Post
1548
If you've followed the progress of robotics in the past 18 months, you've likely noticed how robotics is increasingly becoming the next frontier that AI will unlock.
At Hugging Faceβin robotics and across all AI fieldsβwe believe in a future where AI and robots are open-source, transparent, and affordable; community-built and safe; hackable and fun. We've had so much mutual understanding and passion working with the Pollen Robotics team over the past year that we decided to join forces!
You can already find our open-source humanoid robot platform Reachy 2 on the Pollen website and the Pollen community and people here on the hub at
pollen-robotics
We're so excited to build and share more open-source robots with the world in the coming months!
At Hugging Faceβin robotics and across all AI fieldsβwe believe in a future where AI and robots are open-source, transparent, and affordable; community-built and safe; hackable and fun. We've had so much mutual understanding and passion working with the Pollen Robotics team over the past year that we decided to join forces!
You can already find our open-source humanoid robot platform Reachy 2 on the Pollen website and the Pollen community and people here on the hub at
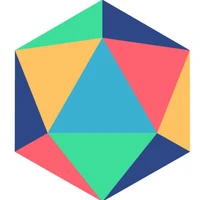
We're so excited to build and share more open-source robots with the world in the coming months!