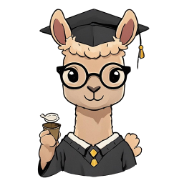
mmSSR-Styler Model Card
Paper | Project | GitHub | HF Collection
Cream of the Crop: Harvesting Rich, Scalable and Transferable Multi-Modal Data for Instruction Fine-Tuning
Mengyao Lyu,
Liyan, Huasong Zhong, Wenhao Yang, Hui Chen, Jungong Han, Guiguang Dingβ , Zhenheng Yang
Tsinghua University, BNRist, Bytedance
π The rapid yet inefficient expansion of multi-modal data, combined with the sheer token volume and increased heterogeneity of sources, amplifies both the significance and complexity of multi-modal data selection at scale.
π We redefine the granularity of data valuation by decomposing quality into 14 VL capabilities and formulating diversity into superficial interaction styles, such that multi-modal rich scorers and styler (mmSSR) guarantee that high-scoring information is conveyed to users in diversified forms.
π mmSSR is the first to scale to the 2.6M open data pool of LLaVA-OVSI, achieving 99.1% of full performance with only 30% of the data.
Across 10+ experimental settings, validated by 14+ multi-modal benchmarks, we demonstrate consistent improvements with varying budget constraints, general or specific capability customization and acquisition, and training-free generalization to new domains for curation.
π Performance
MMBenchen-v1.1 | MMStar | MMMU | MMVet | BLINK | MMT-Bench | MME | AI2D | ScienceQA | MathVistaMINI | >Rand | /FULL | |
---|---|---|---|---|---|---|---|---|---|---|---|---|
5% | ||||||||||||
Random | 73.74 | 47.98 | 43.70 | 42.34 | 50.61 | 58.87 | 2004.50 | 73.07 | 81.52 | 45.47 | - | 89.29 |
PPL-mid | 67.34 | 45.27 | 38.98 | 30.18 | 45.27 | 54.33 | 1887.71 | 66.74 | 74.76 | 31.40 | 0/10 | 78.31 |
PPL-si | 71.98 | 44.67 | 38.48 | 35.14 | 54.10 | 57.98 | 1856.79 | 67.84 | 78.24 | 36.50 | 1/10 | 83.10 |
Deita | 72.91 | 47.47 | 41.28 | 40.23 | 52.59 | 56.57 | 1956.50 | 70.76 | 79.57 | 36.10 | 1/10 | 85.79 |
CLIP | 74.23 | 47.27 | 40.08 | 35.73 | 52.96 | 56.73 | 1902.65 | 73.61 | 78.63 | 39.80 | 3/10 | 85.41 |
E5-V | 70.90 | 43.00 | 38.78 | 38.44 | 49.94 | 54.65 | 1810.47 | 66.58 | 77.54 | 37.40 | 0/10 | 81.87 |
COINCIDE | 72.76 | 48.33 | 43.17 | 45.60 | 49.43 | 57.50 | 1852.66 | 73.15 | 79.62 | 45.40 | 3/10 | 88.47 |
mmSSR | 77.79 | 53.33 | 43.27 | 43.53 | 51.83 | 59.16 | 1938.68 | 77.66 | 88.45 | 52.00 | 8/10 | 93.20 |
10% | ||||||||||||
Random | 74.57 | 51.57 | 44.72 | 42.91 | 52.59 | 58.99 | 2033.28 | 74.42 | 84.33 | 47.80 | 0/10 | 91.70 |
PPL-mid | 63.54 | 46.87 | 39.08 | 36.93 | 45.90 | 54.30 | 1831.03 | 67.23 | 73.87 | 39.50 | 0/10 | 80.72 |
PPL-si | 74.69 | 49.80 | 41.28 | 40.60 | 53.09 | 57.95 | 1841.11 | 75.16 | 80.71 | 40.40 | 3/10 | 87.63 |
Deita | 75.39 | 48.80 | 43.77 | 42.25 | 54.48 | 57.40 | 1996.34 | 71.60 | 78.33 | 40.80 | 2/10 | 88.72 |
CLIP | 75.23 | 49.87 | 40.38 | 37.16 | 53.59 | 59.35 | 1921.04 | 76.62 | 80.07 | 41.00 | 4/10 | 87.69 |
E5-V | 70.51 | 45.13 | 38.78 | 39.59 | 50.57 | 55.10 | 1787.94 | 68.94 | 77.54 | 37.20 | 0/10 | 82.76 |
COINCIDE | 75.23 | 49.73 | 44.77 | 42.52 | 50.69 | 58.71 | 2027.58 | 74.77 | 82.05 | 47.00 | 3/10 | 90.66 |
mmSSR | 77.32 | 53.27 | 45.06 | 42.98 | 54.10 | 59.61 | 2045.00 | 78.76 | 89.94 | 52.40 | 10/10 | 94.75 |
30% | ||||||||||||
Random | 78.25 | 54.60 | 44.40 | 46.10 | 55.23 | 59.61 | 2092.60 | 78.28 | 88.32 | 52.57 | - | 95.82 |
PPL-mid | 73.99 | 54.93 | 43.97 | 41.01 | 53.09 | 58.78 | 2036.54 | 77.20 | 87.01 | 56.40 | 2/10 | 93.77 |
PPL-si | 72.52 | 48.33 | 42.57 | 43.62 | 51.83 | 55.07 | 1976.46 | 76.55 | 78.48 | 42.20 | 0/10 | 88.22 |
Deita | 76.93 | 54.13 | 43.67 | 44.04 | 55.11 | 59.66 | 2042.63 | 79.50 | 83.54 | 50.30 | 2/10 | 94.05 |
CLIP | 74.30 | 53.80 | 43.07 | 45.87 | 51.95 | 59.16 | 2039.14 | 80.02 | 83.99 | 48.80 | 1/10 | 93.07 |
E5-V | 74.30 | 46.07 | 43.27 | 47.80 | 50.32 | 57.85 | 1955.13 | 74.45 | 81.61 | 43.70 | 1/10 | 89.52 |
COINCIDE | 78.02 | 55.47 | 45.66 | 46.24 | 52.84 | 59.80 | 2047.37 | 79.73 | 84.33 | 55.10 | 6/10 | 95.82 |
mmSSR | 79.57 | 57.53 | 44.87 | 48.49 | 56.24 | 59.83 | 2132.93 | 81.25 | 92.46 | 57.40 | 10/10 | 99.11 |
FULL | ||||||||||||
LLaVAOVSI | 80.57 | 59.40 | 45.16 | 47.16 | 56.87 | 60.73 | 2117.56 | 81.87 | 92.76 | 59.60 | - | 100 |
π₯ Example Usage
human: You are an AI expert annotator responsible for classifying the interaction styles of image-question-answer pairs. Identify the applicable styles from the candidate list, then rank the selected styles by frequency of occurrence.
Question: <image>
According to the question shown in the image, please first conduct reasoning, and then answer the question and provide the final value, e.g., The answer is xxx
Question: What is the area of the parallelogram? Answer: This parallelogram has base $b=4$ millimeters and height $h=3$ millimeters.
Multiply the base by the height to find the area in square millimeters.
\$\$
\\begin{aligned}
A & =b h \\\\
& =(4)(3) \\\\
& =12
\\end{aligned}
$$
The area of the parallelogram is $\\mathbf{1 2}$ square millimeters. So the answer is 12
The answer is 12
Interaction style candidates: [multi-choice, coordinate, yes/no, word/short-phrase, short description, detailed description, comparison, chain-of-thought (step-by-step), specified style]
Styles:
gpt: chain-of-thought (step-by-step), detailed description
The obtained styles will be used for subset sampling. Check out the codebase at lyumengyao/mmssr for detailed instructions.
π Citation
If you find mmSSR useful for your research or applications, please cite our paper:
@article{lyu2025cream,
title={Cream of the Crop: Harvesting Rich, Scalable and Transferable Multi-Modal Data for Instruction Fine-Tuning},
author={Lyu, Mengyao and Li, Yan and Zhong, Huasong and Yang, Wenhao and Chen, Hui and Han, Jungong and Ding, Guiguang and Yang, Zhenheng},
journal={arXiv preprint arXiv:2503.13383},
year={2025}
}