Post
700
As
xet-team
infrastructure begins backing hundreds of repositories on the Hugging Face Hub, we’re getting to put on our researcher hats and peer into the bytes. 👀 🤓
IMO, one of the most interesting ideas Xet storage introduces is a globally shared store of data.
When you upload a file through Xet, the contents are split into ~64KB chunks and deduplicated, but what if those same chunks already exist in another repo on the Hub?
If we can detect and reuse them, we skip them as well saving time and bandwidth for AI builders. More on how that works here:
🔗 https://huggingface.co/blog/from-chunks-to-blocks#scaling-deduplication-with-aggregation
Because of this, different repositories can share bytes we store. That opens up something cool - we can draw a graph of which repos actually share data at the chunk level, where:
- Nodes = repositories
- Edges = shared chunks
- Edge thickness = how much they overlap
xet-team/repo-graph
Come find the many BERT islands. Or see how datasets relate in practice, not just in theory. See how libraries or tasks can tie repositories together. You can play around with node size using storage/likes/downloads too.
The result is a super fun visualization from @saba9 and @znation that I’ve already lost way too much time to. I'm excited to see how the networks grow as we add more repositories!
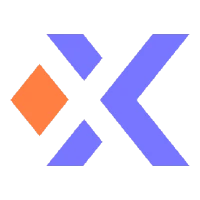
IMO, one of the most interesting ideas Xet storage introduces is a globally shared store of data.
When you upload a file through Xet, the contents are split into ~64KB chunks and deduplicated, but what if those same chunks already exist in another repo on the Hub?
If we can detect and reuse them, we skip them as well saving time and bandwidth for AI builders. More on how that works here:
🔗 https://huggingface.co/blog/from-chunks-to-blocks#scaling-deduplication-with-aggregation
Because of this, different repositories can share bytes we store. That opens up something cool - we can draw a graph of which repos actually share data at the chunk level, where:
- Nodes = repositories
- Edges = shared chunks
- Edge thickness = how much they overlap
xet-team/repo-graph
Come find the many BERT islands. Or see how datasets relate in practice, not just in theory. See how libraries or tasks can tie repositories together. You can play around with node size using storage/likes/downloads too.
The result is a super fun visualization from @saba9 and @znation that I’ve already lost way too much time to. I'm excited to see how the networks grow as we add more repositories!