_source", "dtype": "string"}, {"name": "category", "dtype": "string"}, {"name": "ground_truth", "dtype": "string"}, {"name": "test_case", "dtype": "string"}, {"name": "instruction_constrain", "dtype": "string"}, {"name": "pass_rate_r1", "dtype": "float32"}, {"name": "pass_rate_7b", "dtype": "float32"}, {"name": "pass_rate_1.5b", "dtype": "float32"}, {"name": "verify_score", "dtype": "float32"}, {"name": "ppl", "dtype": "float32"}, {"name": "model_name", "dtype": "string"}]}, {"config_name": "math_r1_3pass", "data_files": "math_r1_3pass.jsonl", "features": [{"name": "question", "dtype": "string"}, {"name": "answer", "dtype": "string"}, {"name": "question_source", "dtype": "string"}, {"name": "answer_source", "dtype": "string"}, {"name": "category", "dtype": "string"}, {"name": "ground_truth", "dtype": "string"}, {"name": "test_case", "dtype": "string"}, {"name": "instruction_constrain", "dtype": "string"}, {"name": "pass_rate_r1", "dtype": "float32"}, {"name": "pass_rate_7b", "dtype": "float32"}, {"name": "pass_rate_1.5b", "dtype": "float32"}, {"name": "verify_score", "dtype": "float32"}, {"name": "ppl", "dtype": "float32"}, {"name": "model_name", "dtype": "string"}]}, {"config_name": "math_r1_4pass", "data_files": "math_r1_4pass.jsonl", "features": [{"name": "question", "dtype": "string"}, {"name": "answer", "dtype": "string"}, {"name": "question_source", "dtype": "string"}, {"name": "answer_source", "dtype": "string"}, {"name": "category", "dtype": "string"}, {"name": "ground_truth", "dtype": "string"}, {"name": "test_case", "dtype": "string"}, {"name": "instruction_constrain", "dtype": "string"}, {"name": "pass_rate_r1", "dtype": "float32"}, {"name": "pass_rate_7b", "dtype": "float32"}, {"name": "pass_rate_1.5b", "dtype": "float32"}, {"name": "verify_score", "dtype": "float32"}, {"name": "ppl", "dtype": "float32"}, {"name": "model_name", "dtype": "string"}]}]} | false | null | 2025-04-20T04:59:03 | 15 | 15 | false | b456b3e8f37918e80a771f7d9722fd45cf0452e3 | For more open-source datasets, models, and methodologies, please visit our GitHub repository.
Long reasoning processes have demonstrated significant effectiveness in enhancing model performance across domains such as mathematics,
code generation, and reasoning. Recent studies have highlighted that training outcomes are markedly influenced by task difficulty.
Although many existing methods utilize a large language model (LLM) to rate the task difficulty,
our empirical analysis reveals that… See the full description on the dataset page: https://huggingface.co/datasets/a-m-team/AM-DeepSeek-Distilled-40M. | 1,044 | 1,044 | [
"task_categories:text-generation",
"language:zh",
"language:en",
"license:cc-by-nc-4.0",
"size_categories:10M<n<100M",
"format:json",
"modality:tabular",
"modality:text",
"library:datasets",
"library:pandas",
"library:mlcroissant",
"library:polars",
"region:us",
"code",
"math",
"science",
"instruction follow",
"reasoning",
"thinking",
"deepseek-r1",
"distill"
] | 2025-04-18T03:13:58 | null | null |
67d0465e363941e3a07ed1bb | AnonRes/OpenMind | AnonRes | {"license": "cc-by-4.0", "task_categories": ["image-feature-extraction"], "pretty_name": "The OpenMind Dataset", "tags": ["3d", "image"]} | false | null | 2025-04-03T11:51:07 | 15 | 14 | false | 7a1d5ce1ff35de400b7f4c0dc957a69c5b581409 |
The OpenMind Dataset: A large-scale Head-And-Neck 3D MRI Dataset for self-supervised learning
Description
The OpenMind Dataset is a large-scale 3D MRI dataset of the head and neck region featuring 114k MRI Images. Its purpose is to provide access of large amounts of 3D medical imaging data to accelerate the development of self-supervised learning methods for 3D medical imaging. This data was pooled from exactly 800 datasets from the OpenNeuro platform and provides 23… See the full description on the dataset page: https://huggingface.co/datasets/AnonRes/OpenMind. | 1,501 | 1,929 | [
"task_categories:image-feature-extraction",
"license:cc-by-4.0",
"modality:3d",
"modality:image",
"region:us",
"3d",
"image"
] | 2025-03-11T14:19:10 | null | null |
68051956b83ee49250233b17 | marcodsn/academic-chains | marcodsn | {"tags": ["reasoning-datasets-competition", "reasoning", "academic-papers", "question-answering", "chain-of-thought", "biology", "economics"], "language": ["en"], "license": "apache-2.0", "pretty_name": "Academic Reasoning and Intuition Chains", "configs": [{"config_name": "default", "data_files": [{"split": "train", "path": "data/train.jsonl"}, {"split": "zraw", "path": "data/zraw.jsonl"}, {"split": "zraw_curator", "path": "data/zraw_curator.jsonl"}]}]} | false | null | 2025-04-23T19:59:13 | 14 | 14 | false | fffc0e1498e1f1181227ea6a5852ed47ec1384d3 |
Dataset Card for Academic Reasoning and Intuition Chains
This dataset contains reasoning (and intuition) chains distilled from open-access research papers, primarily focusing on the q-bio and econ.GN categories (check arXiv for more information about the categories). The goal is to create academically-grounded reasoning chains that capture the underlying logical structure, argumentation, or justification presented by the authors.
This dataset was created as a proof-of-concept for… See the full description on the dataset page: https://huggingface.co/datasets/marcodsn/academic-chains. | 141 | 141 | [
"language:en",
"license:apache-2.0",
"size_categories:1K<n<10K",
"format:json",
"modality:tabular",
"modality:text",
"library:datasets",
"library:pandas",
"library:mlcroissant",
"library:polars",
"region:us",
"reasoning-datasets-competition",
"reasoning",
"academic-papers",
"question-answering",
"chain-of-thought",
"biology",
"economics"
] | 2025-04-20T15:57:10 | null | null |
67f94a007b1c131bebc82d5c | wildflow/sweet-corals | wildflow | {"license": "cc-by-4.0", "tags": ["coral reef", "3d gaussian splatting", "photogrammetry", "3d", "wildflow", "orthomosaic"], "pretty_name": "3D Coral Reefs"} | false | null | 2025-04-21T13:20:46 | 14 | 13 | false | 237ca3ad4e52d3f0b21df49995beb5034cf579b8 |
Coral reefs 3D photogrammetry
Description
We 3D mapped multiple coral reefs in Indonesia (following this protocol) and sharing all our data with you 🤗
This dataset currently contains 90,289 (352GB) of raw GoPro images and some colour-corrected images.
Additional data - including camera poses, reconstructed 3D point clouds, 3D polygonal meshes, orthomosaics, annotations, and 3D Gaussian Splatting models - will be added soon. We just decided share raw data right now, and… See the full description on the dataset page: https://huggingface.co/datasets/wildflow/sweet-corals. | 3,451 | 3,454 | [
"license:cc-by-4.0",
"size_categories:1K<n<10K",
"format:imagefolder",
"modality:image",
"modality:3d",
"library:datasets",
"library:mlcroissant",
"doi:10.57967/hf/5162",
"region:us",
"coral reef",
"3d gaussian splatting",
"photogrammetry",
"3d",
"wildflow",
"orthomosaic"
] | 2025-04-11T16:57:36 | null | null |
680636da910fa3a21b4acd1e | newsletter/HiDream-I1-Artists | newsletter | null | false | null | 2025-04-21T12:32:46 | 13 | 13 | false | a5a3958eedaf092219d6eb9ca9c9fe8a43b534d4 | null | 3,116 | 3,116 | [
"size_categories:1K<n<10K",
"format:imagefolder",
"modality:image",
"library:datasets",
"library:mlcroissant",
"region:us"
] | 2025-04-21T12:15:22 | null | null |
672d8bf4bde669ec7e63ba72 | allenai/tulu-3-sft-mixture | allenai | {"annotations_creators": ["crowdsourced", "expert-generated", "machine-generated"], "language": ["amh", "arb", "ary", "ars", "acq", "arz", "apc", "ben", "ceb", "dan", "deu", "ell", "eng", "eus", "fil", "fin", "fra", "gle", "guj", "hat", "hau", "hin", "hun", "ibo", "ind", "ita", "jav", "jpn", "kan", "kir", "kor", "kur", "lit", "mal", "mar", "mlg", "msa", "mya", "nep", "nld", "nso", "nya", "pan", "pes", "pol", "por", "pus", "rus", "sin", "sna", "snd", "som", "spa", "sqi", "srp", "sun", "swa", "swe", "tam", "tel", "tha", "tur", "ukr", "urd", "vie", "wol", "xho", "yor", "zho", "zul"], "license": "odc-by", "multilinguality": ["multilingual"], "size_categories": ["100K<n<1M"], "source_datasets": ["allenai/coconot", "ai2-adapt-dev/flan_v2_converted", "HuggingFaceH4/no_robots", "OpenAssistant/oasst1", "allenai/tulu-3-personas-math", "allenai/tulu-3-sft-personas-math-grade", "allenai/tulu-3-sft-personas-code", "allenai/tulu-3-personas-algebra", "allenai/tulu-3-sft-personas-instruction-following", "AI-MO/NuminaMath-TIR", "allenai/wildguardmix", "allenai/wildjailbreak", "allenai/tulu-3-hard-coded", "CohereForAI/aya_dataset", "allenai/WildChat-1M", "LipengCS/Table-GPT", "allenai/SciRIFF", "theblackcat102/evol-codealpaca-v1"], "task_categories": ["other"], "dataset_info": {"features": [{"name": "id", "dtype": "string"}, {"name": "messages", "list": [{"name": "content", "dtype": "string"}, {"name": "role", "dtype": "string"}]}, {"name": "source", "dtype": "string"}], "splits": [{"name": "train", "num_bytes": 2914250826.5647593, "num_examples": 939343}], "download_size": 1412954868, "dataset_size": 2914250826.5647593}, "configs": [{"config_name": "default", "data_files": [{"split": "train", "path": "data/train-*"}]}]} | false | null | 2024-12-02T19:48:33 | 137 | 12 | false | b14afda60f1bbebe55d5d2fa1e4df5042f97f8be |
Tulu 3 SFT Mixture
Note that this collection is licensed under ODC-BY-1.0 license; different licenses apply to subsets of the data. Some portions of the dataset are non-commercial. We present the mixture as a research artifact.
The Tulu 3 SFT mixture was used to train the Tulu 3 series of models.
It contains 939,344 samples from the following sets:
CoCoNot (ODC-BY-1.0), 10,983 prompts (Brahman et al., 2024)
FLAN v2 via ai2-adapt-dev/flan_v2_converted, 89,982 prompts (Longpre… See the full description on the dataset page: https://huggingface.co/datasets/allenai/tulu-3-sft-mixture. | 4,985 | 26,506 | [
"task_categories:other",
"annotations_creators:crowdsourced",
"annotations_creators:expert-generated",
"annotations_creators:machine-generated",
"multilinguality:multilingual",
"source_datasets:allenai/coconot",
"source_datasets:ai2-adapt-dev/flan_v2_converted",
"source_datasets:HuggingFaceH4/no_robots",
"source_datasets:OpenAssistant/oasst1",
"source_datasets:allenai/tulu-3-personas-math",
"source_datasets:allenai/tulu-3-sft-personas-math-grade",
"source_datasets:allenai/tulu-3-sft-personas-code",
"source_datasets:allenai/tulu-3-personas-algebra",
"source_datasets:allenai/tulu-3-sft-personas-instruction-following",
"source_datasets:AI-MO/NuminaMath-TIR",
"source_datasets:allenai/wildguardmix",
"source_datasets:allenai/wildjailbreak",
"source_datasets:allenai/tulu-3-hard-coded",
"source_datasets:CohereForAI/aya_dataset",
"source_datasets:allenai/WildChat-1M",
"source_datasets:LipengCS/Table-GPT",
"source_datasets:allenai/SciRIFF",
"source_datasets:theblackcat102/evol-codealpaca-v1",
"language:amh",
"language:arb",
"language:ary",
"language:ars",
"language:acq",
"language:arz",
"language:apc",
"language:ben",
"language:ceb",
"language:dan",
"language:deu",
"language:ell",
"language:eng",
"language:eus",
"language:fil",
"language:fin",
"language:fra",
"language:gle",
"language:guj",
"language:hat",
"language:hau",
"language:hin",
"language:hun",
"language:ibo",
"language:ind",
"language:ita",
"language:jav",
"language:jpn",
"language:kan",
"language:kir",
"language:kor",
"language:kur",
"language:lit",
"language:mal",
"language:mar",
"language:mlg",
"language:msa",
"language:mya",
"language:nep",
"language:nld",
"language:nso",
"language:nya",
"language:pan",
"language:pes",
"language:pol",
"language:por",
"language:pus",
"language:rus",
"language:sin",
"language:sna",
"language:snd",
"language:som",
"language:spa",
"language:sqi",
"language:srp",
"language:sun",
"language:swa",
"language:swe",
"language:tam",
"language:tel",
"language:tha",
"language:tur",
"language:ukr",
"language:urd",
"language:vie",
"language:wol",
"language:xho",
"language:yor",
"language:zho",
"language:zul",
"license:odc-by",
"size_categories:100K<n<1M",
"format:parquet",
"modality:text",
"library:datasets",
"library:dask",
"library:mlcroissant",
"library:polars",
"region:us"
] | 2024-11-08T03:56:36 | null | null |
676f70846bf205795346d2be | FreedomIntelligence/medical-o1-reasoning-SFT | FreedomIntelligence | {"license": "apache-2.0", "task_categories": ["question-answering", "text-generation"], "language": ["en", "zh"], "tags": ["medical", "biology"], "configs": [{"config_name": "en", "data_files": "medical_o1_sft.json"}, {"config_name": "zh", "data_files": "medical_o1_sft_Chinese.json"}, {"config_name": "en_mix", "data_files": "medical_o1_sft_mix.json"}, {"config_name": "zh_mix", "data_files": "medical_o1_sft_mix_Chinese.json"}]} | false | null | 2025-04-22T15:11:21 | 655 | 12 | false | fc2c9e8a37b38f38da6d449564a8c350b244aef4 |
News
[2025/04/22] We split the data and kept only the medical SFT dataset (medical_o1_sft.json). The file medical_o1_sft_mix.json contains a mix of medical and general instruction data.
[2025/02/22] We released the distilled dataset from Deepseek-R1 based on medical verifiable problems. You can use it to initialize your models with the reasoning chain from Deepseek-R1.
[2024/12/25] We open-sourced the medical reasoning dataset for SFT, built on medical verifiable problems and an LLM… See the full description on the dataset page: https://huggingface.co/datasets/FreedomIntelligence/medical-o1-reasoning-SFT. | 15,125 | 59,462 | [
"task_categories:question-answering",
"task_categories:text-generation",
"language:en",
"language:zh",
"license:apache-2.0",
"size_categories:10K<n<100K",
"format:json",
"modality:text",
"library:datasets",
"library:pandas",
"library:mlcroissant",
"library:polars",
"arxiv:2412.18925",
"region:us",
"medical",
"biology"
] | 2024-12-28T03:29:08 | null | null |
67f62a9296e24db82ed27e76 | divaroffical/real_estate_ads | divaroffical | {"license": "odbl"} | false | null | 2025-04-16T08:31:19 | 60 | 12 | false | bdc2a655ad7955e1cfcf6a7467de85144d5d9099 |
🏠 Divar Real Estate Ads Dataset
📋 Overview
The real_estate_ads dataset contains one million anonymized real estate advertisements collected from the Divar platform, one of the largest classified ads platforms in the Middle East. This comprehensive dataset provides researchers, data scientists, and entrepreneurs with authentic real estate market data to build innovative solutions such as price evaluation models, market analysis tools, and forecasting systems.… See the full description on the dataset page: https://huggingface.co/datasets/divaroffical/real_estate_ads. | 1,725 | 1,725 | [
"license:odbl",
"size_categories:1M<n<10M",
"format:csv",
"modality:tabular",
"modality:text",
"library:datasets",
"library:pandas",
"library:mlcroissant",
"library:polars",
"region:us"
] | 2025-04-09T08:06:42 | null | null |
651fbfa3be34a5f2cf6871d1 | a686d380/h-corpus-2023 | a686d380 | {"viewer": false, "language": ["zh"]} | false | null | 2023-10-06T08:38:36 | 174 | 11 | false | 770d79e988706a68df8e2bc9dc37348e109ded59 | 经过清洗和去重过的H小说
共205,028篇文章,解压后17.0 GB
仅用于科学研究!
| 596 | 3,053 | [
"language:zh",
"region:us"
] | 2023-10-06T08:04:51 | null | null |
67e72b7a8743733af57793b1 | facebook/PLM-Video-Human | facebook | {"annotations_creators": ["other"], "language_creators": ["other"], "language": ["en"], "task_categories": ["multiple-choice", "visual-question-answering"], "pretty_name": "plm_video_human", "dataset_info": [{"config_name": "fgqa", "features": [{"name": "qa_id", "dtype": "string"}, {"name": "segment_id", "dtype": "string"}, {"name": "question", "dtype": "string"}, {"name": "answer", "dtype": "string"}, {"name": "metadata", "struct": [{"name": "source_video_id", "dtype": "string"}, {"name": "source_dataset", "dtype": "string"}, {"name": "source_start_time", "dtype": "float"}, {"name": "source_end_time", "dtype": "float"}, {"name": "what_description", "dtype": "string"}, {"name": "q_type", "dtype": "string"}, {"name": "q_subtype", "dtype": "string"}, {"name": "domain", "dtype": "string"}, {"name": "is_audited", "dtype": "int32"}]}], "splits": [{"name": "train", "num_bytes": 409709782, "num_examples": 2321035}]}, {"config_name": "rcap", "features": [{"name": "uid", "dtype": "int32"}, {"name": "video", "dtype": "string"}, {"name": "masklet_id", "dtype": "int32"}, {"name": "total_frames", "dtype": "int32"}, {"name": "caption", "dtype": "string"}, {"name": "start_frame", "dtype": "int32"}, {"name": "end_frame", "dtype": "int32"}], "splits": [{"name": "train", "num_bytes": 13738246, "num_examples": 179447}]}, {"config_name": "rdcap", "features": [{"name": "uid", "dtype": "int32"}, {"name": "video", "dtype": "string"}, {"name": "masklet_id", "dtype": "int32"}, {"name": "total_frames", "dtype": "int32"}, {"name": "dense_captions", "list": [{"name": "start_frame", "dtype": "int32"}, {"name": "end_frame", "dtype": "int32"}, {"name": "caption", "dtype": "string"}]}], "splits": [{"name": "train", "num_bytes": 14268327, "num_examples": 117248}]}, {"config_name": "rtloc", "features": [{"name": "uid", "dtype": "int32"}, {"name": "video", "dtype": "string"}, {"name": "masklet_id", "dtype": "int32"}, {"name": "total_frames", "dtype": "int32"}, {"name": "caption", "dtype": "string"}, {"name": "start_frame", "dtype": "int32"}, {"name": "end_frame", "dtype": "int32"}], "splits": [{"name": "train", "num_bytes": 13739069, "num_examples": 179447}]}], "configs": [{"config_name": "fgqa", "data_files": [{"split": "train", "path": "fgqa/plm_fgqa_train.parquet"}]}, {"config_name": "rcap", "data_files": [{"split": "train", "path": "rcap/plm_rcap_train.parquet"}]}, {"config_name": "rdcap", "data_files": [{"split": "train", "path": "rdcap/plm_rdcap_train.parquet"}]}, {"config_name": "rtloc", "data_files": [{"split": "train", "path": "rtloc/plm_rtloc_train.parquet"}]}], "license": "cc-by-4.0"} | false | null | 2025-04-18T21:50:35 | 11 | 11 | false | b79420a848932e314e096d9607f08d066f9b838d |
Dataset Card for PLM-Video Human
PLM-Video-Human is a collection of human-annotated resources for training Vision Language Models,
focused on detailed video understanding. Training tasks include: fine-grained open-ended question answering (FGQA), Region-based Video Captioning (RCap),
Region-based Dense Video Captioning (RDCap) and Region-based Temporal Localization (RTLoc).
[📃 Tech Report]
[📂 Github]
Dataset Structure
Fine-Grained Question Answering… See the full description on the dataset page: https://huggingface.co/datasets/facebook/PLM-Video-Human. | 1,084 | 1,084 | [
"task_categories:multiple-choice",
"task_categories:visual-question-answering",
"annotations_creators:other",
"language_creators:other",
"language:en",
"license:cc-by-4.0",
"size_categories:1M<n<10M",
"format:parquet",
"modality:tabular",
"modality:text",
"library:datasets",
"library:pandas",
"library:mlcroissant",
"library:polars",
"arxiv:2504.13180",
"region:us"
] | 2025-03-28T23:06:34 | null | null |
68072cc4cce05035af98207e | nvidia/OpenMathReasoning | nvidia | {"language": ["en"], "license": "cc-by-4.0", "size_categories": ["1M<n<10M"], "task_categories": ["question-answering", "text-generation"], "pretty_name": "OpenMathReasoning", "tags": ["math", "nvidia"], "configs": [{"config_name": "default", "data_files": [{"split": "cot", "path": "data/cot-*"}, {"split": "tir", "path": "data/tir-*"}, {"split": "genselect", "path": "data/genselect-*"}]}], "dataset_info": {"features": [{"name": "expected_answer", "dtype": "string"}, {"name": "problem_type", "dtype": "string"}, {"name": "problem_source", "dtype": "string"}, {"name": "generation_model", "dtype": "string"}, {"name": "pass_rate_72b_tir", "dtype": "string"}, {"name": "problem", "dtype": "string"}, {"name": "generated_solution", "dtype": "string"}, {"name": "inference_mode", "dtype": "string"}], "splits": [{"name": "cot", "num_bytes": 71638774515, "num_examples": 3201061}, {"name": "tir", "num_bytes": 35467270369, "num_examples": 1703010}, {"name": "genselect", "num_bytes": 6981053721, "num_examples": 565620}], "download_size": 49370957110, "dataset_size": 114087098605}} | false | null | 2025-04-23T19:57:24 | 11 | 11 | false | 26678c76c71d0d584b184edc5226e22adbd95ff6 |
OpenMathReasoning
OpenMathReasoning is a large-scale math reasoning dataset for training large language models (LLMs).
This dataset contains
540K unique mathematical problems sourced from AoPS forums,
3.2M long chain-of-thought (CoT) solutions
1.7M long tool-integrated reasoning (TIR) solutions
566K samples that select the most promising solution out of many candidates (GenSelect)
We used Qwen2.5-32B-Instruct to preprocess problems, and
DeepSeek-R1 and QwQ-32B to generate… See the full description on the dataset page: https://huggingface.co/datasets/nvidia/OpenMathReasoning. | 18 | 18 | [
"task_categories:question-answering",
"task_categories:text-generation",
"language:en",
"license:cc-by-4.0",
"size_categories:1M<n<10M",
"format:parquet",
"modality:text",
"library:datasets",
"library:dask",
"library:mlcroissant",
"library:polars",
"region:us",
"math",
"nvidia"
] | 2025-04-22T05:44:36 | null | null |
67b32145bac2756ce9a4a0fe | Congliu/Chinese-DeepSeek-R1-Distill-data-110k | Congliu | {"license": "apache-2.0", "language": ["zh"], "size_categories": ["100K<n<1M"], "task_categories": ["text-generation", "text2text-generation", "question-answering"]} | false | null | 2025-02-21T02:18:08 | 637 | 10 | false | 8520b649430617c2be4490f424d251d09d835ed3 |
中文基于满血DeepSeek-R1蒸馏数据集(Chinese-Data-Distill-From-R1)
🤗 Hugging Face | 🤖 ModelScope | 🚀 Github | 📑 Blog
注意:提供了直接SFT使用的版本,点击下载。将数据中的思考和答案整合成output字段,大部分SFT代码框架均可直接直接加载训练。
本数据集为中文开源蒸馏满血R1的数据集,数据集中不仅包含math数据,还包括大量的通用类型数据,总数量为110K。
为什么开源这个数据?
R1的效果十分强大,并且基于R1蒸馏数据SFT的小模型也展现出了强大的效果,但检索发现,大部分开源的R1蒸馏数据集均为英文数据集。 同时,R1的报告中展示,蒸馏模型中同时也使用了部分通用场景数据集。
为了帮助大家更好地复现R1蒸馏模型的效果,特此开源中文数据集。该中文数据集中的数据分布如下:
Math:共计36568个样本,
Exam:共计2432个样本,
STEM:共计12648个样本,… See the full description on the dataset page: https://huggingface.co/datasets/Congliu/Chinese-DeepSeek-R1-Distill-data-110k. | 3,012 | 12,666 | [
"task_categories:text-generation",
"task_categories:text2text-generation",
"task_categories:question-answering",
"language:zh",
"license:apache-2.0",
"size_categories:100K<n<1M",
"format:json",
"modality:tabular",
"modality:text",
"library:datasets",
"library:pandas",
"library:mlcroissant",
"library:polars",
"region:us"
] | 2025-02-17T11:45:09 | null | null |
67c0cda5c0b7a236a5f070e3 | glaiveai/reasoning-v1-20m | glaiveai | {"dataset_info": {"features": [{"name": "prompt", "dtype": "string"}, {"name": "response", "dtype": "string"}], "splits": [{"name": "train", "num_bytes": 177249016911, "num_examples": 22199375}], "download_size": 87247205094, "dataset_size": 177249016911}, "configs": [{"config_name": "default", "data_files": [{"split": "train", "path": "data/train-*"}]}], "license": "apache-2.0", "task_categories": ["text-generation"], "language": ["en"], "size_categories": ["10M<n<100M"]} | false | null | 2025-03-19T13:21:37 | 202 | 10 | false | da6bb3d0ff8fd8ea5abacee8519762ca6aaf367e |
We are excited to release a synthetic reasoning dataset containing 22mil+ general reasoning questions and responses generated using deepseek-ai/DeepSeek-R1-Distill-Llama-70B. While there have been multiple efforts to build open reasoning datasets for math and code tasks, we noticed a lack of large datasets containing reasoning traces for diverse non code/math topics like social and natural sciences, education, creative writing and general conversations, which is why we decided to release this… See the full description on the dataset page: https://huggingface.co/datasets/glaiveai/reasoning-v1-20m. | 11,852 | 14,949 | [
"task_categories:text-generation",
"language:en",
"license:apache-2.0",
"size_categories:10M<n<100M",
"format:parquet",
"modality:text",
"library:datasets",
"library:dask",
"library:mlcroissant",
"library:polars",
"region:us"
] | 2025-02-27T20:40:05 | null | null |
66212f29fb07c3e05ad0432e | HuggingFaceFW/fineweb | HuggingFaceFW | {"license": "odc-by", "task_categories": ["text-generation"], "language": ["en"], "pretty_name": "FineWeb", "size_categories": ["n>1T"], "configs": [{"config_name": "default", "data_files": [{"split": "train", "path": "data/*/*"}]}, {"config_name": "sample-10BT", "data_files": [{"split": "train", "path": "sample/10BT/*"}]}, {"config_name": "sample-100BT", "data_files": [{"split": "train", "path": "sample/100BT/*"}]}, {"config_name": "sample-350BT", "data_files": [{"split": "train", "path": "sample/350BT/*"}]}, {"config_name": "CC-MAIN-2024-51", "data_files": [{"split": "train", "path": "data/CC-MAIN-2024-51/*"}]}, {"config_name": "CC-MAIN-2024-46", "data_files": [{"split": "train", "path": "data/CC-MAIN-2024-46/*"}]}, {"config_name": "CC-MAIN-2024-42", "data_files": [{"split": "train", "path": "data/CC-MAIN-2024-42/*"}]}, {"config_name": "CC-MAIN-2024-38", "data_files": [{"split": "train", "path": "data/CC-MAIN-2024-38/*"}]}, {"config_name": "CC-MAIN-2024-33", "data_files": [{"split": "train", "path": "data/CC-MAIN-2024-33/*"}]}, {"config_name": "CC-MAIN-2024-30", "data_files": [{"split": "train", "path": "data/CC-MAIN-2024-30/*"}]}, {"config_name": "CC-MAIN-2024-26", "data_files": [{"split": "train", "path": "data/CC-MAIN-2024-26/*"}]}, {"config_name": "CC-MAIN-2024-22", "data_files": [{"split": "train", "path": "data/CC-MAIN-2024-22/*"}]}, {"config_name": "CC-MAIN-2024-18", "data_files": [{"split": "train", "path": "data/CC-MAIN-2024-18/*"}]}, {"config_name": "CC-MAIN-2024-10", "data_files": [{"split": "train", "path": "data/CC-MAIN-2024-10/*"}]}, {"config_name": "CC-MAIN-2023-50", "data_files": [{"split": "train", "path": "data/CC-MAIN-2023-50/*"}]}, {"config_name": "CC-MAIN-2023-40", "data_files": [{"split": "train", "path": "data/CC-MAIN-2023-40/*"}]}, {"config_name": "CC-MAIN-2023-23", "data_files": [{"split": "train", "path": "data/CC-MAIN-2023-23/*"}]}, {"config_name": "CC-MAIN-2023-14", "data_files": [{"split": "train", "path": "data/CC-MAIN-2023-14/*"}]}, {"config_name": "CC-MAIN-2023-06", "data_files": [{"split": "train", "path": "data/CC-MAIN-2023-06/*"}]}, {"config_name": "CC-MAIN-2022-49", "data_files": [{"split": "train", "path": "data/CC-MAIN-2022-49/*"}]}, {"config_name": "CC-MAIN-2022-40", "data_files": [{"split": "train", "path": "data/CC-MAIN-2022-40/*"}]}, {"config_name": "CC-MAIN-2022-33", "data_files": [{"split": "train", "path": "data/CC-MAIN-2022-33/*"}]}, {"config_name": "CC-MAIN-2022-27", "data_files": [{"split": "train", "path": "data/CC-MAIN-2022-27/*"}]}, {"config_name": "CC-MAIN-2022-21", "data_files": [{"split": "train", "path": "data/CC-MAIN-2022-21/*"}]}, {"config_name": "CC-MAIN-2022-05", "data_files": [{"split": "train", "path": "data/CC-MAIN-2022-05/*"}]}, {"config_name": "CC-MAIN-2021-49", "data_files": [{"split": "train", "path": "data/CC-MAIN-2021-49/*"}]}, {"config_name": "CC-MAIN-2021-43", "data_files": [{"split": "train", "path": "data/CC-MAIN-2021-43/*"}]}, {"config_name": "CC-MAIN-2021-39", "data_files": [{"split": "train", "path": "data/CC-MAIN-2021-39/*"}]}, {"config_name": "CC-MAIN-2021-31", "data_files": [{"split": "train", "path": "data/CC-MAIN-2021-31/*"}]}, {"config_name": "CC-MAIN-2021-25", "data_files": [{"split": "train", "path": "data/CC-MAIN-2021-25/*"}]}, {"config_name": "CC-MAIN-2021-21", "data_files": [{"split": "train", "path": "data/CC-MAIN-2021-21/*"}]}, {"config_name": "CC-MAIN-2021-17", "data_files": [{"split": "train", "path": "data/CC-MAIN-2021-17/*"}]}, {"config_name": "CC-MAIN-2021-10", "data_files": [{"split": "train", "path": "data/CC-MAIN-2021-10/*"}]}, {"config_name": "CC-MAIN-2021-04", "data_files": [{"split": "train", "path": "data/CC-MAIN-2021-04/*"}]}, {"config_name": "CC-MAIN-2020-50", "data_files": [{"split": "train", "path": "data/CC-MAIN-2020-50/*"}]}, {"config_name": "CC-MAIN-2020-45", "data_files": [{"split": "train", "path": "data/CC-MAIN-2020-45/*"}]}, {"config_name": "CC-MAIN-2020-40", "data_files": [{"split": "train", "path": "data/CC-MAIN-2020-40/*"}]}, {"config_name": "CC-MAIN-2020-34", "data_files": [{"split": "train", "path": "data/CC-MAIN-2020-34/*"}]}, {"config_name": "CC-MAIN-2020-29", "data_files": [{"split": "train", "path": "data/CC-MAIN-2020-29/*"}]}, {"config_name": "CC-MAIN-2020-24", "data_files": [{"split": "train", "path": "data/CC-MAIN-2020-24/*"}]}, {"config_name": "CC-MAIN-2020-16", "data_files": [{"split": "train", "path": "data/CC-MAIN-2020-16/*"}]}, {"config_name": "CC-MAIN-2020-10", "data_files": [{"split": "train", "path": "data/CC-MAIN-2020-10/*"}]}, {"config_name": "CC-MAIN-2020-05", "data_files": [{"split": "train", "path": "data/CC-MAIN-2020-05/*"}]}, {"config_name": "CC-MAIN-2019-51", "data_files": [{"split": "train", "path": "data/CC-MAIN-2019-51/*"}]}, {"config_name": "CC-MAIN-2019-47", "data_files": [{"split": "train", "path": "data/CC-MAIN-2019-47/*"}]}, {"config_name": "CC-MAIN-2019-43", "data_files": [{"split": "train", "path": "data/CC-MAIN-2019-43/*"}]}, {"config_name": "CC-MAIN-2019-39", "data_files": [{"split": "train", "path": "data/CC-MAIN-2019-39/*"}]}, {"config_name": "CC-MAIN-2019-35", "data_files": [{"split": "train", "path": "data/CC-MAIN-2019-35/*"}]}, {"config_name": "CC-MAIN-2019-30", "data_files": [{"split": "train", "path": "data/CC-MAIN-2019-30/*"}]}, {"config_name": "CC-MAIN-2019-26", "data_files": [{"split": "train", "path": "data/CC-MAIN-2019-26/*"}]}, {"config_name": "CC-MAIN-2019-22", "data_files": [{"split": "train", "path": "data/CC-MAIN-2019-22/*"}]}, {"config_name": "CC-MAIN-2019-18", "data_files": [{"split": "train", "path": "data/CC-MAIN-2019-18/*"}]}, {"config_name": "CC-MAIN-2019-13", "data_files": [{"split": "train", "path": "data/CC-MAIN-2019-13/*"}]}, {"config_name": "CC-MAIN-2019-09", "data_files": [{"split": "train", "path": "data/CC-MAIN-2019-09/*"}]}, {"config_name": "CC-MAIN-2019-04", "data_files": [{"split": "train", "path": "data/CC-MAIN-2019-04/*"}]}, {"config_name": "CC-MAIN-2018-51", "data_files": [{"split": "train", "path": "data/CC-MAIN-2018-51/*"}]}, {"config_name": "CC-MAIN-2018-47", "data_files": [{"split": "train", "path": "data/CC-MAIN-2018-47/*"}]}, {"config_name": "CC-MAIN-2018-43", "data_files": [{"split": "train", "path": "data/CC-MAIN-2018-43/*"}]}, {"config_name": "CC-MAIN-2018-39", "data_files": [{"split": "train", "path": "data/CC-MAIN-2018-39/*"}]}, {"config_name": "CC-MAIN-2018-34", "data_files": [{"split": "train", "path": "data/CC-MAIN-2018-34/*"}]}, {"config_name": "CC-MAIN-2018-30", "data_files": [{"split": "train", "path": "data/CC-MAIN-2018-30/*"}]}, {"config_name": "CC-MAIN-2018-26", "data_files": [{"split": "train", "path": "data/CC-MAIN-2018-26/*"}]}, {"config_name": "CC-MAIN-2018-22", "data_files": [{"split": "train", "path": "data/CC-MAIN-2018-22/*"}]}, {"config_name": "CC-MAIN-2018-17", "data_files": [{"split": "train", "path": "data/CC-MAIN-2018-17/*"}]}, {"config_name": "CC-MAIN-2018-13", "data_files": [{"split": "train", "path": "data/CC-MAIN-2018-13/*"}]}, {"config_name": "CC-MAIN-2018-09", "data_files": [{"split": "train", "path": "data/CC-MAIN-2018-09/*"}]}, {"config_name": "CC-MAIN-2018-05", "data_files": [{"split": "train", "path": "data/CC-MAIN-2018-05/*"}]}, {"config_name": "CC-MAIN-2017-51", "data_files": [{"split": "train", "path": "data/CC-MAIN-2017-51/*"}]}, {"config_name": "CC-MAIN-2017-47", "data_files": [{"split": "train", "path": "data/CC-MAIN-2017-47/*"}]}, {"config_name": "CC-MAIN-2017-43", "data_files": [{"split": "train", "path": "data/CC-MAIN-2017-43/*"}]}, {"config_name": "CC-MAIN-2017-39", "data_files": [{"split": "train", "path": "data/CC-MAIN-2017-39/*"}]}, {"config_name": "CC-MAIN-2017-34", "data_files": [{"split": "train", "path": "data/CC-MAIN-2017-34/*"}]}, {"config_name": "CC-MAIN-2017-30", "data_files": [{"split": "train", "path": "data/CC-MAIN-2017-30/*"}]}, {"config_name": "CC-MAIN-2017-26", "data_files": [{"split": "train", "path": "data/CC-MAIN-2017-26/*"}]}, {"config_name": "CC-MAIN-2017-22", "data_files": [{"split": "train", "path": "data/CC-MAIN-2017-22/*"}]}, {"config_name": "CC-MAIN-2017-17", "data_files": [{"split": "train", "path": "data/CC-MAIN-2017-17/*"}]}, {"config_name": "CC-MAIN-2017-13", "data_files": [{"split": "train", "path": "data/CC-MAIN-2017-13/*"}]}, {"config_name": "CC-MAIN-2017-09", "data_files": [{"split": "train", "path": "data/CC-MAIN-2017-09/*"}]}, {"config_name": "CC-MAIN-2017-04", "data_files": [{"split": "train", "path": "data/CC-MAIN-2017-04/*"}]}, {"config_name": "CC-MAIN-2016-50", "data_files": [{"split": "train", "path": "data/CC-MAIN-2016-50/*"}]}, {"config_name": "CC-MAIN-2016-44", "data_files": [{"split": "train", "path": "data/CC-MAIN-2016-44/*"}]}, {"config_name": "CC-MAIN-2016-40", "data_files": [{"split": "train", "path": "data/CC-MAIN-2016-40/*"}]}, {"config_name": "CC-MAIN-2016-36", "data_files": [{"split": "train", "path": "data/CC-MAIN-2016-36/*"}]}, {"config_name": "CC-MAIN-2016-30", "data_files": [{"split": "train", "path": "data/CC-MAIN-2016-30/*"}]}, {"config_name": "CC-MAIN-2016-26", "data_files": [{"split": "train", "path": "data/CC-MAIN-2016-26/*"}]}, {"config_name": "CC-MAIN-2016-22", "data_files": [{"split": "train", "path": "data/CC-MAIN-2016-22/*"}]}, {"config_name": "CC-MAIN-2016-18", "data_files": [{"split": "train", "path": "data/CC-MAIN-2016-18/*"}]}, {"config_name": "CC-MAIN-2016-07", "data_files": [{"split": "train", "path": "data/CC-MAIN-2016-07/*"}]}, {"config_name": "CC-MAIN-2015-48", "data_files": [{"split": "train", "path": "data/CC-MAIN-2015-48/*"}]}, {"config_name": "CC-MAIN-2015-40", "data_files": [{"split": "train", "path": "data/CC-MAIN-2015-40/*"}]}, {"config_name": "CC-MAIN-2015-35", "data_files": [{"split": "train", "path": "data/CC-MAIN-2015-35/*"}]}, {"config_name": "CC-MAIN-2015-32", "data_files": [{"split": "train", "path": "data/CC-MAIN-2015-32/*"}]}, {"config_name": "CC-MAIN-2015-27", "data_files": [{"split": "train", "path": "data/CC-MAIN-2015-27/*"}]}, {"config_name": "CC-MAIN-2015-22", "data_files": [{"split": "train", "path": "data/CC-MAIN-2015-22/*"}]}, {"config_name": "CC-MAIN-2015-18", "data_files": [{"split": "train", "path": "data/CC-MAIN-2015-18/*"}]}, {"config_name": "CC-MAIN-2015-14", "data_files": [{"split": "train", "path": "data/CC-MAIN-2015-14/*"}]}, {"config_name": "CC-MAIN-2015-11", "data_files": [{"split": "train", "path": "data/CC-MAIN-2015-11/*"}]}, {"config_name": "CC-MAIN-2015-06", "data_files": [{"split": "train", "path": "data/CC-MAIN-2015-06/*"}]}, {"config_name": "CC-MAIN-2014-52", "data_files": [{"split": "train", "path": "data/CC-MAIN-2014-52/*"}]}, {"config_name": "CC-MAIN-2014-49", "data_files": [{"split": "train", "path": "data/CC-MAIN-2014-49/*"}]}, {"config_name": "CC-MAIN-2014-42", "data_files": [{"split": "train", "path": "data/CC-MAIN-2014-42/*"}]}, {"config_name": "CC-MAIN-2014-41", "data_files": [{"split": "train", "path": "data/CC-MAIN-2014-41/*"}]}, {"config_name": "CC-MAIN-2014-35", "data_files": [{"split": "train", "path": "data/CC-MAIN-2014-35/*"}]}, {"config_name": "CC-MAIN-2014-23", "data_files": [{"split": "train", "path": "data/CC-MAIN-2014-23/*"}]}, {"config_name": "CC-MAIN-2014-15", "data_files": [{"split": "train", "path": "data/CC-MAIN-2014-15/*"}]}, {"config_name": "CC-MAIN-2014-10", "data_files": [{"split": "train", "path": "data/CC-MAIN-2014-10/*"}]}, {"config_name": "CC-MAIN-2013-48", "data_files": [{"split": "train", "path": "data/CC-MAIN-2013-48/*"}]}, {"config_name": "CC-MAIN-2013-20", "data_files": [{"split": "train", "path": "data/CC-MAIN-2013-20/*"}]}]} | false | null | 2025-01-31T14:10:44 | 2,118 | 9 | false | 0f039043b23fe1d4eed300b504aa4b4a68f1c7ba |
🍷 FineWeb
15 trillion tokens of the finest data the 🌐 web has to offer
What is it?
The 🍷 FineWeb dataset consists of more than 15T tokens of cleaned and deduplicated english web data from CommonCrawl. The data processing pipeline is optimized for LLM performance and ran on the 🏭 datatrove library, our large scale data processing library.
🍷 FineWeb was originally meant to be a fully open replication of 🦅 RefinedWeb, with a release of the full dataset under… See the full description on the dataset page: https://huggingface.co/datasets/HuggingFaceFW/fineweb. | 838,782 | 3,130,454 | [
"task_categories:text-generation",
"language:en",
"license:odc-by",
"size_categories:10B<n<100B",
"format:parquet",
"modality:tabular",
"modality:text",
"library:datasets",
"library:dask",
"library:mlcroissant",
"library:polars",
"arxiv:2306.01116",
"arxiv:2109.07445",
"arxiv:2406.17557",
"doi:10.57967/hf/2493",
"region:us"
] | 2024-04-18T14:33:13 | null | null |
67b20fc10861cec33b3afb8a | Conard/fortune-telling | Conard | {"license": "mit"} | false | null | 2025-02-17T05:13:43 | 131 | 9 | false | 6261fe0d35a75997972bbfcd9828020e340303fb | null | 3,969 | 9,477 | [
"license:mit",
"size_categories:n<1K",
"format:json",
"modality:text",
"library:datasets",
"library:pandas",
"library:mlcroissant",
"library:polars",
"region:us"
] | 2025-02-16T16:18:09 | null | null |
67c03fd6b9fe27a2ac49784d | open-r1/codeforces-cots | open-r1 | {"dataset_info": [{"config_name": "checker_interactor", "features": [{"name": "id", "dtype": "string"}, {"name": "aliases", "sequence": "string"}, {"name": "contest_id", "dtype": "string"}, {"name": "contest_name", "dtype": "string"}, {"name": "contest_type", "dtype": "string"}, {"name": "contest_start", "dtype": "int64"}, {"name": "contest_start_year", "dtype": "int64"}, {"name": "index", "dtype": "string"}, {"name": "time_limit", "dtype": "float64"}, {"name": "memory_limit", "dtype": "float64"}, {"name": "title", "dtype": "string"}, {"name": "description", "dtype": "string"}, {"name": "input_format", "dtype": "string"}, {"name": "output_format", "dtype": "string"}, {"name": "interaction_format", "dtype": "string"}, {"name": "note", "dtype": "string"}, {"name": "examples", "list": [{"name": "input", "dtype": "string"}, {"name": "output", "dtype": "string"}]}, {"name": "editorial", "dtype": "string"}, {"name": "prompt", "dtype": "string"}, {"name": "generation", "dtype": "string"}, {"name": "finish_reason", "dtype": "string"}, {"name": "api_metadata", "struct": [{"name": "completion_tokens", "dtype": "int64"}, {"name": "prompt_tokens", "dtype": "int64"}, {"name": "prompt_tokens_details", "dtype": "null"}, {"name": "total_tokens", "dtype": "int64"}]}, {"name": "messages", "list": [{"name": "content", "dtype": "string"}, {"name": "role", "dtype": "string"}]}], "splits": [{"name": "train", "num_bytes": 994149425, "num_examples": 35718}], "download_size": 274975300, "dataset_size": 994149425}, {"config_name": "solutions", "features": [{"name": "id", "dtype": "string"}, {"name": "aliases", "sequence": "string"}, {"name": "contest_id", "dtype": "string"}, {"name": "contest_name", "dtype": "string"}, {"name": "contest_type", "dtype": "string"}, {"name": "contest_start", "dtype": "int64"}, {"name": "contest_start_year", "dtype": "int64"}, {"name": "index", "dtype": "string"}, {"name": "time_limit", "dtype": "float64"}, {"name": "memory_limit", "dtype": "int64"}, {"name": "title", "dtype": "string"}, {"name": "description", "dtype": "string"}, {"name": "input_format", "dtype": "string"}, {"name": "output_format", "dtype": "string"}, {"name": "examples", "list": [{"name": "input", "dtype": "string"}, {"name": "output", "dtype": "string"}]}, {"name": "note", "dtype": "string"}, {"name": "editorial", "dtype": "string"}, {"name": "prompt", "dtype": "string"}, {"name": "generation", "dtype": "string"}, {"name": "finish_reason", "dtype": "string"}, {"name": "api_metadata", "struct": [{"name": "completion_tokens", "dtype": "int64"}, {"name": "prompt_tokens", "dtype": "int64"}, {"name": "prompt_tokens_details", "dtype": "null"}, {"name": "total_tokens", "dtype": "int64"}]}, {"name": "interaction_format", "dtype": "string"}, {"name": "messages", "list": [{"name": "content", "dtype": "string"}, {"name": "role", "dtype": "string"}]}], "splits": [{"name": "train", "num_bytes": 4968074271, "num_examples": 47780}], "download_size": 1887049179, "dataset_size": 4968074271}, {"config_name": "solutions_decontaminated", "features": [{"name": "id", "dtype": "string"}, {"name": "aliases", "sequence": "string"}, {"name": "contest_id", "dtype": "string"}, {"name": "contest_name", "dtype": "string"}, {"name": "contest_type", "dtype": "string"}, {"name": "contest_start", "dtype": "int64"}, {"name": "contest_start_year", "dtype": "int64"}, {"name": "index", "dtype": "string"}, {"name": "time_limit", "dtype": "float64"}, {"name": "memory_limit", "dtype": "float64"}, {"name": "title", "dtype": "string"}, {"name": "description", "dtype": "string"}, {"name": "input_format", "dtype": "string"}, {"name": "output_format", "dtype": "string"}, {"name": "examples", "list": [{"name": "input", "dtype": "string"}, {"name": "output", "dtype": "string"}]}, {"name": "note", "dtype": "string"}, {"name": "editorial", "dtype": "string"}, {"name": "problem", "dtype": "string"}, {"name": "generation", "dtype": "string"}, {"name": "finish_reason", "dtype": "string"}, {"name": "api_metadata", "struct": [{"name": "completion_tokens", "dtype": "int64"}, {"name": "prompt_tokens", "dtype": "int64"}, {"name": "prompt_tokens_details", "dtype": "null"}, {"name": "total_tokens", "dtype": "int64"}]}, {"name": "interaction_format", "dtype": "string"}, {"name": "messages", "list": [{"name": "content", "dtype": "string"}, {"name": "role", "dtype": "string"}]}, {"name": "problem_type", "dtype": "string"}, {"name": "public_tests", "struct": [{"name": "input", "sequence": "string"}, {"name": "output", "sequence": "string"}]}, {"name": "private_tests", "struct": [{"name": "input", "sequence": "string"}, {"name": "output", "sequence": "string"}]}, {"name": "generated_tests", "struct": [{"name": "input", "sequence": "string"}, {"name": "output", "sequence": "string"}]}, {"name": "public_tests_ms", "list": [{"name": "input", "dtype": "string"}, {"name": "output", "dtype": "string"}]}, {"name": "failed_solutions", "list": [{"name": "code", "dtype": "string"}, {"name": "passedTestCount", "dtype": "int64"}, {"name": "programmingLanguage", "dtype": "string"}, {"name": "verdict", "dtype": "string"}]}, {"name": "accepted_solutions", "list": [{"name": "code", "dtype": "string"}, {"name": "passedTestCount", "dtype": "int64"}, {"name": "passed_test_count", "dtype": "null"}, {"name": "programmingLanguage", "dtype": "string"}, {"name": "programming_language", "dtype": "string"}, {"name": "submission_id", "dtype": "string"}, {"name": "verdict", "dtype": "string"}]}], "splits": [{"name": "train", "num_bytes": 6719356671, "num_examples": 40665}], "download_size": 2023394671, "dataset_size": 6719356671}, {"config_name": "solutions_py", "features": [{"name": "id", "dtype": "string"}, {"name": "aliases", "sequence": "string"}, {"name": "contest_id", "dtype": "string"}, {"name": "contest_name", "dtype": "string"}, {"name": "contest_type", "dtype": "string"}, {"name": "contest_start", "dtype": "int64"}, {"name": "contest_start_year", "dtype": "int64"}, {"name": "index", "dtype": "string"}, {"name": "time_limit", "dtype": "float64"}, {"name": "memory_limit", "dtype": "float64"}, {"name": "title", "dtype": "string"}, {"name": "description", "dtype": "string"}, {"name": "input_format", "dtype": "string"}, {"name": "output_format", "dtype": "string"}, {"name": "interaction_format", "dtype": "string"}, {"name": "note", "dtype": "string"}, {"name": "examples", "list": [{"name": "input", "dtype": "string"}, {"name": "output", "dtype": "string"}]}, {"name": "editorial", "dtype": "string"}, {"name": "prompt", "dtype": "string"}, {"name": "generation", "dtype": "string"}, {"name": "finish_reason", "dtype": "string"}, {"name": "api_metadata", "struct": [{"name": "completion_tokens", "dtype": "int64"}, {"name": "prompt_tokens", "dtype": "int64"}, {"name": "prompt_tokens_details", "dtype": "null"}, {"name": "total_tokens", "dtype": "int64"}]}, {"name": "messages", "list": [{"name": "content", "dtype": "string"}, {"name": "role", "dtype": "string"}]}], "splits": [{"name": "train", "num_bytes": 1000253222, "num_examples": 9556}], "download_size": 411697337, "dataset_size": 1000253222}, {"config_name": "solutions_py_decontaminated", "features": [{"name": "id", "dtype": "string"}, {"name": "aliases", "sequence": "string"}, {"name": "contest_id", "dtype": "string"}, {"name": "contest_name", "dtype": "string"}, {"name": "contest_type", "dtype": "string"}, {"name": "contest_start", "dtype": "int64"}, {"name": "contest_start_year", "dtype": "int64"}, {"name": "index", "dtype": "string"}, {"name": "time_limit", "dtype": "float64"}, {"name": "memory_limit", "dtype": "float64"}, {"name": "title", "dtype": "string"}, {"name": "description", "dtype": "string"}, {"name": "input_format", "dtype": "string"}, {"name": "output_format", "dtype": "string"}, {"name": "interaction_format", "dtype": "string"}, {"name": "note", "dtype": "string"}, {"name": "examples", "list": [{"name": "input", "dtype": "string"}, {"name": "output", "dtype": "string"}]}, {"name": "editorial", "dtype": "string"}, {"name": "prompt", "dtype": "string"}, {"name": "generation", "dtype": "string"}, {"name": "finish_reason", "dtype": "string"}, {"name": "api_metadata", "struct": [{"name": "completion_tokens", "dtype": "int64"}, {"name": "prompt_tokens", "dtype": "int64"}, {"name": "prompt_tokens_details", "dtype": "null"}, {"name": "total_tokens", "dtype": "int64"}]}, {"name": "messages", "list": [{"name": "content", "dtype": "string"}, {"name": "role", "dtype": "string"}]}, {"name": "accepted_solutions", "list": [{"name": "code", "dtype": "string"}, {"name": "passedTestCount", "dtype": "int64"}, {"name": "passed_test_count", "dtype": "null"}, {"name": "programmingLanguage", "dtype": "string"}, {"name": "programming_language", "dtype": "string"}, {"name": "submission_id", "dtype": "string"}, {"name": "verdict", "dtype": "string"}]}, {"name": "failed_solutions", "list": [{"name": "code", "dtype": "string"}, {"name": "passedTestCount", "dtype": "int64"}, {"name": "programmingLanguage", "dtype": "string"}, {"name": "verdict", "dtype": "string"}]}, {"name": "generated_tests", "struct": [{"name": "input", "sequence": "string"}, {"name": "output", "sequence": "string"}]}, {"name": "private_tests", "struct": [{"name": "input", "sequence": "string"}, {"name": "output", "sequence": "string"}]}, {"name": "problem_type", "dtype": "string"}, {"name": "public_tests", "struct": [{"name": "input", "sequence": "string"}, {"name": "output", "sequence": "string"}]}, {"name": "public_tests_ms", "list": [{"name": "input", "dtype": "string"}, {"name": "output", "dtype": "string"}]}], "splits": [{"name": "train", "num_bytes": 1349328880, "num_examples": 8133}], "download_size": 500182086, "dataset_size": 1349328880}, {"config_name": "solutions_short_and_long_decontaminated", "features": [{"name": "id", "dtype": "string"}, {"name": "aliases", "sequence": "string"}, {"name": "contest_id", "dtype": "string"}, {"name": "contest_name", "dtype": "string"}, {"name": "contest_type", "dtype": "string"}, {"name": "contest_start", "dtype": "int64"}, {"name": "contest_start_year", "dtype": "int64"}, {"name": "index", "dtype": "string"}, {"name": "time_limit", "dtype": "float64"}, {"name": "memory_limit", "dtype": "float64"}, {"name": "title", "dtype": "string"}, {"name": "description", "dtype": "string"}, {"name": "input_format", "dtype": "string"}, {"name": "output_format", "dtype": "string"}, {"name": "examples", "list": [{"name": "input", "dtype": "string"}, {"name": "output", "dtype": "string"}]}, {"name": "note", "dtype": "string"}, {"name": "editorial", "dtype": "string"}, {"name": "prompt", "dtype": "string"}, {"name": "generation", "dtype": "string"}, {"name": "finish_reason", "dtype": "string"}, {"name": "api_metadata", "struct": [{"name": "completion_tokens", "dtype": "int64"}, {"name": "prompt_tokens", "dtype": "int64"}, {"name": "prompt_tokens_details", "dtype": "null"}, {"name": "total_tokens", "dtype": "int64"}]}, {"name": "interaction_format", "dtype": "string"}, {"name": "messages", "list": [{"name": "content", "dtype": "string"}, {"name": "role", "dtype": "string"}]}, {"name": "accepted_solutions", "list": [{"name": "code", "dtype": "string"}, {"name": "passedTestCount", "dtype": "int64"}, {"name": "passed_test_count", "dtype": "null"}, {"name": "programmingLanguage", "dtype": "string"}, {"name": "programming_language", "dtype": "string"}, {"name": "submission_id", "dtype": "string"}, {"name": "verdict", "dtype": "string"}]}, {"name": "failed_solutions", "list": [{"name": "code", "dtype": "string"}, {"name": "passedTestCount", "dtype": "int64"}, {"name": "programmingLanguage", "dtype": "string"}, {"name": "verdict", "dtype": "string"}]}, {"name": "generated_tests", "struct": [{"name": "input", "sequence": "string"}, {"name": "output", "sequence": "string"}]}, {"name": "private_tests", "struct": [{"name": "input", "sequence": "string"}, {"name": "output", "sequence": "string"}]}, {"name": "problem_type", "dtype": "string"}, {"name": "public_tests", "struct": [{"name": "input", "sequence": "string"}, {"name": "output", "sequence": "string"}]}, {"name": "public_tests_ms", "list": [{"name": "input", "dtype": "string"}, {"name": "output", "dtype": "string"}]}], "splits": [{"name": "train", "num_bytes": 2699204607, "num_examples": 16266}], "download_size": 1002365269, "dataset_size": 2699204607}, {"config_name": "solutions_w_editorials", "features": [{"name": "id", "dtype": "string"}, {"name": "aliases", "sequence": "string"}, {"name": "contest_id", "dtype": "string"}, {"name": "contest_name", "dtype": "string"}, {"name": "contest_type", "dtype": "string"}, {"name": "contest_start", "dtype": "int64"}, {"name": "contest_start_year", "dtype": "int64"}, {"name": "index", "dtype": "string"}, {"name": "time_limit", "dtype": "float64"}, {"name": "memory_limit", "dtype": "int64"}, {"name": "title", "dtype": "string"}, {"name": "description", "dtype": "string"}, {"name": "input_format", "dtype": "string"}, {"name": "output_format", "dtype": "string"}, {"name": "interaction_format", "dtype": "string"}, {"name": "note", "dtype": "string"}, {"name": "examples", "list": [{"name": "input", "dtype": "string"}, {"name": "output", "dtype": "string"}]}, {"name": "editorial", "dtype": "string"}, {"name": "prompt", "dtype": "string"}, {"name": "generation", "dtype": "string"}, {"name": "finish_reason", "dtype": "string"}, {"name": "api_metadata", "struct": [{"name": "completion_tokens", "dtype": "int64"}, {"name": "prompt_tokens", "dtype": "int64"}, {"name": "prompt_tokens_details", "dtype": "null"}, {"name": "total_tokens", "dtype": "int64"}]}, {"name": "messages", "list": [{"name": "content", "dtype": "string"}, {"name": "role", "dtype": "string"}]}], "splits": [{"name": "train", "num_bytes": 2649620432, "num_examples": 29180}], "download_size": 972089090, "dataset_size": 2649620432}, {"config_name": "solutions_w_editorials_decontaminated", "features": [{"name": "id", "dtype": "string"}, {"name": "aliases", "sequence": "string"}, {"name": "contest_id", "dtype": "string"}, {"name": "contest_name", "dtype": "string"}, {"name": "contest_type", "dtype": "string"}, {"name": "contest_start", "dtype": "int64"}, {"name": "contest_start_year", "dtype": "int64"}, {"name": "index", "dtype": "string"}, {"name": "time_limit", "dtype": "float64"}, {"name": "memory_limit", "dtype": "int64"}, {"name": "title", "dtype": "string"}, {"name": "description", "dtype": "string"}, {"name": "input_format", "dtype": "string"}, {"name": "output_format", "dtype": "string"}, {"name": "interaction_format", "dtype": "string"}, {"name": "note", "dtype": "string"}, {"name": "examples", "list": [{"name": "input", "dtype": "string"}, {"name": "output", "dtype": "string"}]}, {"name": "editorial", "dtype": "string"}, {"name": "prompt", "dtype": "string"}, {"name": "generation", "dtype": "string"}, {"name": "finish_reason", "dtype": "string"}, {"name": "api_metadata", "struct": [{"name": "completion_tokens", "dtype": "int64"}, {"name": "prompt_tokens", "dtype": "int64"}, {"name": "prompt_tokens_details", "dtype": "null"}, {"name": "total_tokens", "dtype": "int64"}]}, {"name": "messages", "list": [{"name": "content", "dtype": "string"}, {"name": "role", "dtype": "string"}]}, {"name": "accepted_solutions", "list": [{"name": "code", "dtype": "string"}, {"name": "passedTestCount", "dtype": "int64"}, {"name": "passed_test_count", "dtype": "null"}, {"name": "programmingLanguage", "dtype": "string"}, {"name": "programming_language", "dtype": "string"}, {"name": "submission_id", "dtype": "string"}, {"name": "verdict", "dtype": "string"}]}, {"name": "failed_solutions", "list": [{"name": "code", "dtype": "string"}, {"name": "passedTestCount", "dtype": "int64"}, {"name": "programmingLanguage", "dtype": "string"}, {"name": "verdict", "dtype": "string"}]}, {"name": "generated_tests", "struct": [{"name": "input", "sequence": "string"}, {"name": "output", "sequence": "string"}]}, {"name": "private_tests", "struct": [{"name": "input", "sequence": "string"}, {"name": "output", "sequence": "string"}]}, {"name": "problem_type", "dtype": "string"}, {"name": "public_tests", "struct": [{"name": "input", "sequence": "string"}, {"name": "output", "sequence": "string"}]}, {"name": "public_tests_ms", "list": [{"name": "input", "dtype": "string"}, {"name": "output", "dtype": "string"}]}], "splits": [{"name": "train", "num_bytes": 3738669884, "num_examples": 24490}], "download_size": 1012247387, "dataset_size": 3738669884}, {"config_name": "solutions_w_editorials_py", "features": [{"name": "id", "dtype": "string"}, {"name": "aliases", "sequence": "string"}, {"name": "contest_id", "dtype": "string"}, {"name": "contest_name", "dtype": "string"}, {"name": "contest_type", "dtype": "string"}, {"name": "contest_start", "dtype": "int64"}, {"name": "contest_start_year", "dtype": "int64"}, {"name": "index", "dtype": "string"}, {"name": "time_limit", "dtype": "float64"}, {"name": "memory_limit", "dtype": "float64"}, {"name": "title", "dtype": "string"}, {"name": "description", "dtype": "string"}, {"name": "input_format", "dtype": "string"}, {"name": "output_format", "dtype": "string"}, {"name": "interaction_format", "dtype": "string"}, {"name": "note", "dtype": "string"}, {"name": "examples", "list": [{"name": "input", "dtype": "string"}, {"name": "output", "dtype": "string"}]}, {"name": "editorial", "dtype": "string"}, {"name": "prompt", "dtype": "string"}, {"name": "generation", "dtype": "string"}, {"name": "finish_reason", "dtype": "string"}, {"name": "api_metadata", "struct": [{"name": "completion_tokens", "dtype": "int64"}, {"name": "prompt_tokens", "dtype": "int64"}, {"name": "prompt_tokens_details", "dtype": "null"}, {"name": "total_tokens", "dtype": "int64"}]}, {"name": "messages", "list": [{"name": "content", "dtype": "string"}, {"name": "role", "dtype": "string"}]}], "splits": [{"name": "train", "num_bytes": 1067124847, "num_examples": 11672}], "download_size": 415023817, "dataset_size": 1067124847}, {"config_name": "solutions_w_editorials_py_decontaminated", "features": [{"name": "id", "dtype": "string"}, {"name": "aliases", "sequence": "string"}, {"name": "contest_id", "dtype": "string"}, {"name": "contest_name", "dtype": "string"}, {"name": "contest_type", "dtype": "string"}, {"name": "contest_start", "dtype": "int64"}, {"name": "contest_start_year", "dtype": "int64"}, {"name": "index", "dtype": "string"}, {"name": "time_limit", "dtype": "float64"}, {"name": "memory_limit", "dtype": "float64"}, {"name": "title", "dtype": "string"}, {"name": "description", "dtype": "string"}, {"name": "input_format", "dtype": "string"}, {"name": "output_format", "dtype": "string"}, {"name": "interaction_format", "dtype": "string"}, {"name": "note", "dtype": "string"}, {"name": "examples", "list": [{"name": "input", "dtype": "string"}, {"name": "output", "dtype": "string"}]}, {"name": "editorial", "dtype": "string"}, {"name": "prompt", "dtype": "string"}, {"name": "generation", "dtype": "string"}, {"name": "finish_reason", "dtype": "string"}, {"name": "api_metadata", "struct": [{"name": "completion_tokens", "dtype": "int64"}, {"name": "prompt_tokens", "dtype": "int64"}, {"name": "prompt_tokens_details", "dtype": "null"}, {"name": "total_tokens", "dtype": "int64"}]}, {"name": "messages", "list": [{"name": "content", "dtype": "string"}, {"name": "role", "dtype": "string"}]}, {"name": "accepted_solutions", "list": [{"name": "code", "dtype": "string"}, {"name": "passedTestCount", "dtype": "int64"}, {"name": "passed_test_count", "dtype": "null"}, {"name": "programmingLanguage", "dtype": "string"}, {"name": "programming_language", "dtype": "string"}, {"name": "submission_id", "dtype": "string"}, {"name": "verdict", "dtype": "string"}]}, {"name": "failed_solutions", "list": [{"name": "code", "dtype": "string"}, {"name": "passedTestCount", "dtype": "int64"}, {"name": "programmingLanguage", "dtype": "string"}, {"name": "verdict", "dtype": "string"}]}, {"name": "generated_tests", "struct": [{"name": "input", "sequence": "string"}, {"name": "output", "sequence": "string"}]}, {"name": "private_tests", "struct": [{"name": "input", "sequence": "string"}, {"name": "output", "sequence": "string"}]}, {"name": "problem_type", "dtype": "string"}, {"name": "public_tests", "struct": [{"name": "input", "sequence": "string"}, {"name": "output", "sequence": "string"}]}, {"name": "public_tests_ms", "list": [{"name": "input", "dtype": "string"}, {"name": "output", "dtype": "string"}]}], "splits": [{"name": "train", "num_bytes": 1499075280, "num_examples": 9796}], "download_size": 466078291, "dataset_size": 1499075280}, {"config_name": "test_input_generator", "features": [{"name": "id", "dtype": "string"}, {"name": "aliases", "sequence": "string"}, {"name": "contest_id", "dtype": "string"}, {"name": "contest_name", "dtype": "string"}, {"name": "contest_type", "dtype": "string"}, {"name": "contest_start", "dtype": "int64"}, {"name": "contest_start_year", "dtype": "int64"}, {"name": "index", "dtype": "string"}, {"name": "time_limit", "dtype": "float64"}, {"name": "memory_limit", "dtype": "float64"}, {"name": "title", "dtype": "string"}, {"name": "description", "dtype": "string"}, {"name": "input_format", "dtype": "string"}, {"name": "output_format", "dtype": "string"}, {"name": "examples", "list": [{"name": "input", "dtype": "string"}, {"name": "output", "dtype": "string"}]}, {"name": "note", "dtype": "string"}, {"name": "editorial", "dtype": "string"}, {"name": "prompt", "dtype": "string"}, {"name": "generation", "dtype": "string"}, {"name": "finish_reason", "dtype": "string"}, {"name": "api_metadata", "struct": [{"name": "completion_tokens", "dtype": "int64"}, {"name": "completion_tokens_details", "dtype": "null"}, {"name": "prompt_tokens", "dtype": "int64"}, {"name": "prompt_tokens_details", "dtype": "null"}, {"name": "total_tokens", "dtype": "int64"}]}, {"name": "interaction_format", "dtype": "string"}, {"name": "messages", "list": [{"name": "content", "dtype": "string"}, {"name": "role", "dtype": "string"}]}], "splits": [{"name": "train", "num_bytes": 1851104290, "num_examples": 20620}], "download_size": 724157877, "dataset_size": 1851104290}], "configs": [{"config_name": "checker_interactor", "data_files": [{"split": "train", "path": "checker_interactor/train-*"}]}, {"config_name": "solutions", "default": true, "data_files": [{"split": "train", "path": "solutions/train-*"}]}, {"config_name": "solutions_decontaminated", "data_files": [{"split": "train", "path": "solutions_decontaminated/train-*"}]}, {"config_name": "solutions_py", "data_files": [{"split": "train", "path": "solutions_py/train-*"}]}, {"config_name": "solutions_py_decontaminated", "data_files": [{"split": "train", "path": "solutions_py_decontaminated/train-*"}]}, {"config_name": "solutions_short_and_long_decontaminated", "data_files": [{"split": "train", "path": "solutions_short_and_long_decontaminated/train-*"}]}, {"config_name": "solutions_w_editorials", "data_files": [{"split": "train", "path": "solutions_w_editorials/train-*"}]}, {"config_name": "solutions_w_editorials_decontaminated", "data_files": [{"split": "train", "path": "solutions_w_editorials_decontaminated/train-*"}]}, {"config_name": "solutions_w_editorials_py", "data_files": [{"split": "train", "path": "solutions_w_editorials_py/train-*"}]}, {"config_name": "solutions_w_editorials_py_decontaminated", "data_files": [{"split": "train", "path": "solutions_w_editorials_py_decontaminated/train-*"}]}, {"config_name": "test_input_generator", "data_files": [{"split": "train", "path": "test_input_generator/train-*"}]}], "license": "cc-by-4.0"} | false | null | 2025-03-28T12:21:06 | 150 | 9 | false | 39ac85c150806230473c70ad72c31f6232fe3f41 |
Dataset Card for CodeForces-CoTs
Dataset description
CodeForces-CoTs is a large-scale dataset for training reasoning models on competitive programming tasks. It consists of 10k CodeForces problems with up to five reasoning traces generated by DeepSeek R1. We did not filter the traces for correctness, but found that around 84% of the Python ones pass the public tests.
The dataset consists of several subsets:
solutions: we prompt R1 to solve the problem and produce code.… See the full description on the dataset page: https://huggingface.co/datasets/open-r1/codeforces-cots. | 10,300 | 16,079 | [
"license:cc-by-4.0",
"size_categories:100K<n<1M",
"format:parquet",
"modality:tabular",
"modality:text",
"library:datasets",
"library:dask",
"library:mlcroissant",
"library:polars",
"region:us"
] | 2025-02-27T10:35:02 | null | null |
67e104c5e5179149a17a9b58 | amazon-agi/SIFT-50M | amazon-agi | {"license": "cdla-sharing-1.0", "language": ["en", "de", "fr", "it", "es"], "size_categories": ["10M<n<100M"], "task_categories": ["audio-text-to-text", "audio-classification", "text-to-speech", "audio-to-audio"], "pretty_name": "SIFT-50M", "configs": [{"config_name": "closed_ended_acoustic_level", "data_files": [{"split": "train", "path": "train/closed_ended/acoustic_level/*/*.jsonl"}, {"split": "validation", "path": "dev/closed_ended/acoustic_level/*/*.jsonl"}, {"split": "EvalSIFT", "path": "EvalSIFT/closed_ended/acoustic_level/*/*.jsonl"}]}, {"config_name": "closed_ended_content_level", "data_files": [{"split": "train", "path": "train/closed_ended/content_level/*/*.jsonl"}, {"split": "validation", "path": "dev/closed_ended/content_level/*/*.jsonl"}, {"split": "EvalSIFT", "path": "EvalSIFT/closed_ended/content_level/*/*.jsonl"}]}, {"config_name": "closed_ended_word_align", "data_files": [{"split": "train", "path": "train/closed_ended/word_align/*/*.jsonl"}, {"split": "validation", "path": "dev/closed_ended/word_align/*/*.jsonl"}, {"split": "EvalSIFT", "path": "EvalSIFT/closed_ended/word_align/*/*.jsonl"}]}, {"config_name": "closed_ended_comparison", "data_files": [{"split": "train", "path": "train/closed_ended/comparison/*/*.jsonl"}, {"split": "validation", "path": "dev/closed_ended/comparison/*/*.jsonl"}, {"split": "EvalSIFT", "path": "EvalSIFT/closed_ended/comparison/*/*.jsonl"}]}, {"config_name": "open_ended", "data_files": [{"split": "train", "path": "train/open_ended/*/*.jsonl"}, {"split": "validation", "path": "dev/open_ended/*/*.jsonl"}, {"split": "EvalSIFT", "path": "EvalSIFT/open_ended/*/*.jsonl"}]}, {"config_name": "controllable_generation", "data_files": [{"split": "train", "path": "train/controllable_generation/*/*.jsonl"}, {"split": "validation", "path": "dev/controllable_generation/*/*.jsonl"}, {"split": "EvalSIFT", "path": "EvalSIFT/controllable_generation/*/*.jsonl"}]}], "tags": ["speech", "speech-llm", "spoken-language-understanding", "controllable-speech-synthesis", "instruction-finetuning"]} | false | null | 2025-04-23T05:08:59 | 11 | 9 | false | 1277f4010c28983c41e4e549071f01b22af4bc87 |
Dataset Card for SIFT-50M
SIFT-50M (Speech Instruction Fine-Tuning) is a 50-million-example dataset designed for instruction fine-tuning and pre-training of speech-text large language models (LLMs). It is built from publicly available speech corpora containing a total of 14K hours of speech and leverages LLMs and off-the-shelf expert models. The dataset spans five languages, covering diverse aspects of speech understanding and controllable speech generation instructions. SIFT-50M… See the full description on the dataset page: https://huggingface.co/datasets/amazon-agi/SIFT-50M. | 7,034 | 7,034 | [
"task_categories:audio-text-to-text",
"task_categories:audio-classification",
"task_categories:text-to-speech",
"task_categories:audio-to-audio",
"language:en",
"language:de",
"language:fr",
"language:it",
"language:es",
"license:cdla-sharing-1.0",
"size_categories:10M<n<100M",
"format:json",
"modality:text",
"library:datasets",
"library:dask",
"library:mlcroissant",
"arxiv:2504.09081",
"region:us",
"speech",
"speech-llm",
"spoken-language-understanding",
"controllable-speech-synthesis",
"instruction-finetuning"
] | 2025-03-24T07:07:49 | null | null |
67e72c15e33793d82aebf06a | facebook/PLM-Video-Auto | facebook | {"language": ["en"], "license": "llama3.2", "multilinguality": ["monolingual"], "size_categories": ["1K<n<10K", "10K<n<100K", "100K<n<1M", "1M<n<10M", "10M<n<100M"], "source_datasets": ["YT1B", "Ego4D"], "task_categories": ["video-text-to-text"], "dataset_info": [{"config_name": "ego4d_qa", "features": [{"name": "video_id", "dtype": "string"}, {"name": "start_time", "dtype": "float"}, {"name": "end_time", "dtype": "float"}, {"name": "conversations", "list": [{"name": "from", "dtype": "string"}, {"name": "value", "dtype": "string"}]}], "splits": [{"name": "train", "num_bytes": 347276083, "num_examples": 703935}]}, {"config_name": "ego4d_cap", "features": [{"name": "video_id", "dtype": "string"}, {"name": "start_time", "dtype": "float"}, {"name": "end_time", "dtype": "float"}, {"name": "conversations", "list": [{"name": "from", "dtype": "string"}, {"name": "value", "dtype": "string"}]}], "splits": [{"name": "train", "num_bytes": 258468535, "num_examples": 183029}]}, {"config_name": "yt1b_cap", "features": [{"name": "video_id", "dtype": "string"}, {"name": "scene_id", "dtype": "string"}, {"name": "start_time", "dtype": "float"}, {"name": "end_time", "dtype": "float"}, {"name": "conversations", "list": [{"name": "from", "dtype": "string"}, {"name": "value", "dtype": "string"}]}, {"name": "data_engine_long_caption", "dtype": "string"}, {"name": "data_engine_short_caption", "dtype": "string"}, {"name": "plm_video_caption", "dtype": "string"}], "splits": [{"name": "train", "num_bytes": 25707216503, "num_examples": 2139893}]}, {"config_name": "yt1b_mcqa", "features": [{"name": "conversations", "list": [{"name": "from", "dtype": "string"}, {"name": "value", "dtype": "string"}]}, {"name": "category", "dtype": "string"}, {"name": "video_id", "dtype": "string"}, {"name": "start_time", "dtype": "float"}, {"name": "end_time", "dtype": "float"}], "splits": [{"name": "train", "num_bytes": 1716101945, "num_examples": 3383670}]}], "download_size": 11571038, "dataset_size": 17341769, "configs": [{"config_name": "ego4d_qa", "data_files": [{"split": "train", "path": "ego4d_qa/train-00000-of-00001.parquet"}]}, {"config_name": "ego4d_cap", "data_files": [{"split": "train", "path": "ego4d_cap/train-00000-of-00001.parquet"}]}, {"config_name": "yt1b_mcqa", "data_files": [{"split": "train", "path": "yt1b_mcqa/train-00000-of-00001.parquet"}]}, {"config_name": "yt1b_cap", "data_files": [{"split": "train", "path": "yt1b_cap/train-00000-of-000*.parquet"}]}]} | false | null | 2025-04-21T17:31:11 | 9 | 9 | false | 85d7f79e0eaa5058b0e7c7a553c7b5ce7ab53678 |
Dataset Card for PLM-Video Auto
[📃 Tech Report]
[📂 Github]
Sythetic video captions and MCQs used in PLM, please refer to the paper, Section 3, for more details. The sythetic annotations covers: YT-1B, Ego4d with captions, YT-1B with MCQAs and Ego4d with QAs.
Dataset Structure
YT-1B Captions (yt1b_cap)
Data fields are :
video_id: a string feature, unique identifier for the YouTube videoid.
scene_id: a string feature, unique identifier for the scene_id.… See the full description on the dataset page: https://huggingface.co/datasets/facebook/PLM-Video-Auto. | 265 | 265 | [
"task_categories:video-text-to-text",
"multilinguality:monolingual",
"source_datasets:YT1B",
"source_datasets:Ego4D",
"language:en",
"license:llama3.2",
"size_categories:1M<n<10M",
"format:parquet",
"modality:tabular",
"modality:text",
"library:datasets",
"library:pandas",
"library:mlcroissant",
"library:polars",
"arxiv:2504.13180",
"region:us"
] | 2025-03-28T23:09:09 | null | null |
67edf568d1631250f17528af | open-thoughts/OpenThoughts2-1M | open-thoughts | {"dataset_info": {"features": [{"name": "conversations", "list": [{"name": "from", "dtype": "string"}, {"name": "value", "dtype": "string"}]}, {"name": "question", "dtype": "string"}, {"name": "source", "dtype": "string"}, {"name": "id", "dtype": "int64"}], "splits": [{"name": "train", "num_bytes": 18986223337, "num_examples": 1143205}], "download_size": 8328411205, "dataset_size": 18986223337}, "configs": [{"config_name": "default", "data_files": [{"split": "train", "path": "data/train-*"}]}], "tags": ["synthetic", "curator"], "license": "apache-2.0"} | false | null | 2025-04-07T21:40:23 | 121 | 9 | false | 40766050d883e0aa951fd3ddee33faf3ad83f26b |
OpenThoughts2-1M
Open synthetic reasoning dataset with 1M high-quality examples covering math, science, code, and puzzles!
OpenThoughts2-1M builds upon our previous OpenThoughts-114k dataset, augmenting it with existing datasets like OpenR1, as well as additional math and code reasoning data.
This dataset was used to train OpenThinker2-7B and OpenThinker2-32B.
Inspect the content with rich formatting and search & filter capabilities in Curator Viewer.
See our blog post… See the full description on the dataset page: https://huggingface.co/datasets/open-thoughts/OpenThoughts2-1M. | 14,719 | 14,719 | [
"license:apache-2.0",
"size_categories:1M<n<10M",
"format:parquet",
"modality:text",
"library:datasets",
"library:dask",
"library:mlcroissant",
"library:polars",
"region:us",
"synthetic",
"curator"
] | 2025-04-03T02:41:44 | null | null |
67ff96ff01428cf3b86f78c2 | Lod34/sentiment-analysis-test | Lod34 | {"dataset_info": {"features": [{"name": "text", "dtype": "string"}, {"name": "sentiment", "dtype": "string"}], "splits": [{"name": "train", "num_bytes": 28302.111747851002, "num_examples": 279}, {"name": "test", "num_bytes": 7100.888252148997, "num_examples": 70}], "download_size": 23157, "dataset_size": 35403}, "configs": [{"config_name": "default", "data_files": [{"split": "train", "path": "data/train-*"}, {"split": "test", "path": "data/test-*"}]}], "annotations_creators": ["expert-generated", "crowdsourced"], "language": ["it"], "language_creators": ["crowdsourced"], "license": ["mit"], "multilinguality": ["monolingual"], "pretty_name": "A sentiment analysis database created in a school environment.", "size_categories": ["n<1K"], "source_datasets": ["original"], "tags": ["school", "high-school"], "task_categories": ["text-classification"], "task_ids": ["sentiment-analysis"]} | false | null | 2025-04-16T12:51:48 | 9 | 9 | false | cb263caa70c03ee67d894ea585bd51db9c74aac0 |
Progetto scolastico per l'analisi dei sentimenti
Il dataset è stato creato con un questionario online in cui si chiedeva ad un pubblico di studenti, docenti, personale amministrativo, famiglie di rispondere ad alcune domande sul loro rapporto con la scuola.
Le annotazioni sono state effettuate correlando le risposte testuali ad indicatori di gradimento.
Il dataset è stato realizzato all'interno di un corso pomeridiano scolastico dedicato all'intelligenza artificiale.
Grazie a tutti… See the full description on the dataset page: https://huggingface.co/datasets/Lod34/sentiment-analysis-test. | 57 | 57 | [
"task_categories:text-classification",
"task_ids:sentiment-analysis",
"annotations_creators:expert-generated",
"annotations_creators:crowdsourced",
"language_creators:crowdsourced",
"multilinguality:monolingual",
"source_datasets:original",
"language:it",
"license:mit",
"size_categories:n<1K",
"format:parquet",
"modality:text",
"library:datasets",
"library:pandas",
"library:mlcroissant",
"library:polars",
"region:us",
"school",
"high-school"
] | 2025-04-16T11:39:43 | null | null |
67ff98b701428cf3b86fe77e | Smatteux/sentiment-analysis-test | Smatteux | {"dataset_info": {"features": [{"name": "text", "dtype": "string"}, {"name": "sentiment", "dtype": "string"}], "splits": [{"name": "train", "num_bytes": 28302.111747851002, "num_examples": 279}, {"name": "test", "num_bytes": 7100.888252148997, "num_examples": 70}], "download_size": 23427, "dataset_size": 35403}, "configs": [{"config_name": "default", "data_files": [{"split": "train", "path": "data/train-*"}, {"split": "test", "path": "data/test-*"}]}], "annotations_creators": ["expert-generated", "crowdsourced"], "language": ["it"], "language_creators": ["crowdsourced"], "license": ["mit"], "multilinguality": ["monolingual"], "pretty_name": "a sentiment analysis database created in a school envronment ", "size_categories": ["n<1K"], "source_datasets": ["original"], "tags": [], "task_categories": ["text-classification"], "task_ids": ["sentiment-analysis"]} | false | null | 2025-04-16T12:51:30 | 9 | 9 | false | f00483c98dfd16fbc1d1e9dcbcd84ba830639b4e |
progetto scolastico per l'analisi dei sentimenti
il dataset è stato creato con un questionario online in cu isi chiedeva ad un pubblico di studenti, docenti, personale amministrativo, famiglie di rispondere ad alcune domande sul loro rapporto con la scuola.
Le annotazioni sono state effettuate correlando le risposte testuali ad indicatori di gradimento.
Il dataset è stato stato realizzato all'interno di un corsp pomeridiano scolastico dedicato all'intelligenza artificiale.
Grazie a… See the full description on the dataset page: https://huggingface.co/datasets/Smatteux/sentiment-analysis-test. | 82 | 82 | [
"task_categories:text-classification",
"task_ids:sentiment-analysis",
"annotations_creators:expert-generated",
"annotations_creators:crowdsourced",
"language_creators:crowdsourced",
"multilinguality:monolingual",
"source_datasets:original",
"language:it",
"license:mit",
"size_categories:n<1K",
"format:parquet",
"modality:text",
"library:datasets",
"library:pandas",
"library:mlcroissant",
"library:polars",
"region:us"
] | 2025-04-16T11:47:03 | null | null |
67ff9b10e3e15a8be9b4971e | MarcPal08/sentiment-analysis-test | MarcPal08 | {"dataset_info": {"features": [{"name": "text", "dtype": "string"}, {"name": "sentiment", "dtype": "string"}], "splits": [{"name": "train", "num_bytes": 28302.111747851002, "num_examples": 279}, {"name": "test", "num_bytes": 7100.888252148997, "num_examples": 70}], "download_size": 23157, "dataset_size": 35403}, "configs": [{"config_name": "default", "data_files": [{"split": "train", "path": "data/train-*"}, {"split": "test", "path": "data/test-*"}]}], "annotations_creators": ["expert-generated", "crowdsourced"], "language": ["it"], "language_creators": ["crowdsourced"], "license": ["mit"], "multilinguality": ["monolingual"], "pretty_name": "A sentiment analisys database created in a school environment.", "size_categories": ["n<1K"], "source_datasets": ["original"], "tags": ["school", "high-school"], "task_categories": ["text-classification"], "task_ids": ["sentiment-analysis"]} | false | null | 2025-04-16T12:51:30 | 9 | 9 | false | f870060177dff27ea2e81215f68d3c3f019bd51e |
Progetto scolastico per l'analisi dei sentimenti
Il dataset è stato creato con un questionario online in cui si chiedeva ad un pubblico di studenti, docenti, personale amministrativo, famiglie di rispondere ad alcune domande sul loro rapporto con la scuola.
Le annotazioni sono state effettuate correlando le risposte testuali ad indicatori di gradimento.
Il dataset è stato realizzato all'interno di un corso pomeridiano scolastico dedicato all'intelligenza artificiale.
Grazie a tutti… See the full description on the dataset page: https://huggingface.co/datasets/MarcPal08/sentiment-analysis-test. | 55 | 55 | [
"task_categories:text-classification",
"task_ids:sentiment-analysis",
"annotations_creators:expert-generated",
"annotations_creators:crowdsourced",
"language_creators:crowdsourced",
"multilinguality:monolingual",
"source_datasets:original",
"language:it",
"license:mit",
"size_categories:n<1K",
"format:parquet",
"modality:text",
"library:datasets",
"library:pandas",
"library:mlcroissant",
"library:polars",
"region:us",
"school",
"high-school"
] | 2025-04-16T11:57:04 | null | null |
67ffa024a1c34809696a1765 | Giova-tech/sentiment-analysis-test | Giova-tech | {"dataset_info": {"features": [{"name": "text", "dtype": "string"}, {"name": "sentiment", "dtype": "string"}], "splits": [{"name": "train", "num_bytes": 28302.111747851002, "num_examples": 279}, {"name": "test", "num_bytes": 7100.888252148997, "num_examples": 70}], "download_size": 23157, "dataset_size": 35403}, "configs": [{"config_name": "default", "data_files": [{"split": "train", "path": "data/train-*"}, {"split": "test", "path": "data/test-*"}]}], "annotations_creators": ["expert-generated", "crowdsourced"], "language": ["it"], "language_creators": ["crowdsourced"], "license": ["mit"], "multilinguality": ["monolingual"], "pretty_name": "A sentiment analisis database created in a school environment\n", "size_categories": ["n<1K"], "source_datasets": ["original"], "tags": ["school", "high-school"], "task_categories": ["text-classification"], "task_ids": ["sentiment-analysis"]} | false | null | 2025-04-16T12:51:43 | 9 | 9 | false | ad783643608757dc726d2376d370b7d6bfe5039d |
progetto scolastico per l'analisi dei sentimenti
Il dataset è stato creato con un questionario online in cui si chiedeva ad un pubblico di studenti, docenti, personake amministrativo e famiglie di rispondere ad alcune domande
sul loro rapporto con la scuola.
Le annotazioni sono state effettuate correlando le risposte testuali an indicatori di gradimento.
Il dataset è stato realizzato all'interno di un corso pomeridiano scolastico dedicato all'intelliggenza artificiale.
Grazie a… See the full description on the dataset page: https://huggingface.co/datasets/Giova-tech/sentiment-analysis-test. | 62 | 62 | [
"task_categories:text-classification",
"task_ids:sentiment-analysis",
"annotations_creators:expert-generated",
"annotations_creators:crowdsourced",
"language_creators:crowdsourced",
"multilinguality:monolingual",
"source_datasets:original",
"language:it",
"license:mit",
"size_categories:n<1K",
"format:parquet",
"modality:text",
"library:datasets",
"library:pandas",
"library:mlcroissant",
"library:polars",
"region:us",
"school",
"high-school"
] | 2025-04-16T12:18:44 | null | null |
621ffdd236468d709f181f3d | qiaojin/PubMedQA | qiaojin | {"annotations_creators": ["expert-generated", "machine-generated"], "language_creators": ["expert-generated"], "language": ["en"], "license": ["mit"], "multilinguality": ["monolingual"], "size_categories": ["100K<n<1M", "10K<n<100K", "1K<n<10K"], "source_datasets": ["original"], "task_categories": ["question-answering"], "task_ids": ["multiple-choice-qa"], "paperswithcode_id": "pubmedqa", "pretty_name": "PubMedQA", "config_names": ["pqa_artificial", "pqa_labeled", "pqa_unlabeled"], "dataset_info": [{"config_name": "pqa_artificial", "features": [{"name": "pubid", "dtype": "int32"}, {"name": "question", "dtype": "string"}, {"name": "context", "sequence": [{"name": "contexts", "dtype": "string"}, {"name": "labels", "dtype": "string"}, {"name": "meshes", "dtype": "string"}]}, {"name": "long_answer", "dtype": "string"}, {"name": "final_decision", "dtype": "string"}], "splits": [{"name": "train", "num_bytes": 443501057, "num_examples": 211269}], "download_size": 233411194, "dataset_size": 443501057}, {"config_name": "pqa_labeled", "features": [{"name": "pubid", "dtype": "int32"}, {"name": "question", "dtype": "string"}, {"name": "context", "sequence": [{"name": "contexts", "dtype": "string"}, {"name": "labels", "dtype": "string"}, {"name": "meshes", "dtype": "string"}, {"name": "reasoning_required_pred", "dtype": "string"}, {"name": "reasoning_free_pred", "dtype": "string"}]}, {"name": "long_answer", "dtype": "string"}, {"name": "final_decision", "dtype": "string"}], "splits": [{"name": "train", "num_bytes": 2088898, "num_examples": 1000}], "download_size": 1075513, "dataset_size": 2088898}, {"config_name": "pqa_unlabeled", "features": [{"name": "pubid", "dtype": "int32"}, {"name": "question", "dtype": "string"}, {"name": "context", "sequence": [{"name": "contexts", "dtype": "string"}, {"name": "labels", "dtype": "string"}, {"name": "meshes", "dtype": "string"}]}, {"name": "long_answer", "dtype": "string"}], "splits": [{"name": "train", "num_bytes": 125922964, "num_examples": 61249}], "download_size": 66010017, "dataset_size": 125922964}], "configs": [{"config_name": "pqa_artificial", "data_files": [{"split": "train", "path": "pqa_artificial/train-*"}]}, {"config_name": "pqa_labeled", "data_files": [{"split": "train", "path": "pqa_labeled/train-*"}]}, {"config_name": "pqa_unlabeled", "data_files": [{"split": "train", "path": "pqa_unlabeled/train-*"}]}]} | false | null | 2024-03-06T01:50:16 | 213 | 8 | false | 9001f2853fb87cab8d220904e0de81ac6973b318 |
Dataset Card for [Dataset Name]
Dataset Summary
The task of PubMedQA is to answer research questions with yes/no/maybe (e.g.: Do preoperative statins reduce atrial fibrillation after coronary artery bypass grafting?) using the corresponding abstracts.
Supported Tasks and Leaderboards
The official leaderboard is available at: https://pubmedqa.github.io/.
500 questions in the pqa_labeled are used as the test set. They can be found at… See the full description on the dataset page: https://huggingface.co/datasets/qiaojin/PubMedQA. | 13,740 | 422,330 | [
"task_categories:question-answering",
"task_ids:multiple-choice-qa",
"annotations_creators:expert-generated",
"annotations_creators:machine-generated",
"language_creators:expert-generated",
"multilinguality:monolingual",
"source_datasets:original",
"language:en",
"license:mit",
"size_categories:100K<n<1M",
"format:parquet",
"modality:text",
"library:datasets",
"library:pandas",
"library:mlcroissant",
"library:polars",
"arxiv:1909.06146",
"region:us"
] | 2022-03-02T23:29:22 | pubmedqa | null |
66c84764a47b2d6c582bbb02 | amphion/Emilia-Dataset | amphion | {"license": "cc-by-4.0", "task_categories": ["text-to-speech", "automatic-speech-recognition"], "language": ["zh", "en", "ja", "fr", "de", "ko"], "pretty_name": "Emilia", "size_categories": ["10M<n<100M"], "extra_gated_prompt": "Terms of Access: The researcher has requested permission to use the Emilia dataset, the Emilia-Pipe preprocessing pipeline, and the Emilia-Yodas dataset. In exchange for such permission, the researcher hereby agrees to the following terms and conditions:\n1. The researcher shall use the Emilia dataset under the CC-BY-NC license and\n the Emilia-YODAS dataset under the CC-BY license.\n2. The authors make no representations or warranties regarding the datasets,\n including but not limited to warranties of non-infringement or fitness for\n a particular purpose.\n3. The researcher accepts full responsibility for their use of the datasets and\n shall defend and indemnify the authors of Emilia, Emilia-Pipe, and\n Emilia-Yodas, including their employees, trustees, officers, and agents,\n against any and all claims arising from the researcher's use of the datasets,\n including but not limited to the researcher's use of any copies of copyrighted\n content that they may create from the datasets.\n4. The researcher may provide research associates and colleagues with access\n to the datasets, provided that they first agree to be bound by these terms\n and conditions.\n5. The authors reserve the right to terminate the researcher's access to the\n datasets at any time.\n6. If the researcher is employed by a for-profit, commercial entity, the\n researcher's employer shall also be bound by these terms and conditions,\n and the researcher hereby represents that they are fully authorized to enter\n into this agreement on behalf of such employer.", "extra_gated_fields": {"Name": "text", "Email": "text", "Affiliation": "text", "Position": "text", "Your Supervisor/manager/director": "text", "I agree to the Terms of Access": "checkbox"}} | false | null | 2025-02-28T05:41:37 | 295 | 8 | false | d7f2f7340a6385696f3766c8049fa920a4707c07 |
Emilia: An Extensive, Multilingual, and Diverse Speech Dataset for Large-Scale Speech Generation
This is the official repository 👑 for the Emilia dataset and the source code for the Emilia-Pipe speech data preprocessing pipeline.
News 🔥
2025/02/26: The Emilia-Large dataset, featuring over 200,000 hours of data, is now available!!! Emilia-Large combines the original 101k-hour Emilia dataset (licensed under CC BY-NC 4.0) with the brand-new 114k-hour Emilia-YODAS… See the full description on the dataset page: https://huggingface.co/datasets/amphion/Emilia-Dataset. | 134,193 | 472,187 | [
"task_categories:text-to-speech",
"task_categories:automatic-speech-recognition",
"language:zh",
"language:en",
"language:ja",
"language:fr",
"language:de",
"language:ko",
"license:cc-by-4.0",
"size_categories:10M<n<100M",
"format:webdataset",
"modality:audio",
"modality:text",
"library:datasets",
"library:webdataset",
"library:mlcroissant",
"arxiv:2407.05361",
"arxiv:2501.15907",
"region:us"
] | 2024-08-23T08:25:08 | null | null |
67e72c49b012e2a5bab13802 | facebook/PLM-Image-Auto | facebook | {"language": ["en"], "license": "llama3.2", "multilinguality": ["monolingual"], "size_categories": ["1K<n<10K", "10K<n<100K", "100K<n<1M", "1M<n<10M", "10M<n<100M"], "source_datasets": ["ArvixQA", "Object365", "OpenImages", "SA1B", "Pdfacc", "UCSF"], "task_categories": ["image-text-to-text"], "dataset_info": [{"config_name": "sa1b", "features": [{"name": "image_id", "dtype": "string"}, {"name": "image", "dtype": "string"}, {"name": "conversations", "list": [{"name": "from", "dtype": "string"}, {"name": "value", "dtype": "string"}]}], "splits": [{"name": "train", "num_bytes": 12136862595, "num_examples": 9360168}]}, {"config_name": "ucsf", "features": [{"name": "uuid", "dtype": "string"}, {"name": "pdf", "dtype": "string"}, {"name": "image", "dtype": "string"}, {"name": "page_id", "dtype": "string"}, {"name": "caption", "dtype": "string"}, {"name": "conversations", "list": [{"name": "from", "dtype": "string"}, {"name": "value", "dtype": "string"}]}], "splits": [{"name": "train", "num_bytes": 16907410115, "num_examples": 5953490}]}, {"config_name": "pdfacc", "features": [{"name": "uuid", "dtype": "string"}, {"name": "image", "dtype": "string"}, {"name": "conversations", "list": [{"name": "from", "dtype": "string"}, {"name": "value", "dtype": "string"}]}, {"name": "page", "dtype": "int32"}], "splits": [{"name": "train", "num_bytes": 40007664956, "num_examples": 12024670}]}, {"config_name": "openimages", "features": [{"name": "image", "dtype": "string"}, {"name": "uuid", "dtype": "string"}, {"name": "llama3v_80b_cap", "dtype": "string"}, {"name": "conversations", "list": [{"name": "from", "dtype": "string"}, {"name": "value", "dtype": "string"}]}], "splits": [{"name": "train", "num_bytes": 3073295811, "num_examples": 1730070}]}, {"config_name": "obj365", "features": [{"name": "image", "dtype": "string"}, {"name": "id", "dtype": "int32"}, {"name": "llama3v_80b_cap", "dtype": "string"}, {"name": "conversations", "list": [{"name": "from", "dtype": "string"}, {"name": "value", "dtype": "string"}]}], "splits": [{"name": "train", "num_bytes": 6051865770, "num_examples": 3435852}]}, {"config_name": "arxivqa", "features": [{"name": "arxiv_id", "dtype": "string"}, {"name": "uuid", "dtype": "string"}, {"name": "conversations", "list": [{"name": "from", "dtype": "string"}, {"name": "value", "dtype": "string"}]}, {"name": "image", "dtype": "string"}, {"name": "page", "dtype": "int32"}, {"name": "figure_num", "dtype": "int32"}], "splits": [{"name": "train", "num_bytes": 4781898174, "num_examples": 1859680}]}], "configs": [{"config_name": "arxivqa", "data_files": [{"split": "train", "path": "arxivqa/train-00000-of-00001.parquet"}]}, {"config_name": "obj365", "data_files": [{"split": "train", "path": "obj365/train-00000-of-00001.parquet"}]}, {"config_name": "openimages", "data_files": [{"split": "train", "path": "openimages/train-00000-of-00001.parquet"}]}, {"config_name": "pdfacc", "data_files": [{"split": "train", "path": "pdfacc/train-000*.parquet"}]}, {"config_name": "sa1b", "data_files": [{"split": "train", "path": "sa1b/train-0000*.parquet"}]}, {"config_name": "ucsf", "data_files": [{"split": "train", "path": "ucsf/train-0000*.parquet"}]}]} | false | null | 2025-04-21T18:03:31 | 8 | 8 | false | 0d00690c035bf82c4f2afd9a33f5bfbc206c38a3 |
Dataset Card for PLM-Image Auto
[📃 Tech Report]
[📂 Github]
Sythetic image captions and QAs used in PLM, please refer to the paper, Section 3, for more details. The sythetic annotations covers: SA1B, Openimages, Obejct365, ArxivQA, UCSF, PDFAcc.
Dataset Structure
Image Captions (SA1B, Openimages, Obejct365)
Data fields are :
image_id: a string feature, unique identifier for the image.
image: a string feature, the actual image path in the correspoding data… See the full description on the dataset page: https://huggingface.co/datasets/facebook/PLM-Image-Auto. | 253 | 253 | [
"task_categories:image-text-to-text",
"multilinguality:monolingual",
"source_datasets:ArvixQA",
"source_datasets:Object365",
"source_datasets:OpenImages",
"source_datasets:SA1B",
"source_datasets:Pdfacc",
"source_datasets:UCSF",
"language:en",
"license:llama3.2",
"size_categories:10M<n<100M",
"format:parquet",
"modality:tabular",
"modality:text",
"library:datasets",
"library:dask",
"library:mlcroissant",
"library:polars",
"arxiv:2504.13180",
"region:us"
] | 2025-03-28T23:10:01 | null | null |
67f44a4ba05a3db252415b21 | facebook/PLM-VideoBench | facebook | {"annotations_creators": ["other"], "language_creators": ["other"], "language": ["en"], "license": ["cc-by-4.0"], "task_categories": ["multiple-choice", "visual-question-answering"], "pretty_name": "PLM-VideoBench", "dataset_info": [{"config_name": "fgqa", "features": [{"name": "uid", "dtype": "string"}, {"name": "qa_uid", "dtype": "string"}, {"name": "video", "dtype": "string"}, {"name": "question", "dtype": "string"}, {"name": "answer", "dtype": "string"}, {"name": "options", "struct": [{"name": "option_0", "dtype": "string"}, {"name": "option_1", "dtype": "string"}]}, {"name": "answer_index", "dtype": "int32"}, {"name": "metadata", "struct": [{"name": "source_video_id", "dtype": "string"}, {"name": "source_dataset", "dtype": "string"}, {"name": "source_start_time", "dtype": "float"}, {"name": "source_end_time", "dtype": "float"}, {"name": "question_type", "dtype": "string"}, {"name": "source_domain", "dtype": "string"}], "dtype": "int32"}], "splits": [{"name": "test", "num_bytes": 10000, "num_examples": 10976}]}, {"config_name": "sgqa", "features": [{"name": "uid", "dtype": "string"}, {"name": "video", "dtype": "string"}, {"name": "question", "dtype": "string"}, {"name": "answer", "dtype": "string"}, {"name": "domain", "dtype": "string"}], "splits": [{"name": "test", "num_bytes": 10000, "num_examples": 665}]}, {"config_name": "rcap", "features": [{"name": "uid", "dtype": "int32"}, {"name": "video", "dtype": "string"}, {"name": "masklet_id", "dtype": "int32"}, {"name": "total_frames", "dtype": "int32"}, {"name": "caption", "dtype": "string"}, {"name": "start_frame", "dtype": "int32"}, {"name": "end_frame", "dtype": "int32"}], "splits": [{"name": "test", "num_bytes": 10000, "num_examples": 10060}, {"name": "val", "num_bytes": 10000, "num_examples": 4624}]}, {"config_name": "rdcap", "features": [{"name": "uid", "dtype": "int32"}, {"name": "video", "dtype": "string"}, {"name": "masklet_id", "dtype": "int32"}, {"name": "total_frames", "dtype": "int32"}, {"name": "dense_captions", "list": [{"name": "start_frame", "dtype": "int32"}, {"name": "end_frame", "dtype": "int32"}, {"name": "caption", "dtype": "string"}]}], "splits": [{"name": "test", "num_bytes": 10000, "num_examples": 2620}, {"name": "val", "num_bytes": 10000, "num_examples": 2551}]}, {"config_name": "rtloc", "features": [{"name": "uid", "dtype": "int32"}, {"name": "video", "dtype": "string"}, {"name": "masklet_id", "dtype": "int32"}, {"name": "total_frames", "dtype": "int32"}, {"name": "caption", "dtype": "string"}, {"name": "start_frame", "dtype": "int32"}, {"name": "end_frame", "dtype": "int32"}], "splits": [{"name": "test", "num_bytes": 10000, "num_examples": 7910}, {"name": "val", "num_bytes": 10000, "num_examples": 4624}]}], "configs": [{"config_name": "fgqa", "data_files": [{"split": "test", "path": "fgqa/plm_fgqa_test.parquet"}]}, {"config_name": "sgqa", "data_files": [{"split": "test", "path": "sgqa/plm_sgqa_test.parquet"}]}, {"config_name": "rcap", "data_files": [{"split": "test", "path": "rcap/plm_rcap_test.parquet"}, {"split": "val", "path": "rcap/plm_rcap_val.parquet"}]}, {"config_name": "rdcap", "data_files": [{"split": "test", "path": "rdcap/plm_rdcap_test.parquet"}, {"split": "val", "path": "rdcap/plm_rdcap_val.parquet"}]}, {"config_name": "rtloc", "data_files": [{"split": "test", "path": "rtloc/plm_rtloc_test.parquet"}, {"split": "val", "path": "rtloc/plm_rtloc_val.parquet"}]}]} | false | null | 2025-04-23T16:35:28 | 8 | 8 | false | 34336b1d0908f08fff3c844dba7f6dbebcdb5193 |
Dataset Summary
PLM-VideoBench is a collection of human-annotated resources for evaluating Vision Language models, focused on detailed video understanding.
[📃 Tech Report]
[📂 Github]
Supported Tasks
PLM-VideoBench includes evaluation data for the following tasks:
FGQA
In this task, a model must answer a multiple-choice question (MCQ) that probes fine-grained activity understanding. Given a question and multiple options that differ in a… See the full description on the dataset page: https://huggingface.co/datasets/facebook/PLM-VideoBench. | 848 | 848 | [
"task_categories:multiple-choice",
"task_categories:visual-question-answering",
"annotations_creators:other",
"language_creators:other",
"language:en",
"license:cc-by-4.0",
"size_categories:10K<n<100K",
"format:parquet",
"modality:tabular",
"modality:text",
"modality:video",
"library:datasets",
"library:pandas",
"library:mlcroissant",
"library:polars",
"arxiv:2504.13180",
"region:us"
] | 2025-04-07T21:57:31 | null | null |
67fe66c27cc6eabecbf8891a | davanstrien/fine-reasoning-questions | davanstrien | {"language": "en", "license": "mit", "tags": ["curator", "synthetic", "reasoning-datasets-competition"], "configs": [{"config_name": "default", "data_files": [{"split": "train", "path": "data/train-*"}]}, {"config_name": "raw", "data_files": [{"split": "train", "path": "raw/train-*"}]}], "dataset_info": [{"config_name": "default", "features": [{"name": "question", "dtype": "string"}, {"name": "requires_text_content", "dtype": "bool"}, {"name": "text", "dtype": "string"}, {"name": "id", "dtype": "string"}, {"name": "topic", "dtype": "string"}], "splits": [{"name": "train", "num_bytes": 1583427, "num_examples": 144}], "download_size": 459798, "dataset_size": 1583427}, {"config_name": "raw", "features": [{"name": "text", "dtype": "string"}, {"name": "id", "dtype": "string"}, {"name": "dump", "dtype": "string"}, {"name": "url", "dtype": "string"}, {"name": "file_path", "dtype": "string"}, {"name": "language", "dtype": "string"}, {"name": "language_score", "dtype": "float64"}, {"name": "token_count", "dtype": "int64"}, {"name": "score", "dtype": "float64"}, {"name": "int_score", "dtype": "int64"}, {"name": "raw_reasoning_score", "dtype": "float64"}, {"name": "reasoning_level", "dtype": "int64"}, {"name": "interpretation", "dtype": "string"}, {"name": "topic", "dtype": "string"}, {"name": "parsed_json", "dtype": "bool"}, {"name": "extracted_json", "struct": [{"name": "questions", "list": [{"name": "question", "dtype": "string"}, {"name": "requires_text_content", "dtype": "bool"}]}]}, {"name": "reasoning", "dtype": "string"}, {"name": "full_response", "dtype": "string"}], "splits": [{"name": "train", "num_bytes": 1907264, "num_examples": 100}], "download_size": 978916, "dataset_size": 1907264}]} | false | null | 2025-04-15T14:52:05 | 16 | 8 | false | 7430c6f200bfe605eb6af26c4c4ea4241ef1ae47 |
Dataset Card for Fine Reasoning Questions
Dataset Description
Can we generate reasoning datasets for more domains using web text?
Note: This dataset is submitted partly to give an idea of the kind of dataset you could submit to the reasoning datasets competition. You can find out more about the competition in this blog post.
You can also see more info on using Inference Providers with Curator here
The majority of reasoning datasets on the Hub are focused on maths… See the full description on the dataset page: https://huggingface.co/datasets/davanstrien/fine-reasoning-questions. | 313 | 313 | [
"language:en",
"license:mit",
"size_categories:n<1K",
"format:parquet",
"modality:tabular",
"modality:text",
"library:datasets",
"library:pandas",
"library:mlcroissant",
"library:polars",
"region:us",
"curator",
"synthetic",
"reasoning-datasets-competition"
] | 2025-04-15T14:01:38 | null | null |
67ff98ffb9227fa71db92d68 | qwertychri/sentiment-analysis-test | qwertychri | {"dataset_info": {"features": [{"name": "text", "dtype": "string"}, {"name": "sentiment", "dtype": "string"}], "splits": [{"name": "train", "num_bytes": 28302.111747851002, "num_examples": 279}, {"name": "test", "num_bytes": 7100.888252148997, "num_examples": 70}], "download_size": 23157, "dataset_size": 35403}, "configs": [{"config_name": "default", "data_files": [{"split": "train", "path": "data/train-*"}, {"split": "test", "path": "data/test-*"}]}], "annotations_creators": ["crowdsourced", "expert-generated"], "language": ["it"], "language_creators": ["crowdsourced"], "license": ["mit"], "multilinguality": ["monolingual"], "pretty_name": "A sentiment analysis database created in a school environment.", "size_categories": ["n<1K"], "source_datasets": ["original"], "tags": ["school", "high-school"], "task_categories": ["text-classification"], "task_ids": ["sentiment-analysis"]} | false | null | 2025-04-16T12:55:01 | 8 | 8 | false | 041f59a0be5ee98ed24a3e0a0b5b23b7b2a52e9f | Il dataset è stato creato in un questionario online in cui si chiedeva ad un pubblico di studenti, docenti, personale amministrativo, famiglie di rispondere ad alcune domande sul loro rapporto con la scuola.
Le annotazioni sono state effettuate correlando le risposte testuali ad indicatori di gradimento.
il dataset è stato realizzato all'interno di un corso pomeridiano scolastico dedicato all'intelligenza artificiale
Grazie a tutti per la collaborazione ❤️
| 43 | 43 | [
"task_categories:text-classification",
"task_ids:sentiment-analysis",
"annotations_creators:crowdsourced",
"annotations_creators:expert-generated",
"language_creators:crowdsourced",
"multilinguality:monolingual",
"source_datasets:original",
"language:it",
"license:mit",
"size_categories:n<1K",
"format:parquet",
"modality:text",
"library:datasets",
"library:pandas",
"library:mlcroissant",
"library:polars",
"region:us",
"school",
"high-school"
] | 2025-04-16T11:48:15 | null | null |
End of preview. Expand
in Data Studio
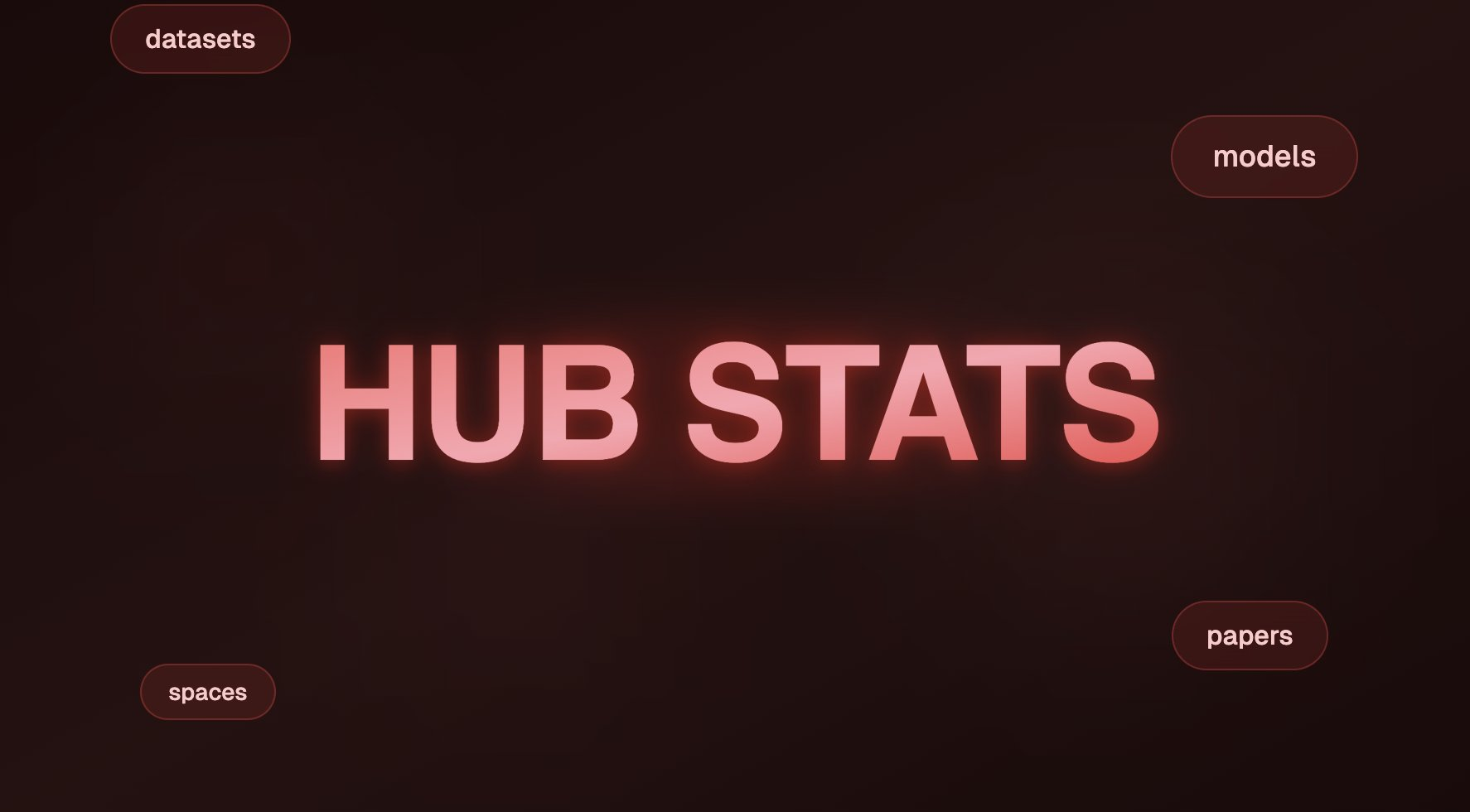
NEW Changes Feb 27th
Added new fields on the
models
split:downloadsAllTime
,safetensors
,gguf
Added new field on the
datasets
split:downloadsAllTime
Added new split:
papers
which is all of the Daily Papers
Updated Daily
- Downloads last month
- 6,542