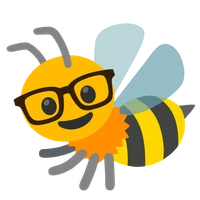
hf-internal-testing/single-file-large-model
Text Generation
โข
Updated
None defined yet.
transformers
๐generate
interface ๐คtransformers
with minimal effort. You'll also have access to all Hub features: a landing page for your creation, discussions, usage metrics, ... ๐ค